Posts Tagged fMRI
[Abstract] Functional MRI Assessment of Brain Activity During Hand Rehabilitation with an MR-Compatible Soft Glove in Chronic Stroke Patients: A Preliminary Study
Posted by Kostas Pantremenos in Neuroplasticity, Paretic Hand, Radiology/Imaging technology, Rehabilitation robotics on November 17, 2023
Abstract:
Brain plasticity plays a significant role in functional recovery after stroke, but the specific benefits of hand rehabilitation robot therapy remain unclear. Evaluating the specific effects of hand rehabilitation robot therapy is crucial in understanding how it impacts brain activity and its relationship to rehabilitation outcomes. This study aimed to investigate the brain activity pattern during hand rehabilitation exercise using functional magnetic resonance imaging (fMRI), and to compare it before and after 3-week hand rehabilitation robot training. To evaluate it, an fMRI experimental environment was constructed to facilitate the same hand posture used in rehabilitation robot therapy. Two stroke survivors participated and the conjunction analysis results from fMRI scans showed that patient 1 exhibited a significant improvement in activation profile after hand rehabilitation robot training, indicative of improved motor function in the bilateral motor cortex. However, activation profile of patient 2 exhibited a slight decrease, potentially due to habituation to the rehabilitation task. Clinical results supported these findings, with patient 1 experiencing a greater increase in FMA score than patient 2. These results suggest that hand rehabilitation robot therapy can induce different brain activity patterns in stroke survivors, which may be linked to patient-specific training outcomes. Further studies with larger sample sizes are necessary to confirm these findings.
[Abstract + References] The effects of rTMS on motor recovery after stroke: a systematic review of fMRI studies
Posted by Kostas Pantremenos in tDCS/rTMS on November 12, 2023
Abstract
Repetitive transcranial magnetic stimulation (rTMS) has been widely used in motor rehabilitation after stroke, and functional magnetic resonance imaging (fMRI) has been used to investigate the neural mechanisms of motor recovery during stroke therapy. However, there is no review on the mechanism of rTMS intervention for motor recovery after stroke based on fMRI explicitly. We aim to reveal and summarize the neural mechanism of the effects of rTMS on motor function after stroke as measured by fMRI. We carefully performed a literature search using PubMed, EMBASE, Web of Science, and Cochrane Library databases from their respective inceptions to November 2022 to identify any relevant randomized controlled trials. Researchers independently screened the literature, extracted data, and qualitatively described the included studies. Eleven studies with a total of 420 poststroke patients were finally included in this systematic review. A total of 338 of those participants received fMRI examinations before and after rTMS intervention. Five studies reported the effects of rTMS on activation of brain regions, and four studies reported results related to brain functional connectivity (FC). Additionally, five studies analyzed the correlation between fMRI and motor evaluation. The neural mechanism of rTMS in improving motor function after stroke may be the activation and FCs of motor-related brain areas, including enhancement of the activation of motor-related brain areas in the affected hemisphere, inhibition of the activation of motor-related brain areas in the unaffected hemisphere, and changing the FCs of intra-hemispheric and inter-hemispheric motor networks.
This is a preview of subscription content, log in via an institution to check access.
Data availability
Data sharing is not applicable as no datasets were generated or analyzed in this study.
References
- Kuriakose D, Xiao Z (2020) Pathophysiology and treatment of stroke: present status and future perspectives. Int J Mol Sci 21(20). https://doi.org/10.3390/ijms21207609
- Peisker T, Koznar B, Stetkarova I, Widimsky P (2017) Acute stroke therapy: a review. Trends Cardiovasc Med 27(1):59–66. https://doi.org/10.1016/j.tcm.2016.06.009Article PubMed Google Scholar
- Gou X, Xu D, Li F, Hou K, Fang W, Li Y (2021) Pyroptosis in stroke-new insights into disease mechanisms and therapeutic strategies. J Physiol Biochem 77(4):511–529. https://doi.org/10.1007/s13105-021-00817-wArticle CAS PubMed Google Scholar
- Global, regional, and national burden of stroke, 1990–2016: a systematic analysis for the Global Burden of Disease Study 2016 (2019) Global, regional, and national burden of stroke, 1990–2016: a systematic analysis for the Global Burden of Disease Study 2016. Lancet Neurol 18(5):439–458. https://doi.org/10.1016/S1474-4422(19)30034-1Article Google Scholar
- Langhorne P, Coupar F, Pollock A (2009) Motor recovery after stroke: a systematic review. Lancet Neurol 8(8):741–754. https://doi.org/10.1016/S1474-4422(09)70150-4Article PubMed Google Scholar
- Stinear CM (2017) Prediction of motor recovery after stroke: advances in biomarkers. Lancet Neurol 16(10):826–836. https://doi.org/10.1016/S1474-4422(17)30283-1Article PubMed Google Scholar
- Somaa FA, de Graaf TA, Sack AT (2022) Transcranial magnetic stimulation in the treatment of neurological diseases. Front Neurol 13:793253. https://doi.org/10.3389/fneur.2022.793253Article PubMed PubMed Central Google Scholar
- Fisicaro F, Lanza G, Grasso AA, Pennisi G, Bella R, Paulus W, Pennisi M (2019) Repetitive transcranial magnetic stimulation in stroke rehabilitation: review of the current evidence and pitfalls. Ther Adv Neurol Disord 12:1278099885. https://doi.org/10.1177/1756286419878317Article Google Scholar
- Lefaucheur JP, Aleman A, Baeken C, Benninger DH, Brunelin J, Di Lazzaro V, Filipović SR, Grefkes C, Hasan A, Hummel FC, Jääskeläinen SK, Langguth B, Leocani L, Londero A, Nardone R, Nguyen JP, Nyffeler T, Oliveira-Maia AJ, Oliviero A, Padberg F, Palm U, Paulus W, Poulet E, Quartarone A, Rachid F, Rektorová I, Rossi S, Sahlsten H, Schecklmann M, Szekely D, Ziemann U (2020) Evidence-based guidelines on the therapeutic use of repetitive transcranial magnetic stimulation (rTMS): an update (2014–2018). Clin Neurophysiol 131(2):474–528. https://doi.org/10.1016/j.clinph.2019.11.002Article PubMed Google Scholar
- Schambra HM (2018) Repetitive transcranial magnetic stimulation for upper extremity motor recovery: does it help? Curr Neurol Neurosci Rep 18(12):97. https://doi.org/10.1007/s11910-018-0913-8Article PubMed PubMed Central Google Scholar
- Kobayashi M, Pascual-Leone A (2003) Transcranial magnetic stimulation in neurology. Lancet Neurol 2(3):145–156. https://doi.org/10.1016/s1474-4422(03)00321-1Article PubMed Google Scholar
- Gilio F, Conte A, Vanacore N, Frasca V, Inghilleri M, Berardelli A (2007) Excitatory and inhibitory after-effects after repetitive magnetic transcranial stimulation (rTMS) in normal subjects. Exp Brain Res 176(4):588–593. https://doi.org/10.1007/s00221-006-0638-9Article CAS PubMed Google Scholar
- Tang Z, Han K, Wang R, Zhang Y, Zhang H (2022) Excitatory repetitive transcranial magnetic stimulation over the ipsilesional hemisphere for upper limb motor function after stroke: a systematic review and meta-analysis. Front Neurol 13:918597. https://doi.org/10.3389/fneur.2022.918597Article PubMed PubMed Central Google Scholar
- Bai Z, Zhang J, Fong K (2022) Effects of transcranial magnetic stimulation in modulating cortical excitability in patients with stroke: a systematic review and meta-analysis. J Neuroeng Rehabil 19(1):24. https://doi.org/10.1186/s12984-022-00999-4Article PubMed PubMed Central Google Scholar
- Gutiérrez-Muto AM, Castilla J, Freire M, Oliviero A, Tornero J (2020) Theta burst stimulation: technical aspects about TMS devices. Brain Stimul 13(3):562–564. https://doi.org/10.1016/j.brs.2020.01.002Article PubMed Google Scholar
- Suppa A, Huang YZ, Funke K, Ridding MC, Cheeran B, Di Lazzaro V, Ziemann U, Rothwell JC (2016) Ten years of theta burst stimulation in humans: established knowledge, unknowns and prospects. Brain Stimul 9(3):323–335. https://doi.org/10.1016/j.brs.2016.01.006Article CAS PubMed Google Scholar
- Huang YZ, Edwards MJ, Rounis E, Bhatia KP, Rothwell JC (2005) Theta burst stimulation of the human motor cortex. Neuron 45(2):201–206. https://doi.org/10.1016/j.neuron.2004.12.033Article CAS PubMed Google Scholar
- Di Pino G, Pellegrino G, Assenza G, Capone F, Ferreri F, Formica D, Ranieri F, Tombini M, Ziemann U, Rothwell JC, Di Lazzaro V (2014) Modulation of brain plasticity in stroke: a novel model for neurorehabilitation. Nat Rev Neurol 10(10):597–608. https://doi.org/10.1038/nrneurol.2014.162Article PubMed Google Scholar
- Simonetta-Moreau M (2014) Non-invasive brain stimulation (NIBS) and motor recovery after stroke. Ann Phys Rehabil Med 57(8):530–542. https://doi.org/10.1016/j.rehab.2014.08.003Article CAS PubMed Google Scholar
- Stefan K, Kunesch E, Cohen LG, Benecke R, Classen J (2000) Induction of plasticity in the human motor cortex by paired associative stimulation. Brain 123(Pt 3):572–584. https://doi.org/10.1093/brain/123.3.572Article PubMed Google Scholar
- Guidali G, Roncoroni C, Bolognini N (2021) Modulating frontal networks’ timing-dependent-like plasticity with paired associative stimulation protocols: recent advances and future perspectives. Front Hum Neurosci 15:658723. https://doi.org/10.3389/fnhum.2021.658723Article PubMed PubMed Central Google Scholar
- Stefan K, Kunesch E, Benecke R, Cohen LG, Classen J (2002) Mechanisms of enhancement of human motor cortex excitability induced by interventional paired associative stimulation. J Physiol 543(Pt 2):699–708. https://doi.org/10.1113/jphysiol.2002.023317Article CAS PubMed PubMed Central Google Scholar
- Hu Y, Guo TC, Zhang XY, Tian J, Lu YS (2019) Paired associative stimulation improves synaptic plasticity and functional outcomes after cerebral ischemia. Neural Regen Res 14(11):1968–1976. https://doi.org/10.4103/1673-5374.259618Article CAS PubMed PubMed Central Google Scholar
- Carson RG, Kennedy NC (2013) Modulation of human corticospinal excitability by paired associative stimulation. Front Hum Neurosci 7:823. https://doi.org/10.3389/fnhum.2013.00823Article PubMed PubMed Central Google Scholar
- Du J, Tian L, Liu W, Hu J, Xu G, Ma M, Fan X, Ye R, Jiang Y, Yin Q, Zhu W, Xiong Y, Yang F, Liu X (2016) Effects of repetitive transcranial magnetic stimulation on motor recovery and motor cortex excitability in patients with stroke: a randomized controlled trial. Eur J Neurol 23(11):1666–1672. https://doi.org/10.1111/ene.13105Article CAS PubMed Google Scholar
- Moslemi HF, Kordi YA, Razeghi M, Shariat A, Bagheri Z, Rezaei K (2021) The effect of high-frequency repetitive transcranial magnetic stimulation on functional indices of affected upper limb in patients with subacute stroke. J Biomed Phys Eng 11(2):175–184. https://doi.org/10.31661/jbpe.v0i0.879Article Google Scholar
- Luk KY, Ouyang HX, Pang M (2022) Low-frequency rTMS over contralesional M1 increases ipsilesional cortical excitability and motor function with decreased interhemispheric asymmetry in subacute stroke: a randomized controlled study. Neural Plast 2022:3815357. https://doi.org/10.1155/2022/3815357Article PubMed PubMed Central Google Scholar
- Avenanti A, Coccia M, Ladavas E, Provinciali L, Ceravolo MG (2012) Low-frequency rTMS promotes use-dependent motor plasticity in chronic stroke: a randomized trial. Neurology 78(4):256–264. https://doi.org/10.1212/WNL.0b013e3182436558Article CAS PubMed Google Scholar
- Guo Z, Jin Y, Bai X, Jiang B, He L, McClure MA, Mu Q (2021) Distinction of high- and low-frequency repetitive transcranial magnetic stimulation on the functional reorganization of the motor network in stroke patients. Neural Plast 2021:8873221. https://doi.org/10.1155/2021/8873221Article PubMed PubMed Central Google Scholar
- Zhang JJ, Bai Z, Fong K (2022) Priming intermittent theta burst stimulation for hemiparetic upper limb after stroke: a randomized controlled trial. Stroke 53(7):2171–2181. https://doi.org/10.1161/STROKEAHA.121.037870Article PubMed Google Scholar
- Park CH, Chang WH, Ohn SH, Kim ST, Bang OY, Pascual-Leone A, Kim YH (2011) Longitudinal changes of resting-state functional connectivity during motor recovery after stroke. Stroke 42(5):1357–1362. https://doi.org/10.1161/STROKEAHA.110.596155Article PubMed PubMed Central Google Scholar
- Golestani AM, Tymchuk S, Demchuk A, Goodyear BG (2013) Longitudinal evaluation of resting-state FMRI after acute stroke with hemiparesis. Neurorehabil Neural Repair 27(2):153–163. https://doi.org/10.1177/1545968312457827Article PubMed Google Scholar
- Lam TK, Dawson DR, Honjo K, Ross B, Binns MA, Stuss DT, Black SE, Chen JJ, Levine BT, Fujioka T, Chen JL (2018) Neural coupling between contralesional motor and frontoparietal networks correlates with motor ability in individuals with chronic stroke. J Neurol Sci 384:21–29. https://doi.org/10.1016/j.jns.2017.11.007Article PubMed Google Scholar
- Peng Y, Liu J, Hua M, Liang M, Yu C (2019) Enhanced effective connectivity from ipsilesional to contralesional M1 in well-recovered subcortical stroke patients. Front Neurol 10:909. https://doi.org/10.3389/fneur.2019.00909Article PubMed PubMed Central Google Scholar
- Frahm J, Bruhn H, Merboldt KD, Hänicke W (1992) Dynamic MR imaging of human brain oxygenation during rest and photic stimulation. J Magn Reson Imaging 2(5):501–505. https://doi.org/10.1002/jmri.1880020505Article CAS PubMed Google Scholar
- Kwong KK, Belliveau JW, Chesler DA, Goldberg IE, Weisskoff RM, Poncelet BP, Kennedy DN, Hoppel BE, Cohen MS, Turner R, Et A (1992) Dynamic magnetic resonance imaging of human brain activity during primary sensory stimulation. Proc Natl Acad Sci USA 89(12):5675–5679. https://doi.org/10.1073/pnas.89.12.5675Article CAS PubMed PubMed Central Google Scholar
- Turner R, Howseman A, Rees GE, Josephs O, Friston K (1998) Functional magnetic resonance imaging of the human brain: data acquisition and analysis. Exp Brain Res 123(1–2):5–12. https://doi.org/10.1007/s002210050538Article CAS PubMed Google Scholar
- Li B, Deng S, Sang B, Zhu W, Zhuo B, Zhang M, Qin C, Lyu Y, Du Y, Meng Z (2022) Revealing the neuroimaging mechanism of acupuncture for poststroke aphasia: a systematic review. Neural Plast 2022:5635596. https://doi.org/10.1155/2022/5635596Article PubMed PubMed Central Google Scholar
- Fox MD, Raichle ME (2007) Spontaneous fluctuations in brain activity observed with functional magnetic resonance imaging. Nat Rev Neurosci 8(9):700–711. https://doi.org/10.1038/nrn2201Article CAS PubMed Google Scholar
- Moher D, Liberati A, Tetzlaff J, Altman DG (2009) Preferred reporting items for systematic reviews and meta-analyses: the PRISMA statement. Plos Med 6(7):e1000097. https://doi.org/10.1371/journal.pmed.1000097Article PubMed PubMed Central Google Scholar
- Page MJ, McKenzie JE, Bossuyt PM, Boutron I, Hoffmann TC, Mulrow CD, Shamseer L, Tetzlaff JM, Akl EA, Brennan SE, Chou R, Glanville J, Grimshaw JM, Hróbjartsson A, Lalu MM, Li T, Loder EW, Mayo-Wilson E, McDonald S, McGuinness LA, Stewart LA, Thomas J, Tricco AC, Welch VA, Whiting P, Moher D (2021) The PRISMA 2020 statement: an updated guideline for reporting systematic reviews. BMJ 372:n71. https://doi.org/10.1136/bmj.n71Article PubMed PubMed Central Google Scholar
- Maher CG, Sherrington C, Herbert RD, Moseley AM, Elkins M (2003) Reliability of the PEDro scale for rating quality of randomized controlled trials. Phys Ther 83(8):713–721Article PubMed Google Scholar
- de Morton NA (2009) The PEDro scale is a valid measure of the methodological quality of clinical trials: a demographic study. Aust J Physiother 55(2):129–133. https://doi.org/10.1016/s0004-9514(09)70043-1Article PubMed Google Scholar
- Ameli M, Grefkes C, Kemper F, Riegg FP, Rehme AK, Karbe H, Fink GR, Nowak DA (2009) Differential effects of high-frequency repetitive transcranial magnetic stimulation over ipsilesional primary motor cortex in cortical and subcortical middle cerebral artery stroke. Ann Neurol 66(3):298–309. https://doi.org/10.1002/ana.21725Article PubMed Google Scholar
- Chang WH, Kim YH, Yoo WK, Goo KH, Park CH, Kim ST, Pascual-Leone A (2012) rTMS with motor training modulates cortico-basal ganglia-thalamocortical circuits in stroke patients. Restor Neurol Neurosci 30(3):179–189. https://doi.org/10.3233/RNN-2012-110162Article PubMed PubMed Central Google Scholar
- Chen Q, Shen W, Sun H, Zhang H, Liu C, Chen Z, Yu L, Cai X, Ke J, Li L, Zhang L, Fang Q (2022) The effect of coupled inhibitory-facilitatory repetitive transcranial magnetic stimulation on shaping early reorganization of the motor network after stroke. Brain Res 1790:147959. https://doi.org/10.1016/j.brainres.2022.147959Article CAS PubMed Google Scholar
- Du J, Yang F, Hu J, Hu J, Xu Q, Cong N, Zhang Q, Liu L, Mantini D, Zhang Z, Lu G, Liu X (2019) Effects of high- and low-frequency repetitive transcranial magnetic stimulation on motor recovery in early stroke patients: evidence from a randomized controlled trial with clinical, neurophysiological and functional imaging assessments. Neuroimage Clin 21:101620. https://doi.org/10.1016/j.nicl.2018.101620Article PubMed Google Scholar
- Juan D, Yao W, Li J, Yang F, Hu J, Xu Q, Liu L, Lv Q, Liu R, Ye R, Ma M, Zhu W, Zhang Z, Liu X (2022) Motor network reorganization after repetitive transcranial magnetic stimulation in early stroke patients: a resting state fMRI study. Neurorehabil Neural Repair 36(1):61–68. https://doi.org/10.1177/15459683211054184Article Google Scholar
- Gottlieb A, Boltzmann M, Schmidt SB, Gutenbrunner C, Krauss JK, Stangel M, Höglinger GU, Wallesch CW, Rollnik JD (2021) Treatment of upper limb spasticity with inhibitory repetitive transcranial magnetic stimulation: a randomized placebo-controlled trial. NeuroRehabilitation 49(3):425–434. https://doi.org/10.3233/NRE-210088Article PubMed Google Scholar
- Li J, Zhang XW, Zuo ZT, Lu J, Meng CL, Fang HY, Xue R, Fan Y, Guan YZ, Zhang WH (2016) Cerebral functional reorganization in ischemic stroke after repetitive transcranial magnetic stimulation: an fMRI study. Cns Neurosci Ther 22(12):952–960. https://doi.org/10.1111/cns.12593Article CAS PubMed PubMed Central Google Scholar
- Nowak DA, Grefkes C, Dafotakis M, Eickhoff S, Küst J, Karbe H, Fink GR (2008) Effects of low-frequency repetitive transcranial magnetic stimulation of the contralesional primary motor cortex on movement kinematics and neural activity in subcortical stroke. Arch Neurol 65(6):741–747. https://doi.org/10.1001/archneur.65.6.741Article PubMed Google Scholar
- Qin Y, Liu X, Guo X, Liu M, Li H, Xu S (2021) Low-frequency repetitive transcranial magnetic stimulation restores dynamic functional connectivity in subcortical stroke. Front Neurol 12:771034. https://doi.org/10.3389/fneur.2021.771034Article PubMed PubMed Central Google Scholar
- Allen EA, Damaraju E, Plis SM, Erhardt EB, Eichele T, Calhoun VD (2014) Tracking whole-brain connectivity dynamics in the resting state. Cereb Cortex 24:663–676. https://doi.org/10.1093/cercor/bhs352Article PubMed Google Scholar
- Dekhil O, Shalaby A, Soliman A, Mahmoud A, Kong M, Barnes G, Elmaghraby A, El-Baz A (2021) Identifying brain areas correlated with ADOS raw scores by studying altered dynamic functional connectivity patterns. Med Image Anal 68:101899. https://doi.org/10.1016/j.media.2020.101899Article PubMed Google Scholar
- Rosso C, Moulton EJ, Kemlin C, Leder S, Corvol JC, Mehdi S, Obadia MA, Obadia M, Yger M, Meseguer E, Perlbarg V, Valabregue R, Magno S, Lindberg P, Meunier S, Lamy JC (2022) Cerebello-motor paired associative stimulation and motor recovery in stroke: a randomized, sham-controlled, double-blind pilot trial. Neurotherapeutics 19(2):491–500. https://doi.org/10.1007/s13311-022-01205-yArticle PubMed PubMed Central Google Scholar
- Tosun A, Türe S, Askin A, Yardimci EU, Demirdal SU, Kurt IT, Tosun O, Kocyigit H, Akhan G, Gelal FM (2017) Effects of low-frequency repetitive transcranial magnetic stimulation and neuromuscular electrical stimulation on upper extremity motor recovery in the early period after stroke: a preliminary study. Top Stroke Rehabil 24(5):361–367. https://doi.org/10.1080/10749357.2017.1305644Article PubMed Google Scholar
- Zhang L, Xing G, Fan Y, Guo Z, Chen H, Mu Q (2017) Short- and long-term effects of repetitive transcranial magnetic stimulation on upper limb motor function after stroke: a systematic review and meta-analysis. Clin Rehabil 31(9):1137–1153. https://doi.org/10.1177/0269215517692386Article PubMed Google Scholar
- Xiang H, Sun J, Tang X, Zeng K, Wu X (2019) The effect and optimal parameters of repetitive transcranial magnetic stimulation on motor recovery in stroke patients: a systematic review and meta-analysis of randomized controlled trials. Clin Rehabil 33(5):847–864. https://doi.org/10.1177/0269215519829897Article PubMed Google Scholar
- Fan H, Song Y, Cen X, Yu P, Bíró I, Gu Y (2021) The effect of repetitive transcranial magnetic stimulation on lower-limb motor ability in stroke patients: a systematic review. Front Hum Neurosci 15:620573. https://doi.org/10.3389/fnhum.2021.620573Article PubMed PubMed Central Google Scholar
- Gao B, Wang Y, Zhang D, Wang Z, Wang Z (2022) Intermittent theta-burst stimulation with physical exercise improves poststroke motor function: a systemic review and meta-analysis. Front Neurol 13:964627. https://doi.org/10.3389/fneur.2022.964627Article PubMed PubMed Central Google Scholar
- Liepert J, Hamzei F, Weiller C (2000) Motor cortex disinhibition of the unaffected hemisphere after acute stroke. Muscle Nerve 23(11):1761–1763. https://doi.org/10.1002/1097-4598(200011)23:11%3c1761::aid-mus14%3e3.0.co;2-mArticle CAS PubMed Google Scholar
- Shimizu T, Hosaki A, Hino T, Sato M, Komori T, Hirai S, Rossini PM (2002) Motor cortical disinhibition in the unaffected hemisphere after unilateral cortical stroke. Brain 125(Pt 8):1896–1907. https://doi.org/10.1093/brain/awf183Article PubMed Google Scholar
- Cicinelli P, Pasqualetti P, Zaccagnini M, Traversa R, Oliveri M, Rossini PM (2003) Interhemispheric asymmetries of motor cortex excitability in the postacute stroke stage: a paired-pulse transcranial magnetic stimulation study. Stroke 34(11):2653–2658. https://doi.org/10.1161/01.STR.0000092122.96722.72Article PubMed Google Scholar
- Murase N, Duque J, Mazzocchio R, Cohen LG (2004) Influence of interhemispheric interactions on motor function in chronic stroke. Ann Neurol 55(3):400–409. https://doi.org/10.1002/ana.10848Article PubMed Google Scholar
- Koski L, Mernar TJ, Dobkin BH (2004) Immediate and long-term changes in corticomotor output in response to rehabilitation: correlation with functional improvements in chronic stroke. Neurorehabil Neural Repair 18(4):230–249. https://doi.org/10.1177/1545968304269210Article PubMed Google Scholar
- Lin YL, Potter-Baker KA, Cunningham DA, Li M, Sankarasubramanian V, Lee J, Jones S, Sakaie K, Wang X, Machado AG, Plow EB (2020) Stratifying chronic stroke patients based on the influence of contralesional motor cortices: an inter-hemispheric inhibition study. Clin Neurophysiol 131(10):2516–2525. https://doi.org/10.1016/j.clinph.2020.06.016Article PubMed PubMed Central Google Scholar
- Bocci T, Pietrasanta M, Cerri C, Restani L, Caleo M, Sartucci F (2014) Visual callosal connections: role in visual processing in health and disease. Rev Neurosci 25(1):113–127. https://doi.org/10.1515/revneuro-2013-0025Article PubMed Google Scholar
- Bocci T, Nasini F, Caleo M, Restani L, Barloscio D, Ardolino G, Priori A, Maffei L, Nardi M, Sartucci F (2018) Unilateral application of cathodal tDCS reduces transcallosal inhibition and improves visual acuity in amblyopic patients. Front Behav Neurosci 12:109. https://doi.org/10.3389/fnbeh.2018.00109Article PubMed PubMed Central Google Scholar
- Wang Q, Zhang D, Zhao Y, Hai H, Ma Y (2020) Effects of high-frequency repetitive transcranial magnetic stimulation over the contralesional motor cortex on motor recovery in severe hemiplegic stroke: a randomized clinical trial. Brain Stimul 13:979–986. https://doi.org/10.1016/j.brs.2020.03.020Article PubMed Google Scholar
- Grefkes C, Fink GR (2012) Disruption of motor network connectivity post-stroke and its noninvasive neuromodulation. Curr Opin Neurol 25(6):670–675. https://doi.org/10.1097/WCO.0b013e3283598473Article PubMed Google Scholar
- Paul T, Hensel L, Rehme AK, Tscherpel C, Eickhoff SB, Fink GR, Grefkes C, Volz LJ (2021) Early motor network connectivity after stroke: an interplay of general reorganization and state-specific compensation. Hum Brain Mapp 42(16):5230–5243. https://doi.org/10.1002/hbm.25612Article PubMed PubMed Central Google Scholar
- Olafson ER, Jamison KW, Sweeney EM, Liu H, Wang D, Bruss JE, Boes AD, Kuceyeski A (2021) Functional connectome reorganization relates to post-stroke motor recovery and structural and functional disconnection. Neuroimage 245:118642. https://doi.org/10.1016/j.neuroimage.2021.118642Article PubMed Google Scholar
- Hartwigsen G, Volz LJ (2021) Probing rapid network reorganization of motor and language functions via neuromodulation and neuroimaging. Neuroimage 224:117449. https://doi.org/10.1016/j.neuroimage.2020.117449Article PubMed Google Scholar
- Binder E, Leimbach M, Pool EM, Volz LJ, Eickhoff SB, Fink GR, Grefkes C (2021) Cortical reorganization after motor stroke: a pilot study on differences between the upper and lower limbs. Hum Brain Mapp 42(4):1013–1033. https://doi.org/10.1002/hbm.25275Article PubMed Google Scholar
- Rogers BP, Morgan VL, Newton AT, Gore JC (2007) Assessing functional connectivity in the human brain by fMRI. Magn Reson Imaging 25(10):1347–1357. https://doi.org/10.1016/j.mri.2007.03.007Article PubMed PubMed Central Google Scholar
- van den Heuvel MP, Hulshoff PH (2010) Exploring the brain network: a review on resting-state fMRI functional connectivity. Eur Neuropsychopharmacol 20(8):519–534. https://doi.org/10.1016/j.euroneuro.2010.03.008Article CAS PubMed Google Scholar
- Desowska A, Turner DL (2019) Dynamics of brain connectivity after stroke. Rev Neurosci 30(6):605–623. https://doi.org/10.1515/revneuro-2018-0082Article PubMed Google Scholar
- Xia Y, Huang G, Quan X, Qin Q, Li H, Xu C, Liang Z (2021) Dynamic structural and functional reorganizations following motor stroke. Med Sci Monit 27:e929092. https://doi.org/10.12659/MSM.929092Article PubMed PubMed Central Google Scholar
- Volz LJ, Rehme AK, Michely J, Nettekoven C, Eickhoff SB, Fink GR, Grefkes C (2016) Shaping early reorganization of neural networks promotes motor function after stroke. Cereb Cortex 26(6):2882–2894. https://doi.org/10.1093/cercor/bhw034Article CAS PubMed PubMed Central Google Scholar
- Li S, Francisco GE, Rymer WZ (2021) A new definition of poststroke spasticity and the interference of spasticity with motor recovery from acute to chronic stages. Neurorehabil Neural Repair 35(7):601–610. https://doi.org/10.1177/15459683211011214Article PubMed Google Scholar
[ARTICLE] The Role of Baseline Functional MRI as a Predictor of Post-Stroke Rehabilitation Efficacy in Patients with Moderate to Severe Upper Extremity Dysfunction – Full Text
Posted by Kostas Pantremenos in Paretic Hand, Radiology/Imaging technology on February 4, 2023
Abstract
Introduction: Upper extremity impairment is one of the common complications following a stroke. There are numerous rehabilitation strategies to address this problem. However, patients with moderate to severe upper limb disabilities respond differently to the same rehabilitation protocol. Apart from each patient’s unique characteristics, there are specific brain reorganizing patterns that affect the post-rehabilitation response rate. Functional magnetic resonance imaging (fMRI) determines brain activation area and connectivity patterns and has been utilized in the neurorehabilitation field.
Material and Methods: Six stroke patients who suffered from moderate to severe upper extremity dysfunction were enrolled in this pilot study. Upper extremity function tests including the Fugl-Meyer assessment test for upper extremity (FMA-UE), and Wolf Motor Function Test (WMFT) were utilized before and after completing an intensive rehabilitation. The intensive rehabilitation program was conducted one hour a day for five days per week for four weeks. Moreover, fMRI was applied before initiating rehabilitation. The regions of interest were those associated with movement, including Brodmann areas (BA) BA1-BA6.
Results: Six stroke patients in the sub-acute to chronic phase and ages ranging between 33 – 75 years were enrolled. All patients showed an improvement in upper limb function after four weeks of rehabilitation. Patient number one (Pt1) had the most improvement in FMA-UE, while patient number four (Pt4) recovered the most measured by WMFT. Pt1 demonstrated increased activity in all contralesional regions, whereas Pt4 had only increased activity in ipsilesional areas. Furthermore, patients with greater activation in the ipsilesional BA6 (Pt1, Pt4, Pt5, and Pt6) had better responses to the rehabilitation therapy.
Conclusion: Patients with greater activation in the baseline fMRI, particularly ipsilesional BA6, had a better response to the intensive rehabilitation therapy. However, the patients with the most severe hand dysfunction showed lesser improvement despite the same brain activity as others in the initial fMRI.
1. Introduction
Stroke is one of the most important causes of disability worldwide. The incidence and mortality of stroke are increasing due to the growing elderly population [1] [2]. Contralateral upper limb hemiparesis is the most prevalent impairment following a stroke, affecting more than 80% of acute stroke patients and more than 40% of chronic ones. Upper-extremity motor paresis may be accompanied by additional neurological symptoms that impede motor function recovery, necessitating targeted rehabilitation therapy [3].
The primary goal of post-stroke management is to re-establish daily activities through different rehabilitation methods despite the residual impairments [4]. Neurological recovery follows a nonlinear, logarithmic pattern, meaning that most recovery is expected in the first three months after a stroke. Furthermore, recovery from a stroke can be characterized as an improvement in several outcomes, starting with biological and neurologic changes that appear to improve performance and activity-based behavioral measures. Adaptation, regeneration, and neuroplasticity are the three fundamental mechanisms in brain recovery following a stroke. Adaptation happens by using alternate physical movements or equipment to compensate for functional loss. Regeneration is known as the growth of damaged neural cells into new tissue to restore function. The main recovery mechanism is neuroplasticity, characterized by alterations or rewiring within the neural network [3] [4] [5].
Functional disabilities after a stroke affect the patient’s quality of life and leave a significant financial burden on society [6]. In addition, only a few patients with severe hand dysfunction will show promising results after the rehabilitation program [7]. However, it is relatively difficult to predict the recovery outcome and success rate only based on the clinical data in severely disabled patients. Thus, a prognostic tool to determine long-term motor recovery in terms of rehabilitation efficacy costs many expenses. Previous clinical studies have suggested the effectiveness of intensive motor training paired with repetitive transcranial magnetic stimulation (rTMS), muscular electrical stimulation, brain-computer interfaces, and robotic devices in upper limb rehabilitation in stroke patients [8] [9].
Notably, stroke patients are more likely to have permanent functional deficits because the non-activated brain has a limited neuroplasticity capacity. Neuroplasticity is also affected by the type of intervention, the patient’s age, the location of the lesion, and handedness. Therefore, tools like functional magnetic resonance imaging (fMRI) are utilized to investigate brain activity and the influence of neurorehabilitation methods on neuroplasticity in addition to clinical disability assessment tools. FMRI has an excellent spatial resolution, allowing researchers to gain insight into the brain reorganization process and its associated functional recovery after stroke neurorehabilitation [10]. In general, fMRI captures the blood oxygen level-dependent (BOLD) signal is captured using T2*-weighted imaging. Higher neuron activity is associated with higher blood flow in the related areas, increasing the oxyhemoglobin-to ratio and the signal [11]. Task performance during fMRI causes hemodynamic response function (HRF) in the motor cortex area, which changes the BOLD signal recorded by the MRI machine.
We conducted this study using the fMRI as a prognostic tool for upper limb functional recovery after intensive rehabilitation from a stroke. Although these findings should not exclude the most severe patients from rehabilitation therapy, but also help to define the realistic rehab consequences for both therapist and patients.[…]


[ARTICLE] Clinical Effectiveness of Non-Immersive Virtual Reality Tasks for Post-Stroke Neuro-Rehabilitation of Distal Upper-Extremities: A Case Report – Full Text
Posted by Kostas Pantremenos in Paretic Hand, Virtual reality rehabilitation on December 29, 2022
Abstract
A library of non-immersive Virtual Reality (VR) tasks were developed for post-stroke rehabilitation of distal upper extremities. The objective was to evaluate the rehabilitation impact of the developed VR-tasks on a patient with chronic stroke. The study involved a 50-year-old male patient with chronic (13 month) stroke. Twenty VR therapy sessions of 45 min each were given. Clinical scales, cortical-excitability measures, functional MRI (fMRI), and diffusion tensor imaging (DTI) data were acquired pre-and post-therapy to evaluate the motor recovery. Increase in Fugl-Meyer Assessment (wrist/hand) by 2 units, Barthel Index by 5 units, Brunnstrom Stage by 1 unit, Addenbrooke’s Cognitive Examination by 3 units, Wrist Active Range of Motion by 5° and decrease in Modified Ashworth Scale by 1 unit were observed. Ipsilesional Motor Evoked Potential (MEP) amplitude (obtained using Transcranial Magnetic Stimulation) was increased by 60.9µV with a decrease in Resting Motor Threshold (RMT) by 7%, and contralesional MEP amplitude was increased by 56.2µV with a decrease in RMT by 7%. The fMRI-derived Laterality Index of Sensorimotor Cortex increased in precentral-gyrus (from 0.28 to 0.33) and in postcentral-gyrus (from 0.07 to 0.3). The DTI-derived FA-asymmetry decreased in precentral-gyrus (from 0.029 to 0.024) and in postcentral-gyrus (from 0.027 to 0.017). Relative reduction in task-specific performance metrics, i.e., time taken to complete the task (31.6%), smoothness of trajectory (76.7%), and relative percentage error (80.7%), were observed from day 1 to day 20 of the VR therapy. VR therapy resulted in improvement in clinical outcomes in a patient with chronic stroke. The research also gives insights to further improve the overall system of rehabilitation.
1. Introduction
Residual upper-arm disabilities are common morbidities in the chronic phase of recovery, affecting more than 66% of patients with stroke [1]. The literature suggests the requirement of intensive practice to facilitate functional recovery in the chronic phase [2]. However, factors such as lack of objective assessment, associated clinical burden and boredom highly limit the effectiveness of traditional rehabilitation [3]. In recent years, Virtual Reality (VR)-assisted rehabilitation has emerged as a supplementary approach to address some of the limitations associated with traditional physiotherapy [4,5]. Post-stroke rehabilitation of distal upper extremities is comparatively slower and requires intensive targeted practice to conduct Activities of Daily Living (ADL) [6]. However, limited literature exists to support targeted VR-based rehabilitation of distal upper extremities [7]. Furthermore, the exact neurophysiological aspects showing enhanced post-stroke recovery at the neuronal level are still unclear [8]. In our previous study, a library of VR tasks specific to distal-joint rehabilitation was developed, and task-specific outcome metrics were validated with forty healthy subjects and two patients with stroke [9]. In this study, we present the case of a 50-year-old male patient with 13-month chronic stroke who participated in VR therapy and the associated functional and neuronal changes observed in response to the therapy. The primary objectives of this study were to evaluate the efficacy of the developed VR tasks and to investigate the neurophysiological behavior supporting post-stroke motor recovery in response to the VR therapy during the chronic phase.
2. Case Description
The study was approved by the Institutional Review Board (IRB) at All India Institute of Medical Science (AIIMS), New Delhi, India, under protocol-number IEC-229/11.4.2020. The patient provided written informed consent before enrollment in this study.
2.1. Subject
The patient was a 50-year-old right-handed male (henceforth, referred as “P”); he was well educated and was a lawyer by profession. He had an incidence of right thalamo-gangliocapsular-bleed in October 2020 (Supplement Figure S1). Additional clinical-presentation details are provided in the Supplementary Materials (Section S1). He had no history of substance abuse, such as consuming tobacco or alcohol, but experienced hypertension in the past 10 years and diabetes for past 1 year. After onset of stroke, he was admitted to a local hospital and discharged after 14 days for rehabilitation management at home. He underwent supervised home-based physiotherapy after 3 months after stroke-onset (see Supplementary Materials). Assessment scores at enrollment are given in Figure 1.
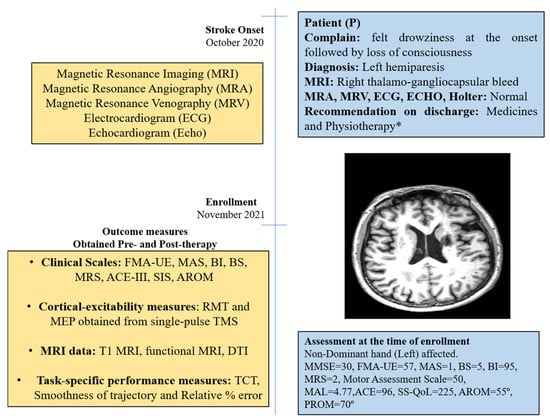
[WEB] Investigating Brain Activity After Acquired and Traumatic Brain Injury: Applications of Functional MRI
Posted by Kostas Pantremenos in Radiology/Imaging technology, TBI on December 3, 2022
About this Research Topic

Every year, the lives of millions of people are affected by traumatic (TBI) or acquired brain injury (ABI). The search for treatments and interventions that aid in TBI and ABI after-effects should be the priority in modern clinical research. In recent decades there has been great progress in functional neuroimaging techniques and analysis methods. However, a disconnect between functional neuroimaging findings and their application to clinical settings remains. Examining the mechanism of brain injury can greatly advance our knowledge of the consequences and functional outcomes of ABI and TBI. Further, it can help characterize the nature of cognitive deficits after brain injury. More importantly, understanding how the functioning of the brain changes after ABI and TBI has the potential to promote development of targeted and effective interventions.
There are methodological barriers that might explain why functional neuroimaging has not received sufficient attention in these patient groups.
Functional neuroimaging in brain injury presents substantial challenges to researchers. Both patient populations are diverse in terms of brain anatomy and lesion locations. Selecting the appropriate control group and control condition can be challenging. The most significant challenge is accurate measurement and interpretation of signal in the damaged brain, because it is not well known how the blood flow to non-damaged areas is affected by the lesion. The main goal in this area of study is to disentangle blood flow effects due to meaningful brain activity and those due to lesion. Alternative methods of imaging, such as perfusion weighted imaging using ASL (arterial spin labeling) are only now beginning to be explored.
Despite the above challenges, functional neuroimaging is still a fruitful and promising area of investigation in TBI and ABI with substantial knowledge and insight into the mechanisms of brain injury remaining to be gained. The ultimate goals of functional neuroimaging in TBI and ABI are in line with the need for solution-oriented research in today’s society. This under-studied area bridges human neuroscience and neurology.
In this Research Topic, we present a collection of original research and review articles focused on functional neuroimaging in TBI and ABI, including task-based functional Magnetic Resonance Imaging (fMRI), resting state MRI, ASL, PET, etc. The assembled articles address
1) how the challenges of working with lesioned brain can be overcome,
2) how findings on cognitive function after ABI and TBI can be linked with brain mechanisms, and
3) how findings about brain mechanisms can be applied to improving quality of care and rehabilitation strategies.
[WEB] What are the Clinical Applications of Functional Neuroimaging?
Posted by Kostas Pantremenos in Radiology/Imaging technology on October 28, 2022
By Vasco Medeiros, BSc Reviewed by Aimee Molineux
Introduction
Functional magnetic resonance imaging (fMRI)
Functional neuroimaging in the monitoring of sleep patterns
Using positron emission tomography (PET) to treat lymphoma
References
Further reading
Most people would categorize computerized tomography (CAT) scans, positron emission tomography (PET) scans, and magnetic resonance imaging (MRI) scans into the same grouping. However, it is important to note that significant variability exists between these neuroimaging techniques. Often separated into two sets, there exists structural neuroimaging and functional neuroimaging.
While structural neuroimaging is used to segregate and assay different brain regions, like the shape of grey matter, cerebrospinal fluid, et cetera., functional neuroimaging incorporates the measure of neural activity. This assay of neural activity, measured through electrodes, magnetic and radio waves, and X-Rays, is used to assess mental function.
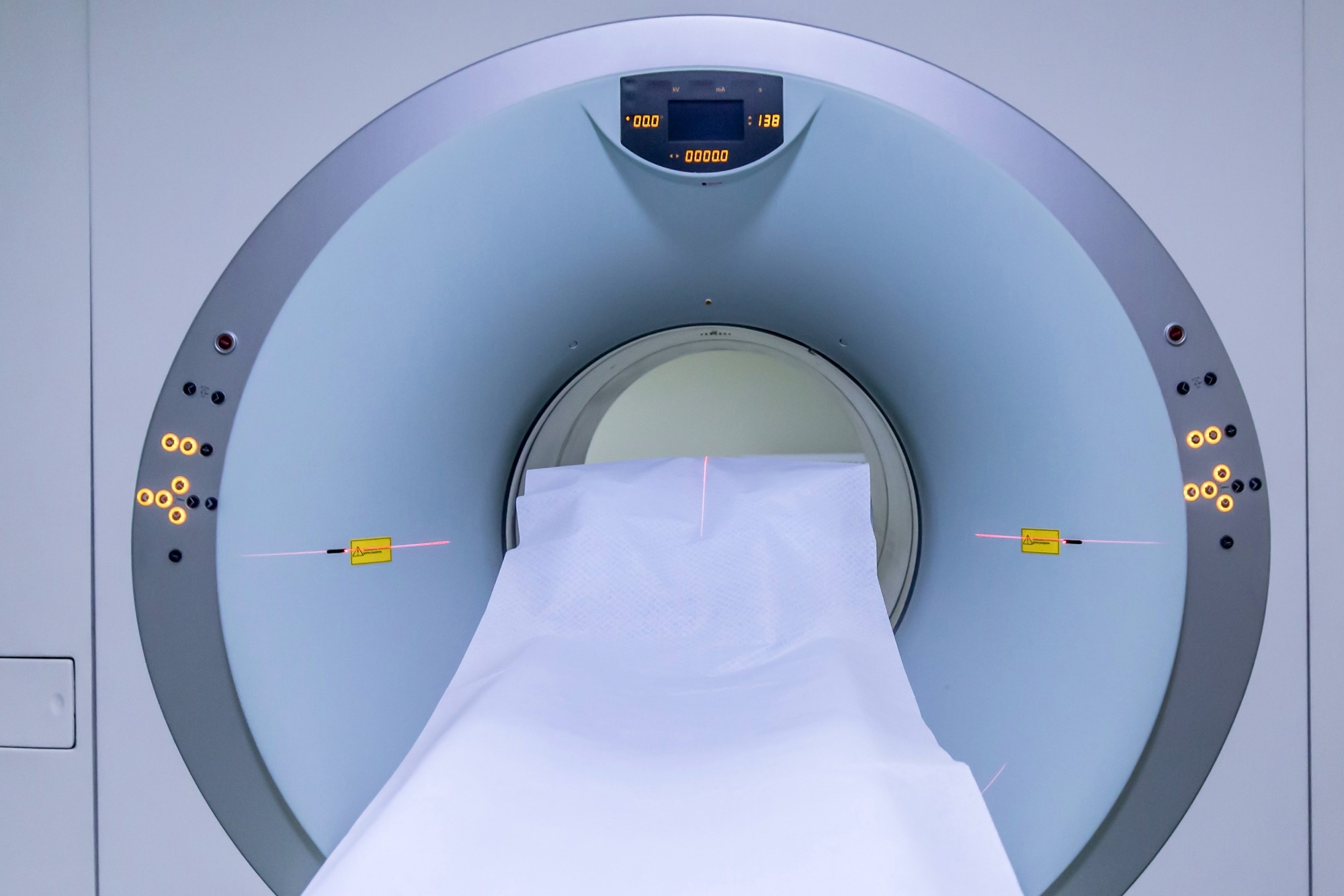
Functional magnetic resonance imaging (fMRI)
Often performed after a diagnosis is achieved, fMRI’s serve mainly to see whether surgery is a favorable option or not. Therefore, scans of this nature are performed roughly 24-48 hours before the scheduled surgery. The images help surgeons navigate through the human body, while in the operating room, showing the points of entry, the location of masses, and other important information. This differs from regular MRIs, which assess other patients’ organs, bones, or tissues.
fMRIs, and MRIs in general, use the duality between magnetic fields and radiofrequency energy to scan the brain. In actuality, the X-rays portray the hydrogen atoms responding to these pulses in energy. This apparatus uses the magnetic properties of living tissue to provide the contrast needed to generate these images. The energy emitted during the relaxation time (T1) of these hydrogen atoms is what the MRI scanner will pick up while the computer reconstructs this information to provide us with an image. If tumors or other major entities are present amidst the brain matter, the use of contrast dye will sometime be employed. This enhances the clarity and visibility of whatever the dye meets, such as target tissues or blood vessels.
A common mistake amongst aspiring medical students and radiologists is that computerized axial tomography (CAT) scans are a type of functional neuroimaging. Conversely, these series of X-ray images combine to reveal the size and shape of structures in the brain, falling under the practice of structural neuroimaging. Amongst other limitations, CAT scans do not have a high enough spatial resolution to be considered functional neuroimaging.
Functional neuroimaging in the monitoring of sleep patterns
Sleep is now being monitored in research settings using electroencephalography (EEG), a methodology that has been in use for a long time, speaking volumes about its practicality. The digital graph resulting from the information provided by electrodes will portray time on the x-axis and frequency in Hz on the y-axis. This will effectively monitor “brain waves,” also known as neural oscillations. The frequency of these brain waves is segmented into bands, each relating to different brain functions. There are oscillations produced at the delta band (0.5-4Hz), which are linked with deep sleep, theta waves (4-8Hz) associated with meditation, and alpha waves (8-12Hz), which indicate an extremely mild awareness. Though we are still aloof about which band corresponds to which specific action, it has been established that the higher frequency these waves are, the more alert the individual will be.
By studying the interworking of these oscillations during hours of sleep and activity, we garner essential information about brain health. Abnormal brain health, and an irregularity in circadian rhythms, have exhibited the amplification of Alzheimer’s symptoms and greater susceptibility to prion diseases. Through the use of EEG, we can monitor neuronal oscillations after some treatment or change of lifestyle is expressed, granting us a better idea of what behavior or treatment must be undergone. In addition, we see how much sleep an individual truly needs during a specified age in their life.
Using positron emission tomography (PET) to treat lymphoma
PET scans, another imperative form of functional neuroimaging, are accomplished by first injecting the patient with a radiopharmaceutical-like simple sugar, most often referred to as fluorodeoxyglucose (FDG). The apparatus will then pick up the gamma rays as the sugar flows through the bloodstream. Surveying this blood flow through the brain will highlight regions of the brain where more activity is present, useful when diagnosing aphasia, brain lesions, irregular masses, and other brain disorders.
While these scans are not ideal for all variants of cancer, it is essential in the monitoring of Hodgkin’s lymphoma, Burkitt lymphoma, and other T-cell lymphomas. PET can detect sites of disease spread within small nodes that would otherwise be undetectable using a CT scan or other form of X-ray technology. PET can also monitor within the bone marrow, a feature that CT scans do not possess. To know where cancerous masses are and how far they have metastasized is to know how to treat the disease. By determining what stage of cancer one has, we are made aware of how many cycles of chemotherapy and radiotherapy are required and in which areas they should be administered.
Before the normalization of PET scans, bone marrow biopsies were common practice – a procedure that could cause extreme pain, bleeding, infection, and death. This technique, amongst other function neuroimaging techniques, has greatly contributed to healthcare and medicine.
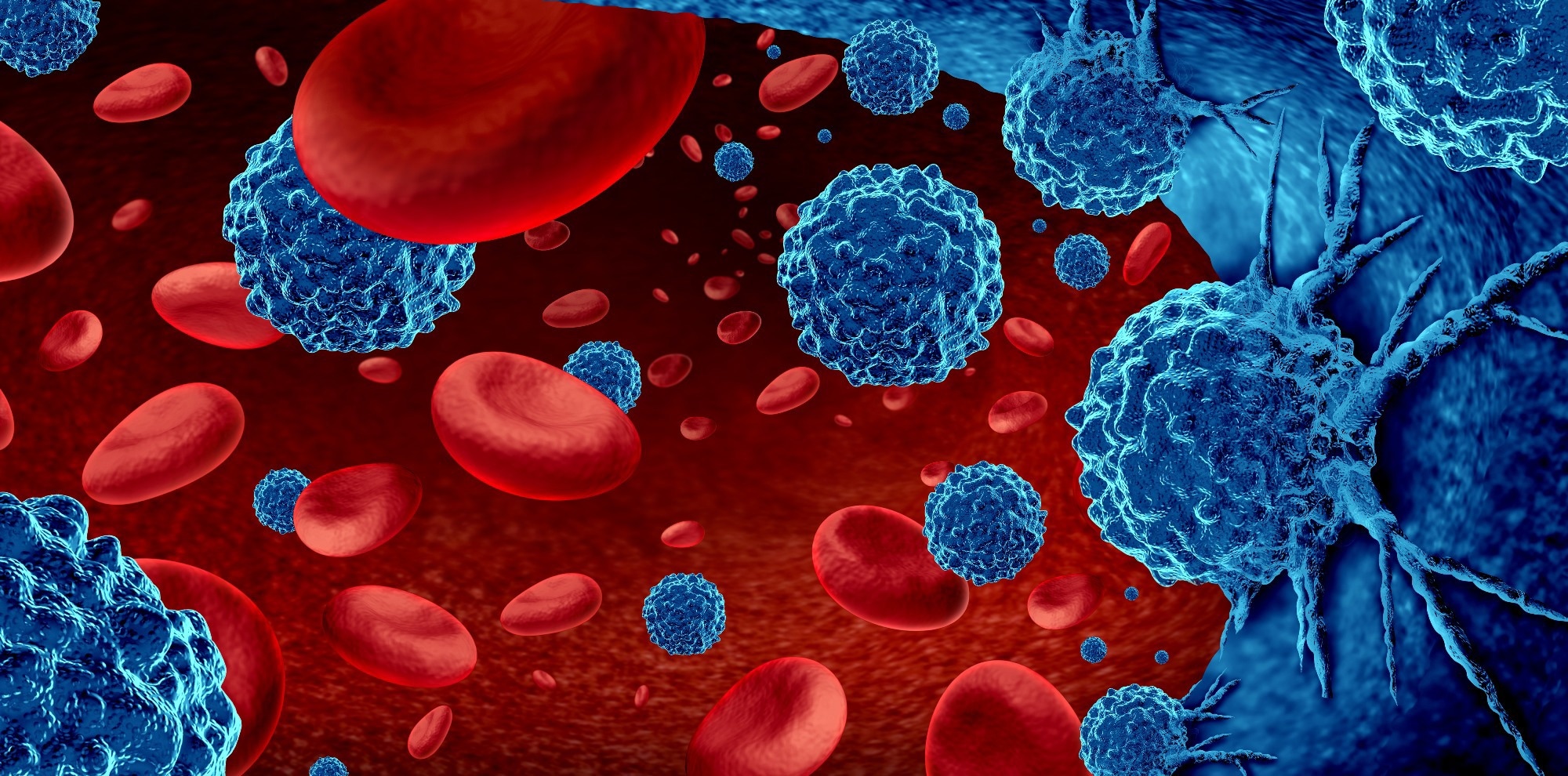
References
- Klimesch W. (2012). α-band oscillations, attention, and controlled access to stored information. Trends in cognitive sciences, 16(12), 606–617. https://doi.org/10.1016/j.tics.2012.10.007
- Khanna, N., Altmeyer, W., Zhuo, J., & Steven, A. (2015). Functional Neuroimaging: Fundamental Principles and Clinical Applications. The neuroradiology journal, 28(2), 87–96. https://doi.org/10.1177/1971400915576311
- Chow, M. S., Wu, S. L., Webb, S. E., Gluskin, K., & Yew, D. T. (2017). Functional magnetic resonance imaging and the brain: A brief review. World journal of radiology, 9(1), 5–9. https://doi.org/10.4329/wjr.v9.i1.5
- Gallamini A, Borra A. Role of PET in lymphoma. Curr Treat Options Oncol. 2014 Jun;15(2):248-61. doi: 10.1007/s11864-014-0278-4. PMID: 24619427.
- Dang-Vu, T. T., Schabus, M., Desseilles, M., Sterpenich, V., Bonjean, M., & Maquet, P. (2010). Functional neuroimaging insights into the physiology of human sleep. Sleep, 33(12), 1589–1603. https://doi.org/10.1093/sleep/33.12.1589
- Nofzinger EA. Functional neuroimaging of sleep. Semin Neurol. 2005 Mar;25(1):9-18. doi: 10.1055/s-2005-867070. PMID: 15798933.
Further Reading
[ARTICLE] Effectiveness of robot-assisted virtual reality mirror therapy for upper limb motor dysfunction after stroke: study protocol for a single-center randomized controlled clinical trial – Full Text
Posted by Kostas Pantremenos in Mirror therapy, Rehabilitation robotics, Virtual reality rehabilitation on September 5, 2022
Abstract
Background
Upper limb motor dysfunction is a common sequela of stroke, and its clinical efficacy needs to be improved. This protocol describes a trial to verify the clinical efficacy of robot-assisted virtual reality mirror therapy (RAVRMT) in improving upper limb motor dysfunction in stroke patients, and to explore the central mechanism by using functional magnetic resonance imaging (fMRI).
Methods
This trial will be a single-center, assessor-blinded, randomized controlled clinical study. Thirty-two eligible patients will be randomly divided into 2 groups according to the ratio of 1:1, namely virtual reality mirror therapy (VRMT) group and robot-assisted virtual reality mirror therapy (RAVRMT) group. The interventions will be performed once a day for 4 weeks. Primary outcome is Fugl–Meyer motor function assessment-Upper Extremity (FMA-UE), secondary outcomes are the Montreal Cognitive Assessment (MoCA), activities of daily living (ADL), quality of life (QOL), the pain visual analogue scale (VAS-pain) and fMRI. Adverse events will be recorded, and severe adverse events will be used as criteria to discontinue the intervention.
Discussion
Combined application of robot-assisted therapy and virtual reality mirror therapy could theoretically activate mirror neuron system and reward circuits to a greater extent, but further high-quality research is needed. The results of this trial will determine whether RAVRMT could better improve upper limb motor dysfunction after stroke and explore its central mechanism using fMRI.
Background
In recent years, stroke has become the second leading cause of death and the third leading cause of disability in the world [1]. Studies have shown that about 80% of acute stroke patients and more than 55% chronic stroke patients have upper limb motor dysfunction [2, 3], which seriously affects the quality of life of patients and their families [4, 5]. At present, the conventional rehabilitation methods for upper limb motor dysfunction after stroke consists of physical therapy [6], occupational therapy [7], but more than 50% of stroke patients still have long-term upper limb motor dysfunction [8]. The clinical efficacy of conventional rehabilitation methods in the treatment of upper limb motor dysfunction after stroke is not satisfactory, and new rehabilitation concepts and techniques are urgently needed.
How can we better promote the recovery of upper limb motor dysfunction after stroke? The disruption of motor-related brain networks is the primary cause of upper limb motor dysfunction after stroke, and another important cause is the upper limb disuse caused by long-term inactivity, so a single treatment for one cause is not adequate [9]. In stroke, the disruption of motor-related brain networks is the damage at the central level [10] and the upper limb disuse caused by long-term inactivity is the damage at the peripheral level [11]. As a clinical central intervention technology, mirror therapy can activate the motor-related brain network through action observation [12]. A recent meta-analysis demonstrates that mirror therapy can significantly improve upper limb motor dysfunction in stroke patients [13]. As a new kind of mirror therapy, virtual reality mirror therapy (VRMT) can provide immersion mirror therapy for stroke patients [14]. However, mirror therapy lacks the participation of proprioception [15], which may not be able to simulate the actual scene well and activate the motor-related brain network to the greatest extent. As a clinical peripheral intervention technology, a large number of studies have proved that robot-assisted therapy (RAT) can have a positive impact on the recovery of upper limb motor dysfunction in stroke patients through programmed task training [16, 17], including motor function and proprioception. At present, more and more studies show that mirror therapy combined with other interventions is superior to a single rehabilitation therapy in the treatment of upper limb motor dysfunction in stroke patients [18–20].
In this study, we designed a device that combined RAT and immersion VRMT to treat upper limb motor dysfunction after stroke. Moreover, the device can dynamically match the motion trajectory of the RAT with the motion images displayed in real time by the VRMT, so as to realize the cycle of “visual information input-visual information output-motion feedback” in the stroke patient. This is different from other combinations of a central intervention and a peripheral intervention for upper limb motor dysfunction after stroke [21, 22], which a central intervention and a peripheral intervention do not dynamically match each other in real time. In summary, the device is an innovative medical rehabilitation machine, which realizes the cycle of “visual information input-visual information output-motion feedback”, and provides patients with a full-body immersive experience that is consistent with vision, touch, and proprioception. We call the treatment robot-assisted virtual reality mirror therapy (RAVRMT) and the device RAVRMT robot. The RAVRMT robot is ultimately expected to activate mirror neuron system (MNS) and reward circuits to the greatest extent possible, thereby promoting the improvement of upper limb motor dysfunction in patients after stroke.
Therefore, we designed a randomized controlled trial to explore the clinical efficacy of RAVRMT in improving upper limb motor dysfunction in patients after stroke. Meanwhile, in this trial, functional magnetic resonance imaging (fMRI) scans will be performed to find significant changes in motor-related brain functional connectivity, and the motor-related brain functional network will be compared before and after the intervention to further reveal the brain neural mechanism of RAVRMT’s effects.[…]

Schematic diagram of virtual reality mirror therapy
[Abstract] Effects of virtual reality-based motor rehabilitation: a systematic review of fMRI studies
Posted by Kostas Pantremenos in Cognitive Rehabilitation, Neuroplasticity, Virtual reality rehabilitation on January 5, 2022
Abstract
Background: The use of virtual reality (VR) as a rehabilitation tool has been shown to induce motor and cognitive improvements in different populations. Functional magnetic resonance imaging (fMRI) has been used to investigate neuroplasticity resulting from these treatments. We hypothesize that VR rehabilitation induces functional improvement and brain changes that can be detected by fMRI.
Objective: To systematically review the effects of VR intervention on the cortical reorganization measured by fMRI and associated with functional improvement.
Methods: We performed a systematic review of studies published between 2005 and 2021. Papers were retrieved from six databases using the following keywords: “motor rehabilitation”, “fMRI” and “virtual reality”. Case studies, pre-post studies, cross-sectional studies, and randomized controlled trials published were included. Manuscripts were assessed by The NIH Study Quality Assessment Tools to determine their quality.
Results: Twenty-three articles met our eligibility criteria: 18 about VR rehabilitation in stroke and five on other clinical conditions (older adults, cerebral palsy, and Parkinson’s disease). Changes in neural patterns of activation and reorganization were revealed in both the ipsilesional and the contralesional hemispheres. Results were located mainly in the primary motor cortex, sensorimotor cortex and supplementary motor area in post-stroke patients in the acute, subacute, and chronic rehabilitation phases, and were associated with functional improvement after VR intervention. Similar effects were observed in older adults and in patients with other neurological diseases with improved performance.
Conclusion: Most stroke-related studies showed either restoration to normal or increase of activation patterns or relateralization at/to the ipsilesional hemisphere, with some also reporting a decrease in activity or extent of activation after VR therapy. In general, VR intervention demonstrated evidence of efficacy both in neurological rehabilitation and in performance improvement of older adults, accompanied by fMRI evidence of brain reorganization.
Similar articles
- Proactive Motor Functional Recovery Following Immersive Virtual Reality-Based Limb Mirroring Therapy in Patients with Subacute Stroke.Mekbib DB, Zhao Z, Wang J, Xu B, Zhang L, Cheng R, Fang S, Shao Y, Yang W, Han J, Jiang H, Zhu J, Ye X, Zhang J, Xu D.Neurotherapeutics. 2020 Oct;17(4):1919-1930. doi: 10.1007/s13311-020-00882-x.PMID: 32671578 Free PMC article.
- Neural Patterns of Reorganization after Intensive Robot-Assisted Virtual Reality Therapy and Repetitive Task Practice in Patients with Chronic Stroke.Saleh S, Fluet G, Qiu Q, Merians A, Adamovich SV, Tunik E.Front Neurol. 2017 Sep 4;8:452. doi: 10.3389/fneur.2017.00452. eCollection 2017.PMID: 28928708 Free PMC article.
- Virtual reality-induced cortical reorganization and associated locomotor recovery in chronic stroke: an experimenter-blind randomized study.You SH, Jang SH, Kim YH, Hallett M, Ahn SH, Kwon YH, Kim JH, Lee MY.Stroke. 2005 Jun;36(6):1166-71. doi: 10.1161/01.STR.0000162715.43417.91. Epub 2005 May 12.PMID: 15890990 Clinical Trial.
- Effects of Virtual Reality Intervention on Neural Plasticity in Stroke Rehabilitation: A Systematic Review.Hao J, Xie H, Harp K, Chen Z, Siu KC.Arch Phys Med Rehabil. 2021 Aug 2:S0003-9993(21)01305-8. doi: 10.1016/j.apmr.2021.06.024. Online ahead of print.PMID: 34352269 Review.
- Virtual Reality Intervention to Help Improve Motor Function in Patients Undergoing Rehabilitation for Cerebral Palsy, Parkinson’s Disease, or Stroke: A Systematic Review of Randomized Controlled Trials.Amirthalingam J, Paidi G, Alshowaikh K, Iroshani Jayarathna A, Salibindla DBAMR, Karpinska-Leydier K, Ergin HE.Cureus. 2021 Jul 30;13(7):e16763. doi: 10.7759/cureus.16763. eCollection 2021 Jul.PMID: 34367835 Free PMC article. Review.
[ARTICLE] BCI Training Effects on Chronic Stroke Correlate with Functional Reorganization in Motor-Related Regions: A Concurrent EEG and fMRI Study – Full Text PDF
Posted by Kostas Pantremenos in REHABILITATION on January 23, 2021
Abstract
Brain–computer interface (BCI)-guided robot-assisted training strategy has been increasingly applied to stroke rehabilitation, while few studies have investigated the neuroplasticity change and functional reorganization after intervention from multimodality neuroimaging perspective. The present study aims to investigate the hemodynamic and electrophysical changes induced by BCI training using functional magnetic resonance imaging (fMRI) and electroencephalography (EEG) respectively, as well as the relationship between the neurological changes and motor function improvement. Fourteen chronic stroke subjects received 20 sessions of BCI-guided robot hand training.
Simultaneous EEG and fMRI data were acquired before and immediately after the intervention. Seed-based functional connectivity for resting-state fMRI data and effective connectivity analysis for EEG were processed to reveal the neuroplasticity changes and interaction between different brain regions. Moreover, the relationship among motor function improvement, hemodynamic changes, and electrophysical changes derived from the two neuroimaging modalities was also investigated.
This work suggested that (a) significant motor function improvement could be obtained after BCI training therapy, (b) training effect significantly correlated with functional connectivity change between ipsilesional M1 (iM1) and contralesional Brodmann area 6 (including premotor area (cPMA) and supplementary motor area (SMA)) derived from fMRI, (c) training effect significantly correlated with information flow change from cPMA to iM1 and strongly correlated with information flow change from SMA to iM1 derived from EEG, and (d) consistency of fMRI and EEG results illustrated by the correlation between functional connectivity change and information flow change. Our study showed changes in the brain after the BCI training therapy from chronic stroke survivors and provided a better understanding of neural mechanisms, especially the interaction among motor-related brain regions during stroke recovery. Besides, our finding demonstrated the feasibility and consistency of combining multiple neuroimaging modalities to investigate the neuroplasticity change.[…]
[Book Review] Introduction to Neuroimaging Analysis
Posted by Kostas Pantremenos in Books, Radiology/Imaging technology on December 19, 2020
A Book Review on
Introduction to Neuroimaging Analysis
Mark Jenkinson and Michael Chappell, (Oxford: Oxford University Press), 2018, 276 pages, ISBN: 978-0198816300 (also available as E-book).
Magnetic resonance imaging (MRI) has become an essential research tool in human neuroscience, and MRI data analysis is a critical skill for students and researchers in the field. Learning—and teaching—the fundamentals of MRI data analysis is tricky, time-consuming and sometimes frustrating. Quantitative analyses of structural and functional MRI depend on knowledge in many disparate areas, such as MR physics, statistics. and computer programming, but also neurophysiology and neuroanatomy. Most of all, MRI data analysis is a practical skill and requires the knowledge of one of the major analysis software packages.
A new book by Jenkinson and Chappell, both at the University of Oxford and experienced teachers of MRI analysis, aims to instruct students and researchers who are new to the field of neuroimaging research.
Chapter 1 of Introduction to Neuroimaging Analysis provides a brief overview of the main MRI modalities, walks the reader through the several steps of a first, imaginary neuroimaging study and gives a concise introduction to MR physics and scanner hardware. Chapter 2 brings a more in-depth description of MRI modalities, primarily structural, functional, and diffusion MRI. The rest of the book discusses quantitative techniques of MRI analysis. Chapter 3, providing a concise and clear summary of structural, functional, and diffusion imaging pipelines, is the heart of the book. If you are a newbie to MRI analysis, you would want to read this chapter, and read it several times to absorb the many concepts introduced here. Additional chapters on brain extraction, registration as well as motion and distortion correction present a comprehensive discussion of major steps in data preprocessing. Finally, chapter 7 illustrates a more advanced method, surface-based analysis of structural and functional data.
The book is accompanied by additional chapters on the Neuroimaging Primers website. The MRI Physics for Neuroimaging appendix provides a short, non-technical overview of the basics of MR physics. The General Linear Model for Neuroimaging appendix is a very well-written and illustrated introduction to the centerpiece of statistical analysis of MRI.
The unique feature of this book is the use of Example Boxes that provide practical knowledge and skills for MRI analysis. Many of these boxes are linked to separate web pages on the Neuroimaging Primers website. These web pages start with instructions how to install and run FSL, the authors’ favorite neuroimaging software package (of which Mark Jenkinson is principal developer). FSL is a software package that includes programs for the analysis of structural MRI, task-based and resting state functional MRI and diffusion tensor imaging and that runs on Unix systems (such as Mac OS), on Linux machines and inside a virtual Linux machine on Windows PCs.
Additional web pages cover, for example, analysis of structural MRI (such as tissue-type segmentation), diffusion tensor imaging (such as tractography) and task-based functional MRI. These web pages act as short online tutorials; they contain MRI data sets for download and demonstrate how to analyze the data in FSL and visualize the results using FSL’s image viewer FSLeyes. We ran several of these tutorials and all worked well. The data downloaded from the tutorial web page not only include the original data set, but also FSL’s results folder. This allows you to check and comprehend the results of every single analysis introduced on the web pages without running the analysis yourself or even installing FSL. These tutorials are ideal for homework, they are written with the student in mind and easy to follow.
Running FSL through the graphical user interface requires only rudimentary UNIX skills, which are introduced on the “Getting Started” web page. Those who long for additional UNIX proficiency simply take the link to the FSL course web page, containing Introduction to UNIX videos and a UNIX handout. To sum up, if you are FSL-inclined and teaching an introductory course on MRI data analysis covering the basics of structural MRI, fMRI, and DTI, this book is everything you need. If the main focus of your course is task-based functional MRI, you may need more detail and you may want to add recent review articles or chapters of the established fMRI textbooks (see below) to the reading list.
If you prefer a different software package, let’s say AFNI, Brainvoyager or SPM, the new textbook by Jenkinson and Chappell is still a very helpful resource. The main text of the book and the online appendices are software-independent. The original and the analyzed data sets on the web pages can be inspected by any nifti file viewer. The web page on MRI artifacts, e.g., offers 25 MRI data sets covering various artifacts. Of course, the web pages describe the commands FSL uses to perform a certain analysis step. If you would like your student to learn a non-FSL package through these web pages, you would need to provide the necessary commands and code.
Jenkinson and Chappell’s book is the most recent of several publications on neuroimaging methods. The textbook by Huettel, Song and McCarthy, Functional Magnetic Resonance Imaging, now in its 3rd edition, has gained a wide readership among students and is used in numerous university courses (Huettel et al., 2014). The textbook by Huettel et al. is useful for undergraduate and graduate students who expect a more detailed, narrative account and who are primarily interested in task-based fMRI. The Handbook of Functional MRI Data Analysis by Poldrack, Mumford, and Nichols presents a more concise and slightly more technical discussion of fMRI analysis techniques (Poldrack et al., 2011). If your focus is on resting state fMRI, you may turn to Introduction to Resting State fMRI Functional Connectivity by Bijsterbosch, Smith and Beckmann, a recent addition to the Oxford Neuroimaging Primers series (Bijsterbosch et al., 2018).
Taken together, Jenkinson and Chappell have crafted an easily accessible and highly readable introduction to the analysis of structural MRI, functional MRI and diffusion tensor imaging. With the online tutorials, tightly connected with the main text, the reader will obtain useful hands-on methodological experience. The combination of main text and online tutorial makes this book an ideal companion for students and researchers who plan to undergo their first steps in structural, functional, or diffusion tensor imaging analysis.
Hopefully, this book will find many readers and see a 2nd edition in the near future. In the next edition, a few points should be revised. As the general linear model has become so important for MRI analysis, the authors may consider including their online chapter on the general linear model in the 2nd edition of the printed book. If necessary, Appendix A, Introduction to Brain Anatomy, might be removed and made online-only. Moreover, the online tutorial on Physiological noise modeling, announced but not available yet, would be very much appreciated. Finally, there is one crucial concept in neuroimaging analysis that we missed in this, otherwise excellent, book: a detailed description of methods for multiple comparison correction. This topic has been widely discussed, even in popular media, following the publication of Eklund et al.’s paper in 2016 (Eklund et al., 2016).