Archive for October, 2023
[ARTICLE] Rehabotics: A Comprehensive Rehabilitation Platform for Post-Stroke Spasticity, Incorporating a Soft Glove, a Robotic Exoskeleton Hand and Augmented Reality Serious Games – Full Text
Posted by Kostas Pantremenos in Paretic Hand, Rehabilitation robotics, Spasticity, Video Games/Exergames on October 31, 2023
Abstract
Spasticity following a stroke often leads to severe motor impairments, necessitating comprehensive and personalized rehabilitation protocols. This paper presents Rehabotics, an innovative rehabilitation platform incorporating a multi-component design for the rehabilitation of patients with post-stroke spasticity in the upper limbs. This system incorporates a sensor-equipped soft glove, a robotic exoskeleton hand, and an augmented reality (AR) platform with serious games of varying difficulties for adaptive therapy personalization. The soft glove collects data regarding hand movements and force exertion levels when the patient touches an object. In conjunction with a web camera, this enables real-time physical therapy using AR serious games, thus targeting specific motor skills. The exoskeleton hand, facilitated by servomotors, assists patients in hand movements, specifically aiding in overcoming the challenge of hand opening. The proposed system utilizes the data collected and (in combination with the clinical measurements) provides personalized and refined rehabilitation plans and targeted therapy to the affected hand. A pilot study of Rehabotics was conducted with a sample of 14 stroke patients. This novel system promises to enhance patient engagement and outcomes in post-stroke spasticity rehabilitation by providing a personalized, adaptive, and engaging therapy experience.
1. Introduction
Stroke is one of the leading causes of long-term disability globally, frequently resulting in upper limb spasticity. Spasticity is characterized by increased muscle tone, stiffness, exaggerated tendon reflexes, involuntary activation of the muscles and resistance to passive movement [1], which significantly affects patients’ quality of life [2]. Rehabilitation, including physical therapy and occupational therapy, is a key part of recovery after a stroke. However, the amount of time that post-stroke patients typically spend in the hospital working on their upper limb rehabilitation is inadequate for achieving a full recovery of function [3,4].
Traditional physiotherapy, while crucial for recovery, often encounters challenges such as suboptimal patient engagement and lack of personalization. Serious games have demonstrated a significant enhancement in patient motivation [5]. Also, innovative technologies, such as robotic exoskeletons and augmented reality (AR), have shown promising results in rehabilitating patients with motor impairments [6]. This maximizes the results when paired with the presence of a therapist (as in traditional physiotherapy), allowing for immediate adjustment and personalization of therapy based on the patient’s performance and feedback. Nevertheless, in telerehabilitation, while immediate therapist feedback might not be possible, the use of advanced technologies like AR can enable real-time adaptation and personalization of therapy protocols. The concept of serious games in rehabilitation is not new. In 2009, Burke et al. determined the principles of game design suitable for upper limb stroke rehabilitation, and several games were developed and showcased based on these principles [5]. Based on this premise, the challenge of customizing games for rehabilitation purposes, as stated in [7], lies in rehabilitation complexity, as it often demands human involvement. For instance, research on game usability has been conducted where assessment involved interviewing both rehabilitators and their patients [4]. In a proposal by Rabin et al. [8], the impact of serious games on motor control was studied using a serious game that progressively increases in difficulty for upper-limb rehabilitation. The primary issue with this approach is the adjustment of game parameters. Hocine et al. [7] detailed a universal technique for adjusting the difficulty of games intended for upper-limb rehabilitation, focusing on modifying pointing tasks and creating game levels. González-González et al. [9] proposed a recommender system which is able to provide the user with personalized games according to their history and skills. Also, devices such as KINECT have been used to adjust the difficulty levels using a fuzzy system [10].
To enhance motor functions, exoskeleton hand rehabilitation robots have been frequently applied, mimicking human limbs in their design, while being attached to the patient at various locations and having joint axes that align with those of the human joints. Moreover, they can be anchored to a table or be either mobile or fixed relative to the patient’s body [11]. Long-term therapy also includes a significant financial burden of the patients involved, as well as the necessity for patients to physically attend therapy sessions. This contributes to diminished enthusiasm, potentially leading to a decline in quality of life.
Taking the above into account, these challenges highlight the need for innovative systems that can support both therapists and patients during the rehabilitation process. Robotic systems, for instance, can alleviate the physical strain experienced by therapists while facilitating more intensive and extended training periods for patients. This can expedite recovery and enable the more effective execution of repetitive action exercises [6,12]. In this paper, we propose an innovative platform, “Rehabotics”, that addresses these limitations by combining a soft glove for data collection, a robotic exoskeleton hand, and AR serious games, thereby offering a comprehensive, patient-centric, and engaging approach to post-stroke spasticity rehabilitation.
The paper is organized as follows: Section 2 describes the components and methodology involved in the proposed system. In Section 3, we present the results from our pilot study. Finally, Section 4 includes a discussion section, reflecting on the study’s results, highlighting the potential of the Rehabotics system to enhance rehabilitation outcomes while suggesting areas for future research and addressing the limitation of the study. […]

[ARTICLE ] Brain–computer interface treatment for gait rehabilitation in stroke patients – Full Text
Posted by Kostas Pantremenos in Gait Rehabilitation - Foot Drop on October 29, 2023
The use of Brain–Computer Interfaces (BCI) as rehabilitation tools for chronically ill neurological patients has become more widespread. BCIs combined with other techniques allow the user to restore neurological function by inducing neuroplasticity through real-time detection of motor-imagery (MI) as patients perform therapy tasks. Twenty-five stroke patients with gait disability were recruited for this study. Participants performed 25 sessions with the MI-BCI and assessment visits to track functional changes during the therapy. The results of this study demonstrated a clinically significant increase in walking speed of 0.19 m/s, 95%CI [0.13–0.25], p < 0.001. Patients also reduced spasticity and improved their range of motion and muscle contraction. The BCI treatment was effective in promoting long-lasting functional improvements in the gait speed of chronic stroke survivors. Patients have more movements in the lower limb; therefore, they can walk better and safer. This functional improvement can be explained by improved neuroplasticity in the central nervous system.
1. Introduction
Stroke is one of the main causes of mortality and long-term disability worldwide. Functional deficit of the lower limb is the most common paresis after a stroke. Stroke patients rarely fully recover after months or even years of therapy and other treatment, leaving them with permanent impairment. Many of these patients never regain the ability to walk well enough to perform all their daily activities (Hesse et al., 2008; Mehrholz et al., 2017). Gait recovery is one of the major therapy goals in rehabilitation programs for stroke patients and many methods for gait analysis and rehabilitation have been developed (Mehrholz et al., 2017). Weakened muscle tone is another common challenge in motor rehabilitation. Therapies such as active foot drop exercises, electromechanically assisted therapy and treadmill therapy are usually limited to patients with mild or moderate impairment (Mills et al., 2011; Mehrholz et al., 2017).
A 2018 study (Mehrholz et al., 2018) conducted a network meta-analysis based on 95 publications out of 44.567 that were considered. In this study, 4.458 patients were included, and the effectiveness of the most common interventions for gait rehabilitation after stroke was analyzed. The interventions where classified in five groups: (1) No walking training, (2) Conventional walking training (walking on the floor, preparatory exercises in a sitting position, balance training etc. without technical aids and without treadmill training or electromechanical-assisted training), (3) Treadmill training without or with body-weight support, (4) Treadmill training with or without a walking speed paradigm, (5) Electromechanical-assisted training with end-effector devices or exoskeletons. For the primary endpoint of walking speed, end-effector-assisted training (EGAIT_EE) achieved significantly greater improvements than conventional walking rehabilitation (mean difference [MD] = 0.16 m/s, 95% CI = [0.04, 0.28]). None of the other interventions improved walking speed significantly.
Functional electrical stimulation (FES) has also been used in motor rehabilitation therapy over the last few decades. Passive FES therapy can reduce muscle spasms and shorten the term of motor recovery (Hong et al., 2018). Passive therapies such as continuous passive motion or cycling therapy have been employed for patients and showed functional improvements in previous studies (Janssen et al., 2008; Yeh et al., 2010; Ambrosini et al., 2011). However, they do not include devices or systems to monitor the patient’s active engagement in the therapy.
Today, Brain-Computer Interfaces (BCIs) can provide an objective tool for measuring Motor Imagery (MI), creating new possibilities for “closed-loop” feedback (Wolpaw and Wolpaw, 2012). Closed-loop feedback depends on sensing the desired mental activity and is possible with MI-based BCIs, which could significantly improve rehabilitation therapy outcomes (Ortner et al., 2012; Cho et al., 2016; Cantillo-Negrete et al., 2018; Irimia et al., 2018).
MI-based BCIs have been employed in rehabilitation training for stroke patients to fill the gap between patients’ expectations and therapy outcomes. In conventional rehabilitation therapies, patients are often asked to try to move the paretic limb, or to imagine moving it, while a FES, physiotherapist and/or robotic device helps them to perform the desired movement. Their feedback is often provided when the users are not performing the required mental activity. There is no objective way to determine whether patients who cannot move are actively performing the desired motor imagery (MI) task and thus producing concordant neural activation. Its efficacy has been shown in multiple studies implementing exoskeleton, orthosis or robots which induce passive movement of their affected limbs (Ramos-Murguialday et al., 2013; Ono et al., 2014; Ang et al., 2015). During repetitive neurofeedback training sessions, even patients with severe impairment could complete the sensorimotor loop in their brains linking coherent sensory feedback with motor intention (Cho et al., 2016; Pichiorri et al., 2017; Irimia et al., 2018).
This concurrent sensory feedback with motor intention is an important factor for motor recovery (Ortner et al., 2012; Bolognini et al., 2016; Pichiorri et al., 2017; Cantillo-Negrete et al., 2018; Irimia et al., 2018). Concurrent feedback based on users’ intention may help them learn mental strategies associated with movement and BCI use, which can affect results (Neuper et al., 2005; Neuper and Allison, 2014). Neural networks are strengthened when the presynaptic and postsynaptic neurons are both active. In conventional therapies, when patients receive feedback while they are not performing MI, these two neuronal networks are not simultaneously firing. This dissociation between motor commands and sensory feedback may explain why the therapy does not significantly induce the reorganization of the patients’ brains around their lesioned area. Non-simultaneous, dissociated feedback cannot underlie the Hebbian learning between two neuronal populations that underlies the desired improvements from rehabilitation (Mayford et al., 2012; Wolpaw and Wolpaw, 2012). Thus, conventional therapies may sometimes fail because they rely on open-loop feedback.
This clinical trial investigated the impact of combining BCI technology with MI and FES feedback for motor recovery of the lower limbs. The patients’ real-time sensory feedback depended on their movement intention. We explore the relationship between the proposed rehabilitation method and rehabilitation results, including changes in walking speed. Patients who use the training mode may have better motor outcomes, and these outcomes will be compared with those from patients who had EGAIT_EE therapy. […]

[WEB] What should a stroke survivor know about the recovery plateau?
Posted by Kostas Pantremenos in Neuroplasticity, Recovery Plateau on October 29, 2023

Zachary Smith, MS, CCC-SLP |
So, you feel stuck…
Whether you’re a brain injury or stroke survivor, caregiver for someone with neurological damage, or a healthcare worker, you’ve probably heard the term “recovery plateau” when talking about the recovery process. Right after a neurological event, things can be very challenging, and the first few months afterward are when you’ll typically see the most rapid progress. During this time, people often dive into intense, acute rehab to make the most of this window and try to improve their speech, mobility, and dexterity, as much as possible.
But here’s the twist: around six months after the initial injury, stroke survivors might start hearing the term “plateau” tossed around. You might be wondering, “What exactly does that mean?” If so, don’t stress—this post is here to demystify the concept of the neurological recovery plateau and give you the information you need to take your next steps forward with confidence!
What is the neurological recovery plateau?
When we talk about stroke recovery, “plateau” is often used to describe a phase when progress starts to flatten out or slow down. Right after a neurological event, recovery can be quite rapid. But at some point, stroke survivors can feel like things aren’t getting better as quickly. During this time, you might find yourself working on the same skills in therapy sessions over and over again. Your therapist might even mention the possibility of ending therapy, leading you to wonder: “Where do I go from here?”
Will stroke survivors be stuck in a plateau forever?
Stroke survivors, we hear you: Recovery plateaus are no fun. If it feels like you’re not progressing, you might start to think that where you are now is where you will always be. But is that really true?
The short answer is: No!
What does the research say about stroke survivors and recovery plateaus?
At BrainWire, we’re constantly in awe of the brain’s resilience and complexity—and recovery plateaus are no exception! Even if it’s been a while since your neurological event, there’s solid evidence that shows you can keep making progress! A study by Basso & Macis (2011) found that people with chronic aphasia can still make neurological process through an intensive program, even after being previously discharged from speech therapy. Likewise, research from Santhanam et al (2018) found that people were able to benefit from the effects of neuroplasticity even if their stroke had occurred more than a decade prior! To cap it off, Moss & Nicholas (2006) found that the amount of time that’s passed since a stroke does not determine how much therapy can help you.
So, when you’re feeling disheartened, remember: Your brain can find ways to rewire itself to get better, even if it’s been a while since your neurological injury!
What steps can I take if I’ve met a recovery plateau?
If you’re in a recovery plateau and you’ve been wondering, “Should I keep going with or restart therapy?”, the answer is a resounding yes! The studies mentioned above are just a taste of the research within the field that shows that therapy can make an impact even years after your neurological event. In other words, that idea of “this is where I’ll always be stuck” doesn’t really apply.
Recovery isn’t a one-size-fits-all journey, and each stroke survivor takes a different route to reach their goals. While traditional speech therapy can be effective, sometimes clinicians working within healthcare institutions have their hands tied due to insurance restrictions. While the science is clear that there is no linear path to recovery, insurance companies often require evidence of rapid and steady progress to continue covering services.
But your recovery journey doesn’t have to be stymied if you find yourself in that situation! Constant Therapy can step in to help you continue striving toward your speech, language, and cognitive goals. With Constant Therapy, stroke survivors have an entire library of evidence-based exercises at their fingertips, and you can move through them at your own pace! This means that you can keep getting therapy right from your home, and you move from “I are where I am” to “I am working towards where I want to be!”
Give Constant Therapy a try for free. Click here to start your 14-day trial today.
References
Basso, A. & Macis, M. (2011). Therapy efficacy in chronic aphasia. Behavioral Neurology, 24, 317-325.
Moss, A. & Nicholas, M. (2006). Language rehabilitation in chronic aphasia and time postonset: A review of single-subject data. Stroke, 37, 3043-3051.
Santhanam, P., Duncan, E.S., & Small, S.L. (2018). Therapy-induced plasticity in chronic aphasia is associated with behavioral improvement and time since stroke. Brain Connect, 8(3), 179-188.
[ARTICLE] A Pilot Feasibility Trial of an Upper Extremity Assistive System – Full Text
Posted by Kostas Pantremenos in Functional Electrical Stimulation (FES), REHABILITATION on October 28, 2023
Abstract
Objective
: To develop and clinically evaluate a customizable active upper extremity (UE) assistive system with integrated functional electrical stimulation (FES) that improves function and independence of individuals during activities of daily living (ADLs).
Design
: Single-arm, prospective, open-label cohort feasibility trial.
Setting
: An academic research institution.
Participants
: Subjects were five adults with a medical history of stroke resulting in distal UE impairment. The subjects volunteered from recruitment materials which detailed information about the study.
Interventions
: A novel, wearable, lightweight, low-profile, and patient-tailored UE assistive system. It comprises a splint component and FES unit that may each be controlled by electromyography (EMG) signals, inertial measurement units (IMU), manual control source (joystick), and/or voice control.
Main Outcome Measure(s)
: Several occupational therapy outcome measures were used, including the Canadian Occupational Performance Measure (COPM), Action Research Arm Test (ARAT), The Box and Blocks Test (BBT), the ABILHAND-Manual Ability Measure, and Patient Reported Outcomes Measurement Information System (PROMIS) Upper Extremity Short Form.
Results
: All participants learned to utilize our UE assistive system to perform ADLs and were able to use it independently at home. Most participants experienced a clinically meaningful improvement in both performance and satisfaction for the majority of their COPM goals while using the system. All participants experienced improvement in hand grip and release as shown by their baseline and post assessment scores for hand function (BBT, ARAT) and patient reported outcomes (ABILHAND, PROMIS).
Conclusion(s)
: The clinical outcomes suggest that our UE assistive system improves functional performance in patients with UE impairment, allowing them to engage more actively in ADLs. Further innovation including elbow and shoulder components will allow users to have more degrees of freedom during tasks.
Figure 2. The NuroSleeve consists of the main control unit (center) which accepts different input control signals (left) to control one or more end effectors (right). Implementations are customized for each patient by the occupational therapist using the clinical configuration software.
[WEB] Company Raises $12 Million to Fund Adoption of Neural Sleeve
Posted by Kostas Pantremenos in Assistive Technology, Gait Rehabilitation - Foot Drop, Neuroplasticity on October 24, 2023
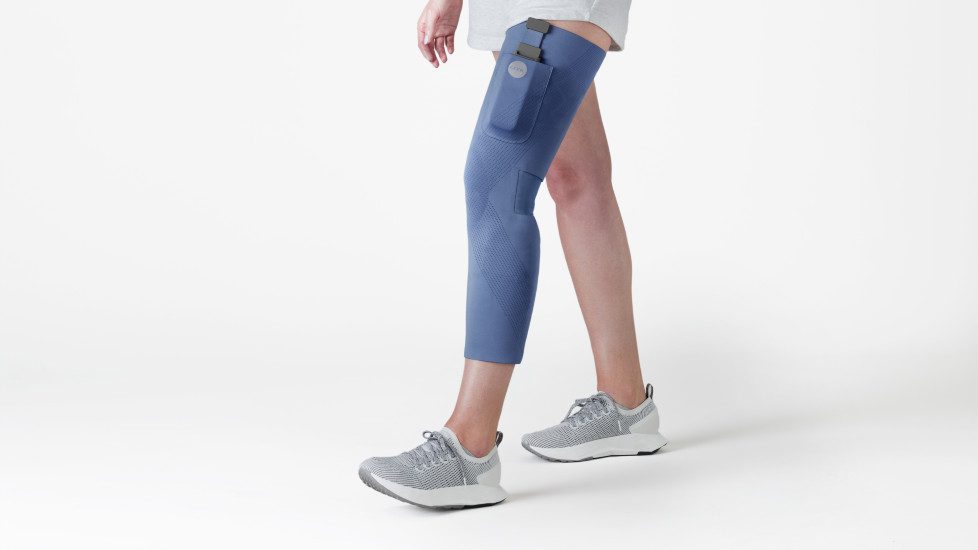
Cionic, the neurotech company that created the FDA-cleared Cionic Neural Sleeve, announced an additional $12 million extension of its Series A financing, bringing the Series A total to $25 million. Part of the funds will be used to grow user and clinician adoption of the Cionic Neural Sleeve for people experiencing impaired mobility as a result of multiple sclerosis, strokes, cerebral palsy, and other neurological conditions.
The round was led by L Catterton, a leading consumer-focused investment firm, and joined by an additional new investor THVC, as well as all major investors. Additionally, Cionic has also partnered with Enable Ventures, the first impact venture fund dedicated to closing the disability wealth gap while achieving competitive, market-rate returns.
As part of L Catterton’s investment, Venture Partner and experienced entrepreneur Whitney Casey has joined Cionic’s board of directors.
“Transformative technologies are powering a new category of consumer medical devices that assist anywhere it is needed, and at any time,” says Whitney Casey, venture partner at L Catterton. “We have seen how Cionic’s unique bionic clothing has already transformed the lives of those living with mobility impairments, and with the growing consumer interest in wearable health technology, Cionic’s platform has the potential to change the trajectory of personalized healthcare, and clothing, as we know it.”
In addition to using funds from the Series A extension to grow user and clinician adoption of the Cionic Neural Sleeve, Cionic will also use the funds to accelerate the company’s product pipeline into new indications addressing the full spectrum of human mobility. Powering this roadmap is continued investment in Cionic’s wearable neuromodulation platform.
Cionic has doubled its headcount in the past six months and established a state-of-the-art manufacturing facility in Northern California. The company is now licensed to ship the Cionic Neural Sleeve to 45 states in the US, with over 400 prescribing institutions. Since the start of shipments in early 2023, Cionic customers have already clocked over 200,000 hours of use.
“There is a revolution underway in healthcare and with it a growing demand for brands that provide not only high-quality care where and when it is needed, but that are also capable of measuring progress and proving outcomes,” says Jeremiah Robison, founder & CEO of Cionic. “Our partners recognize that we are building something truly unique, and we are proud to work with organizations who share not only our vision for the future of healthcare, but whose deep expertise in brand building, healthcare transformation, and disability advocacy will help us accelerate the timeline for making this vision a reality.”
“The confluence of rising societal expectations of people with disabilities plus technological innovation that is driving systems change, and significant capital earmarked for DEI makes this moment the ideal time to pursue this profoundly underserved market,” says Regina “Gina” Kline, founder and managing partner at Enable Ventures. “Throughout history, disability has served as a catalyst for innovation and Cionic is a leading example. In helping his daughter, Jeremiah has addressed the mobility needs of more than 35 million people in the United States alone and has unlocked the potential of bionic clothing. Enable seeks to support enterprises like Cionic which truly represents the intersection of disability and epic technology.”
[ARTICLE] Virtual Reality Music Instrument Playing Game for Upper Limb Rehabilitation Training – Full Text
Posted by Kostas Pantremenos in Music/Music therapy, Paretic Hand, Video Games/Exergames, Virtual reality rehabilitation on October 23, 2023
The motor function of the upper limb is typically impaired in stroke patients; as a result, rehabilitation exercise is crucial to regaining muscular control. While encouraging patients to continue with long-term exercise using standard rehabilitation training methods may be difficult. To deal with this dilemma, virtual reality (VR) games are introduced to motivate patients to take part in therapy. Meanwhile, music therapy has been proven to be extremely beneficial in the early phases of stroke recovery. These activities inspire us to include musical instrument play like xylophone and drums, in the design of VR games. By striking the xylophone’s highlighted keys or the flying notes aimed at the drums, the impaired upper limb functions can be strengthened. Early user evaluations demonstrate that the developed games are straightforward to use and appeal to patients’ desire for more exercise.
1 INTRODUCTION
Tangible games are widely utilized to motivate patients and track their performance to support therapists better [9, 10, 11], while some recent research studies also adopt virtual reality (VR) games in redesigning upper limb exercises. VR games and actual movements can be integrated together to motivate patients in rehabilitation exercises. Rose et al. [7] reported that patient enjoyment and willingness to participate were concluded in healthcare plans incorporating VR due to its immersive, entertaining approach to improving performance. However, some VR games may provide repetitive, intense, and task-specific training to enhance neuroplasticity [8]. In order to mitigate this issue, music therapy, which has been demonstrated to aid in both physical and mental rehabilitation, has been proven to be extremely beneficial in the early phases of stroke recovery [4]. Both sorts of engagement can benefit stroke patients, but generally speaking, low-cost methods have more real-world use. The price of VR-based headset has been extremely expensive in the past. With the improvement of technology, a few cost-effective VR devices are launched in the market, such as PICO4 (an all-in-one device around $425 as shown in Fig. 1), which creates more opportunities for VR game development. In this study, we focus on rhythm-based upper-limb training exercises by incorporating musical instrument playing into VR game design. As a result, the two musical instruments, i.e., the xylophone and drums, are applied to the game design with tactile, auditory, and visual feedback.
2 RELATED WORKS
Projects like TangiBoard demonstrated how sensory technology and tangibles can generally enrich learning and training experience in upper limb rehabilitation [5, 6]. In recent years, many projects aimed to tackle similar problems using VR technology by picking up and positioning objects in the virtual environment at specific places [6]. For example, the Bimeo gadget provided a VR environment to encourage patients to rehabilitation exercise, as well as support therapists to oversee and manage the exercise [1]. The ArmeoSenso system [5] similarly used VR and inertial measurement unit (IMU) for video game-based training and assessment of upper limb functions. VR games have been explored as tools in rehabilitation training.
Playing therapeutic instrumental music assists patients in regaining functional movement patterns and damaged motor functions [3]. Connie Tomaino, the director of the Beth Abraham Music and Neurologic Rehabilitation Institute, states that “focusing attention on rhythmic instruments can increase movement in individuals such as those with Parkinson’s disease or stroke rehabilitation patients” [2]. In music therapy, drums and xylophone are very popular instruments since people without prior knowledge can quickly learn how to play. In fact, stroke rehabilitation patients may exercise more if they concentrate on rhythmic instruments [2]. As a result, we decided to build a rhythm-based VR game using drums and xylophone play for rehabilitation exercise.
3 CONCEPTUAL DESIGN AND GAME PROTOTYPING
We observed patients performing arm-reaching exercises while conducting field research at a local rehabilitation facility, Suzhou Municipal Hospital, by moving a wooden instrument on the table. This exercise is vital to inhibit muscular contraction in the initial stages of stroke recovery. Patients moved from one posture to another as directed by therapists verbally. Even under the care of therapists, patients were quite inactive, although they could exercise independently. To sum up, we identify the design opportunity as providing a low-cost training device that motivates and guides patients through active exercising tasks. Meanwhile, therapists should be able to monitor multiple patients simultaneously and record their performances.
As a result, we created a VR game concept utilizing PICO4 to encourage them to complete the practice. The stroke patients held two controllers that weighed 185 grams each while wearing headsets. Through gripping the controllers, users can play virtual music instruments for upper limb reaching, stretching and extension. PICO4 device can mirror the VR display from the headsets to other devices such as televisions, computers, and smartphones. With this screen mirroring capability, clinicians could not only provide guidance and assistance to patients, but also monitor their gaming performance in real-time. Two distinct game modes are primarily designed: ‘Xylophone Play Mode’ and ‘Drums Play Mode’ to support appropriate upper limb functional training. Two iterations of VR game design are explored to facilitate arm reaching, shoulder extension, wrist and elbow rotation exercises.

3.1 First Edition
In the ‘Xylophone Play Mode’, patients move virtual mallets by arm movement to strike the keys. A melody can be generated by pointing, rotating the wrist, and moving the mallet up and down to strike the keys. This game can improve upper limb-eye coordination and fine motor control.
In the ‘Drums Play Mode’, the rhythmical notes fly and move directly towards the corresponding drums with the background music. Patients use the virtual drumsticks to catch those notes above the drums, and successful strikes are rewarded with points. Clinicians can gauge the patients’ progress based on the scores received and decide whether they can move on to more challenging levels. For user-intuitive feedback, a successful note-catching would trigger a drum beat sound with controller vibration and an explosion effect. We tested our VR game in Suzhou Municipal Hospital Rehabilitation Center and received the following therapist response. Task-driven functionality, such as highlighting particular keys on xylophone to guide users exercise, should be included in the ‘Xylophone Play Mode’. The flight speed of such rhythmical notes in the ‘Drums Play Mode’ is too quick, which causes much miss catching in the exercise. As a result, two difficulty levels are designed for this mode in the revised version: basic level and standard level.
[WEB] Parkinson’s Disease – Early Detection and Monitoring with KeySense
Posted by Kostas Pantremenos in Uncategorized on October 23, 2023

® KeySense is a registered trademark of Parkinson’s Foundation (Australia) © Copyright 2023 Parkinson’s Foundation (Australia)
Parkinson’s Disease
Early Detection and Monitoring with KeySense
Parkinson’s disease is a progressive neurodegenerative disorder that affects movement, motor skills, and coordination. It is the second-most common neurodegenerative disease after Alzheimer’s, affecting over 10 million people worldwide. The symptoms of Parkinson’s disease can vary from person to person, but visible changes may include tremors, rigidity, bradykinesia (slowness of movement), and postural instability. The disease progresses slowly over time, and the symptoms become more severe. If you are concerned that you or a loved one may have Parkinson’s disease, we encourage you to take the KeySense assessment. It is free and can be taken from the comfort of your own home.
Disclaimer: KeySense is just an assessment tool. It does not provide a medical diagnosis and the results can not be used as such. Only a medical practitioner can establish whether or not you actually have Parkinson’s Disease.
GPs, on the other hand, were only 75% accurate in their diagnoses. KeySense can also detect early Parkinson’s up to 5 years before usual diagnosis. This means that KeySense can help people get the treatment and support they need early on.
You don’t need to be a good typist to do the assessment
The KeySense techniques don’t care about how quickly you type or how many mistakes you make. Even slow, two-finger typing is fine – we are mainly assessing the pattern of finger rhythms. We will ask you to transcribe a passage of text, then you will have the KeySense results immediately. The results are shown as scores across multiple movement attributes – it is not a pass/fail assessment or a diagnosis, but shows how you compare to others. Have a look at a sample report here
The Problem
There are some key challenges though in diagnosing Parkinson’s disease:The symptoms are often subtle and difficult to detect in the early stages.There is no single, objective, diagnostic test for Parkinson’s disease.The diagnosis of Parkinson’s disease is often delayed by years, and someone may have it for a decade or more before it’s finally diagnosed. Although there is currently no cure for Parkinson’s disease, once diagnosed there are medications that can help manage the symptoms, along with lifestyle changes that can improve quality of life for people with Parkinson’s disease.
The KeySense Solution
1. Simplicity
Our KeySense technology provides a simple, non-invasive, and accurate way to detect Parkinson’s disease in its early stages. KeySense analyzes the rhythm and cadence of your keystrokes as you type on a computer keyboard. This data is then used to create a personalized risk score for Parkinson’s disease. The KeySense test is quick and easy to take. Simply type some paragraphs of text on your own computer. The assessment takes about 10 minutes or so to complete, and your results are available immediately
2. Accuracy
The KeySense test is typically more accurate than a GP’s diagnosis. KeySense has been used by more than 40,000 people already and, in the latest analysis, has 90% accuracy in detecting Parkinson’s disease in its early stages.
GET STARTED NOW
See whether the way you type shows any indications of early Parkinson’s Disease. Less than a page is needed for immediate analysis, based on characteristics and rhythms in your typing. It will take about 10 minutes to complete. We don’t ask you for any personal details, so you remain completely anonymous.
Source: https://www.parkinsons-research.org/?gclid=EAIaIQobChMI9tLHmKCKggMVosbnAx1KMwXLEAEYASAAEgJ3zvD_BwE
[BLOG POST] Smartphone app quickly analyzes human motion to aid physical rehab
Posted by Kostas Pantremenos in Apps, Gait Rehabilitation - Foot Drop, REHABILITATION on October 20, 2023
A research team funded by the National Institutes of Health has developed a smart phone app that can track and analyze a person’s ability to move from one place to another, known as locomotion, and other types of movements. Human motion analysis is used to evaluate patients with movement difficulties, to help clinicians plan surgery, and to assess the results of treatment procedures. The research team believes that using the app costs about 1% of conventional motion analysis techniques and works 25 times faster. The study appears in PLOS Computational Biology.
[Abstract + References] A new adaptive VR-based exergame for hand rehabilitation after stroke
Posted by Kostas Pantremenos in Paretic Hand, REHABILITATION, Video Games/Exergames, Virtual reality rehabilitation on October 18, 2023
Abstract
The aim of this work is to present an adaptive serious game based on virtual reality (VR) for functional rehabilitation of the hand after stroke. The game focuses on simulating the palmar grasping exercise commonly used in clinical settings. The system’s design follows a user-centered approach, involving close collaboration with functional rehabilitation specialists and stroke patients. It uses the Leap motion controller to enable patient interaction in the virtual environment, which was created using the Unity 3D game engine. The system relies on hand gestures involving opening and closing movements to interact with virtual objects. It incorporates parameters to objectively measure participants’ performance throughout the game session. These metrics are used to personalize the game’s difficulty to each patient’s motor skills. To do this, we implemented an approach that dynamically adjusts the difficulty of the exergame according to the patient’s performance during the game session. To achieve this, we used an unsupervised machine learning technique known as clustering, in particular using the K-means algorithm. By applying this technique, we were able to classify patients’ performance into distinct groups, enabling us to assess their skill level and adapt the difficulty of the game accordingly. To evaluate the system’s effectiveness and reliability, we conducted a subjective evaluation involving 11 stroke patients. The standardized System Usability Scale (SUS) questionnaire was used to assess the system’s ease of use, while the Intrinsic Motivation Inventory (IMI) was used to evaluate the participants’ subjective experience with the system. Evaluations showed that our proposed system is usable and acceptable on a C-level scale, with a good adjective score, and the patients perceived a high intrinsic motivation.
References
- Montoya, M.F., Munoz, J.E., Henao, O.A.: Enhancing virtual rehabilitation in upper limbs with biocybernetic adaptation: the effects of virtual reality on perceived muscle fatigue, game performance and user experience. IEEE Trans. Neural Syst. Rehabil. Eng. 28, 740–747 (2020). https://doi.org/10.1109/TNSRE.2020.2968869Article Google Scholar
- Then, J.W., Shivdas, S., Tunku Ahmad Yahaya, T.S., Ab Razak, N.I., Choo, P.T.: Gamification in rehabilitation of metacarpal fracture using cost-effective end-user device: a randomized controlled trial. J. Hand Therap. 33, 235–242 (2020). https://doi.org/10.1016/j.jht.2020.03.029
- Goršič, M., Cikajlo, I., Novak, D.: Competitive and cooperative arm rehabilitation games played by a patient and unimpaired person: effects on motivation and exercise intensity. J NeuroEng. Rehabil. 14, 23 (2017). https://doi.org/10.1186/s12984-017-0231-4Article Google Scholar
- Alarcón-Aldana, A.C., Callejas-Cuervo, M., Bo, A.P.L.: Upper limb physical rehabilitation using serious videogames and motion capture systems: a systematic review. Sensors. 20, 5989 (2020). https://doi.org/10.3390/s20215989Article Google Scholar
- Bortone, I., Leonardis, D., Solazzi, M., Procopio, C., Crecchi, A., Bonfiglio, L., Frisoli, A.: Integration of serious games and wearable haptic interfaces for Neuro Rehabilitation of children with movement disorders: A feasibility study. In: 2017 International Conference on Rehabilitation Robotics (ICORR). pp. 1094–1099. IEEE, London (2017)
- Avola, D., Cinque, L., Foresti, G.L., Marini, M.R.: An interactive and low-cost full body rehabilitation framework based on 3D immersive serious games. J. Biomed. Inform. 89, 81–100 (2019). https://doi.org/10.1016/j.jbi.2018.11.012Article Google Scholar
- Ribé, J.T., Serrancolí, G., Arriaga, I.A., Alpiste, F., Iriarte, A., Margelí, C., Mayans, B., García, A., Morancho, S., Molas, C.: Design and Development of Biomedical Applications for Post-Stroke Rehabilitation Subjects
- Ferreira, B., Menezes, P.: An Adaptive Virtual Reality-Based Serious Game for Therapeutic Rehabilitation. Int. J. Onl. Eng. 16, 63 (2020). https://doi.org/10.3991/ijoe.v16i04.11923Article Google Scholar
- Pillai, M., Yang, Y., Ditmars, C., Subhash, H.M.: Artificial intelligence-based interactive virtual reality-assisted gaming system for hand rehabilitation. In: Deserno, T.M. and Chen, P.-H. (eds.) Medical Imaging 2020: Imaging Informatics for Healthcare, Research, and Applications. p. 18. SPIE, Houston, United States (2020)
- Piette, P., Pasquier, J.: Réalité virtuelle et rééducation. Kinésithérapie, la Revue. 12, 38–41 (2012). https://doi.org/10.1016/j.kine.2012.07.003Article Google Scholar
- Kim, W.-S., Cho, S., Ku, J., Kim, Y., Lee, K., Hwang, H.-J., Paik, N.-J.: Clinical Application of Virtual Reality for Upper Limb Motor Rehabilitation in Stroke: Review of Technologies and Clinical Evidence. JCM. 9, 3369 (2020). https://doi.org/10.3390/jcm9103369Article Google Scholar
- Ning, H., Wang, Z., Li, R., Zhang, Y., Mao, L.: A Review on Serious Games for Exercise Rehabilitation. arXiv:2201.04984 [cs]. (2022)
- Amorim, P., Sousa Santos, B., Dias, P., Silva, S., Martins, H.: Serious Games for Stroke Telerehabilitation of Upper Limb – A Review for Future Research. Int J Telerehab. 12, 65–76 (2020). https://doi.org/10.5195/ijt.2020.6326Article Google Scholar
- Lawrence, E.S., Coshall, C., Dundas, R., Stewart, J., Rudd, A.G., Howard, R., Wolfe, C.D.A.: Estimates of the Prevalence of Acute Stroke Impairments and Disability in a Multiethnic Population. Stroke 32, 1279–1284 (2001). https://doi.org/10.1161/01.STR.32.6.1279Article Google Scholar
- Avola, D., Cinque, L., Pannone, D.: Design of a 3D Platform for Immersive Neurocognitive Rehabilitation. Information 11, 134 (2020). https://doi.org/10.3390/info11030134Article Google Scholar
- Zahabi, M., Abdul Razak, A.M.: Adaptive virtual reality-based training: a systematic literature review and framework. Virtual Reality 24, 725–752 (2020). https://doi.org/10.1007/s10055-020-00434-wArticle Google Scholar
- Debnath, B., O’Brien, M., Yamaguchi, M., Behera, A.: A review of computer vision-based approaches for physical rehabilitation and assessment. Multimedia Syst. 28, 209–239 (2022). https://doi.org/10.1007/s00530-021-00815-4Article Google Scholar
- Rincon, A.L., Yamasaki, H., Shimoda, S.: Design of a video game for rehabilitation using motion capture, EMG analysis and virtual reality. In: 2016 International Conference on Electronics. Communications and Computers (CONIELECOMP), pp. 198–204. IEEE, Cholula, Mexico (2016)
- Trombetta, M., Bazzanello Henrique, P.P., Brum, M.R., Colussi, E.L., De Marchi, A.C.B., Rieder, R.: Motion Rehab AVE 3D: A VR-based exergame for post-stroke rehabilitation. Computer Methods and Programs in Biomedicine. 151, 15-20 (2017). https://doi.org/10.1016/j.cmpb.2017.08.008
- Hashim, S.H.M., Ismail, M., Manaf, H., Hanapiah, F.A.: Development of Dual Cognitive Task Virtual Reality Game Addressing Stroke Rehabilitation. In: Proceedings of the 2019 3rd International Conference on Virtual and Augmented Reality Simulations. pp. 21-25. ACM, Perth WN Australia (2019
- Ma, M., Zheng, H.: Virtual Reality and Serious Games in Healthcare. In: Brahnam, S. and Jain, L.C. (eds.) Advanced Computational Intelligence Paradigms in Healthcare 6. Virtual Reality in Psychotherapy, Rehabilitation, and Assessment. pp. 169-192. Springer Berlin Heidelberg, Berlin, Heidelberg (2011)
- Gonçalves, A.R., Muñoz, J.E., Gouveia, É.R., Cameirão, M. da S., Bermúdez i Badia, S.: Effects of prolonged multidimensional fitness training with exergames on the physical exertion levels of older adults. Vis Comput. 37, 19-30 (2021). https://doi.org/10.1007/s00371-019-01736-0
- Hossain, M.S., Hardy, S., Alamri, A., Alelaiwi, A., Hardy, V., Wilhelm, C.: AR-based serious game framework for post-stroke rehabilitation. Multimedia Syst. 22, 659–674 (2016). https://doi.org/10.1007/s00530-015-0481-6Article Google Scholar
- Streicher, A., Smeddinck, J.D.: Personalized and Adaptive Serious Games. In: Dörner, R., Göbel, S., Kickmeier-Rust, M., Masuch, M., Zweig, K. (eds.) Entertainment Computing and Serious Games, pp. 332–377. Springer International Publishing, Cham (2016)Chapter Google Scholar
- Hocine, N., Gouaïch, A., Cerri, S.A., Mottet, D., Froger, J., Laffont, I.: Adaptation in serious games for upper-limb rehabilitation: an approach to improve training outcomes. User Model. User-Adap. Inter. 25, 65–98 (2015). https://doi.org/10.1007/s11257-015-9154-6Article Google Scholar
- Thielbar, K.O., Lord, T.J., Fischer, H.C., Lazzaro, E.C., Barth, K.C., Stoykov, M.E., Triandafilou, K.M., Kamper, D.G.: Training finger individuation with a mechatronic-virtual reality system leads to improved fine motor control post-stroke. J NeuroEngineering Rehabil. 11, 171 (2014). https://doi.org/10.1186/1743-0003-11-171Article Google Scholar
- Sinaga, K.P., Yang, M.-S.: Unsupervised K-Means Clustering Algorithm. IEEE. Access 8, 80716–80727 (2020). https://doi.org/10.1109/ACCESS.2020.2988796Article Google Scholar
- Colombo, R., Pisano, F., Micera, S., Mazzone, A., Delconte, C., Carrozza, M.C., Dario, P., Minuco, G.: Robotic techniques for upper limb evaluation and rehabilitation of stroke patients. IEEE Trans. Neural Syst. Rehabil. Eng. 13, 311–324 (2005). https://doi.org/10.1109/TNSRE.2005.848352Article Google Scholar
- Sunderland, A., Tinson, D., Bradley, L., Hewer, R.L.: Arm function after stroke. An evaluation of grip strength as a measure of recovery and a prognostic indicator. Journal of Neurology, Neurosurgery & Psychiatry. 52, 1267-1272 (1989). https://doi.org/10.1136/jnnp.52.11.1267
- Kim, H., Miller, L.M., Fedulow, I., Simkins, M., Abrams, G.M., Byl, N., Rosen, J.: Kinematic Data Analysis for Post-Stroke Patients Following Bilateral Versus Unilateral Rehabilitation With an Upper Limb Wearable Robotic System. IEEE Trans. Neural Syst. Rehabil. Eng. 21, 153–164 (2013). https://doi.org/10.1109/TNSRE.2012.2207462Article Google Scholar
- Kong, K.-H., Lee, J.: Temporal recovery and predictors of upper limb dexterity in the first year of stroke: A prospective study of patients admitted to a rehabilitation centre. NRE. 32, 345–350 (2013). https://doi.org/10.3233/NRE-130854Article Google Scholar
- Bigoni, M., Baudo, S., Cimolin, V., Cau, N., Galli, M., Pianta, L., Tacchini, E., Capodaglio, P., Mauro, A.: Does kinematics add meaningful information to clinical assessment in post-stroke upper limb rehabilitation? A case report. J Phys Ther Sci. 28, 2408–2413 (2016). https://doi.org/10.1589/jpts.28.2408
- Sucar, L.E., Luis, R., Leder, R., Hernáindez, J., Sáinchez, I.: Gesture therapy: A vision-based system for upper extremity stroke rehabilitation. In: 2010 Annual International Conference of the IEEE Engineering in Medicine and Biology. pp. 3690-3693. IEEE, Buenos Aires (2010)
- Housman, S.J., Scott, K.M., Reinkensmeyer, D.J.: A Randomized Controlled Trial of Gravity-Supported, Computer-Enhanced Arm Exercise for Individuals With Severe Hemiparesis. Neurorehabil. Neural Repair 23, 505–514 (2009). https://doi.org/10.1177/1545968308331148Article Google Scholar
- Kong, K.-H., Loh, Y.-J., Thia, E., Chai, A., Ng, C.-Y., Soh, Y.-M., Toh, S., Tjan, S.-Y.: Efficacy of a Virtual Reality Commercial Gaming Device in Upper Limb Recovery after Stroke: A Randomized. Controlled Study. Topics in Stroke Rehabilitation. 23, 333–340 (2016). https://doi.org/10.1080/10749357.2016.1139796Article Google Scholar
- Rand, D., Givon, N., Weingarden, H., Nota, A., Zeilig, G.: Eliciting Upper Extremity Purposeful Movements Using Video Games: A Comparison With Traditional Therapy for Stroke Rehabilitation. Neurorehabil. Neural Repair 28, 733–739 (2014). https://doi.org/10.1177/1545968314521008Article Google Scholar
- Broeren, J., Claesson, L., Goude, D., Rydmark, M., Sunnerhagen, K.S.: Virtual Rehabilitation in an Activity Centre for Community-Dwelling Persons with Stroke. Cerebrovasc. Dis. 26, 289–296 (2008). https://doi.org/10.1159/000149576Article Google Scholar
- Sin, H., Lee, G.: Additional Virtual Reality Training Using Xbox Kinect in Stroke Survivors with Hemiplegia. American Journal of Physical Medicine & Rehabilitation. 92, 871–880 (2013). https://doi.org/10.1097/PHM.0b013e3182a38e40Article Google Scholar
- Kwon, J.-S., Park, M.-J., Yoon, I.-J., Park, S.-H.: Effects of virtual reality on upper extremity function and activities of daily living performance in acute stroke: A double-blind randomized clinical trial. NRE. 31, 379–385 (2012). https://doi.org/10.3233/NRE-2012-00807Article Google Scholar
- Lee, S., Kim, Y., Lee, B.-H.: Effect of Virtual Reality-based Bilateral Upper Extremity Training on Upper Extremity Function after Stroke: A Randomized Controlled Clinical Trial: Bilateral Upper Extremity Training in Post Stroke. Occup. Ther. Int. 23, 357–368 (2016). https://doi.org/10.1002/oti.1437Article Google Scholar
- Subramanian, S.K., Lourenço, C.B., Chilingaryan, G., Sveistrup, H., Levin, M.F.: Arm Motor Recovery Using a Virtual Reality Intervention in Chronic Stroke: Randomized Control Trial. Neurorehabil. Neural Repair 27, 13–23 (2013). https://doi.org/10.1177/1545968312449695Article Google Scholar
- Zheng, C., Liao, W., Xia, W.: Effect of combined low-frequency repetitive transcranial magnetic stimulation and virtual reality training on upper limb function in subacute stroke: a double-blind randomized controlled trail. J. Huazhong Univ. Sci. Technol. [Med. Sci.]. 35, 248-254 (2015). https://doi.org/10.1007/s11596-015-1419-0
- Piron, L., Turolla, A., Agostini, M., Zucconi, C., Cortese, F., Zampolini, M., Zannini, M., Dam, M., Ventura, L., Battauz, M., Tonin, P.: Exercises for paretic upper limb after stroke: A combined virtual-reality and telemedicine approach. J. Rehabil. Med. 41, 1016–102 (2009). https://doi.org/10.2340/16501977-0459Article Google Scholar
- Brunner, I., Skouen, J.S., Hofstad, H., Aßmus, J., Becker, F., Sanders, A.-M., Pallesen, H., Qvist Kristensen, L., Michielsen, M., Thijs, L., Verheyden, G.: Virtual Reality Training for Upper Extremity in Subacute Stroke (VIRTUES): A multicenter RCT. Neurology 89, 2413–2421 (2017). https://doi.org/10.1212/WNL.0000000000004744Article Google Scholar
- Choi, Y.-H., Ku, J., Lim, H., Kim, Y.H., Paik, N.-J.: Mobile game-based virtual reality rehabilitation program for upper limb dysfunction after ischemic stroke. RNN. 34, 455–463 (2016). https://doi.org/10.3233/RNN-150626Article Google Scholar
- Kiper, P., Agostini, M., Luque-Moreno, C., Tonin, P., Turolla, A.: Reinforced Feedback in Virtual Environment for Rehabilitation of Upper Extremity Dysfunction after Stroke: Preliminary Data from a Randomized Controlled Trial. Biomed. Res. Int. 2014, 1–8 (2014). https://doi.org/10.1155/2014/752128Article Google Scholar
- Ma, M., Bechkoum, K.: Serious games for movement therapy after stroke. In: 2008 IEEE International Conference on Systems. Man and Cybernetics, pp. 1872–1877. IEEE, Singapore, Singapore (2008)
- Nasri, N., Orts-Escolano, S., Cazorla, M.: An sEMG-Controlled 3D Game for Rehabilitation Therapies: Real-Time Time Hand Gesture Recognition Using Deep Learning Techniques. Sensors. 20, 6451 (2020). https://doi.org/10.3390/s20226451Article Google Scholar
- Dhiman, A., Solanki, D., Bhasin, A., Das, A., Lahiri, U.: An intelligent, adaptive, performance-sensitive, and virtual reality-based gaming platform for the upper limb. Comput Anim Virtual Worlds. 29, e1800 (2018). https://doi.org/10.1002/cav.1800Article Google Scholar
- Vallejo, D., Gmez-Portes, C., Albusac, J., Glez-Morcillo, C., Castro-Schez, J.J.: Personalized Exergames Language: A Novel Approach to the Automatic Generation of Personalized Exergames for Stroke Patients. Appl. Sci. 10, 7378 (2020). https://doi.org/10.3390/app10207378Article Google Scholar
- Afyouni, I., Murad, A., Einea, A.: Adaptive Rehabilitation Bots in Serious Games. Sensors. 20, 7037 (2020). https://doi.org/10.3390/s20247037Article Google Scholar
- Aguilar-Lazcano, C.A., Rechy-Ramirez, E.J.: Performance analysis of Leap motion controller for finger rehabilitation using serious games in two lighting environments. Measurement 157, 107677 (2020). https://doi.org/10.1016/j.measurement.2020.107677Article Google Scholar
- Rechy-Ramirez, E.J., Marin-Hernandez, A., Rios-Figueroa, H.V.: A human-computer interface for wrist rehabilitation: a pilot study using commercial sensors to detect wrist movements. Vis Comput. 35, 41–55 (2019). https://doi.org/10.1007/s00371-017-1446-xArticle Google Scholar
- Burke, J.W., McNeill, M.D.J., Charles, D.K., Morrow, P.J., Crosbie, J.H., McDonough, S.M.: Optimising engagement for stroke rehabilitation using serious games. Vis Comput. 25, 1085–1099 (2009). https://doi.org/10.1007/s00371-009-0387-4Article Google Scholar
- de los Reyes-Guzmàin, A., Lozano-Berrio, V., Alvarez-Rodríguez, M., López-Dolado, E., Ceruelo-Abajo, S., Talavera-Díaz, F., Gil-Agudo, A.: RehabHand: Oriented-tasks serious games for upper limb rehabilitation by using Leap Motion Controller and target population in spinal cord injury. NeuroRehabilitation 48(3), 365–373 (2021). https://doi.org/10.3233/NRE-201598
- Harvey, C., Selmanović, E., O’Connor, J., Chahin, M.: A comparison between expert and beginner learning for motor skill development in a virtual reality serious game. Vis Comput. 37, 3–17 (2021). https://doi.org/10.1007/s00371-019-01702-wArticle Google Scholar
- David, L., Bouyer, G., Otmane, S.: Towards a low-cost interactive system for motor self-rehabilitation after stroke. IJVR. 17, 40-45 (2017). https://doi.org/10.20870/IJVR.2017.17.2.2890
- Li, C., Cheng, L., Yang, H., Zou, Y., Huang, F.: An Automatic Rehabilitation Assessment System for Hand Function Based on Leap Motion and Ensemble Learning. Cybern. Syst. 52, 3–25 (2021). https://doi.org/10.1080/01969722.2020.1827798Article Google Scholar
- de Souza, M.R.S.B., Gonçalves, R.S., Carbone, G.: Feasibility and Performance Validation of a Leap Motion Controller for Upper Limb Rehabilitation. Robotics 10, 130 (2021). https://doi.org/10.3390/robotics10040130Article Google Scholar
- Koter, K., Samowicz, M., Redlicka, J., Zubrycki, I.: Hand Measurement System Based on Haptic and Vision Devices towards Post-Stroke Patients. 23 (2022)
- David, L.: Conception et évaluation d’un système de réalité virtuelle pour l’assistance à l’auto-rééducation motrice du membre supérieur post-AVC. 165
- Orhan, U., Hekim, M., Ozer, M.: EEG signals classification using the K-means clustering and a multilayer perceptron neural network model. Expert Syst. Appl. 38, 13475–13481 (2011). https://doi.org/10.1016/j.eswa.2011.04.149Article Google Scholar
- Bangor, A.: Determining What Individual SUS Scores Mean: Adding an Adjective Rating Scale. 4, 10 (2009)Google Scholar
- Franck, J.A., Timmermans, A.A.A., Seelen, H.A.M.: Effects of a dynamic hand orthosis for functional use of the impaired upper limb in sub-acute stroke patients: A multiple single case experimental design study. TAD. 25, 177–187 (2013). https://doi.org/10.3233/TAD-130374Article Google Scholar
Source: https://link.springer.com/article/10.1007/s00530-023-01180-0
[WEB] The third thumb: between the prosthesis and the extension – Technology
Posted by Kostas Pantremenos in Assistive Technology, Paretic Hand on October 18, 2023
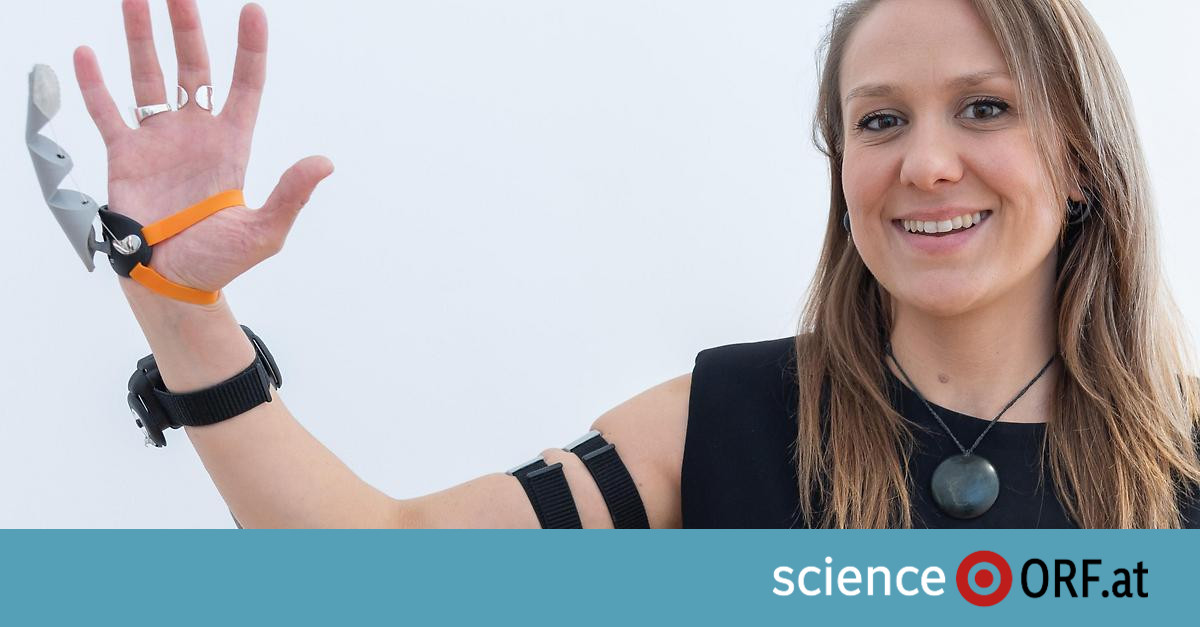
Technology: The third thumb: between the prosthesis and the extension
Victor Booth October 17, 2023 3 min read
This “third thumb” goes to the work of the designer and researcher Danny Claude From the University of Cambridge, one of the conference speakers. The “third thumb” is located just below the little finger and, using two motors, can move in the same way as the normal thumb opposite it.
The system is controlled by pressure sensors placed under the toes. You quickly learn how to deal with this, as British researchers from the Third Thumb Project, among others, did last year. Science fair In London when children and young people tested the prosthetic thumb.
An additional layer of the body
What is actually possible in the world of prosthetics today and what are the far-reaching ideas for linking technical systems with living organisms is the topic of the research meeting entitled, which continues until Wednesday “Convergence?” – roughly translated as “grow together?”
Conference “Convergence?” It is organized by the Academy of Sciences (ÖAW) and the Vienna Science, Research and Technology Fund (WWTF).
One question mark associated with it is whether this is still in the realm of subsidized medical assistance indicated for people with physical disabilities, or whether it is really about targeted enlargement of the human body using technology (“augmentation”).
The “third thumb” in particular can illustrate this: once attached to a patient’s deformed hand, it functions as a kind of prosthetic; On the other hand, it can be considered an extension. For the clade, the latter can be seen as a kind of additional layer to the body – for example, when an exoskeleton helps a worker move heavy objects.
See also Submarines: Australia questions ‘capabilities of French submarines’
Perception and the brain interact quickly
Prosthesis, augmentation or not: The use of technologies with a fairly direct interface to the human body and mind has a negative impact. For example, Claude and her colleague from Cambridge, among others Tamar McCain She found that after just five days of moderate training with the third thumb, the perception of fingers in the test subjects’ brains began to change somewhat.
McCain has already shown in studies that new classes of prosthetics can be formed in the brains of wearers. It is therefore not necessarily related to the control and perception channels of the “hand” or “tool”. McCain stressed that “the brain can find other solutions.”
Despite all the technological considerations, the fundamental key to the sensible and beneficial use of prosthetics is the degree to which the system can be experienced as part of the individual’s body. It’s called research “embodiment”.
Hands are organs of perception
In the future, high-tech prosthetics will be integrated into the skeleton, controlled by redirected neural signals in the muscles and, supported by high-performance algorithms, will also provide feedback to the user – this vision has been described by a research team led by Oscar Assmann. From the Medical University of Vienna more than two years ago In the journal Nature Biomedical Engineering. In the ÖAW ballroom, Assmann explained why fast reactions from the prosthesis to the body are needed.
That’s why you have to learn to understand the “language of nerves” and muscles, says the doctor and technology developer, who and his team have been able to make exciting progress in recent years, for example with new prosthetics for the arm or leg. Straight to the bone.
See also Husqvarna: The Best of Both Worlds
For him, it is clear: the hand is not just an organ that performs movements, but rather a “perception device” that begins in the brain. It is no coincidence that people say they will “look” into the bag and then extend their fingers into it: “My fingers can see,” Azman says. In the coming years, new ideas for direct brain connections, the use of artificial intelligence methods and significant investments by major technology companies will make it clear to what extent these capabilities can be implemented with the help of technology in the future.
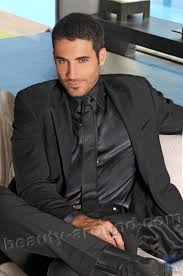
“Certified tv guru. Reader. Professional writer. Avid introvert. Extreme pop culture buff.”