Posts Tagged motor learning
[Abstract] Basis and Clinical Evidence of Virtual Reality-Based Rehabilitation of Sensorimotor Impairments After Stroke – BOOK Chapter
Posted by Kostas Pantremenos in Books, Virtual reality rehabilitation on November 19, 2022
Abstract
In the recent years, the use of virtual reality (VR) to enhance motor skills of persons with activity and participation restriction due to disease or injury has become an important area of research and translation to practice. In this chapter, we describe the design of such VR systems and their underlying principles, such as experience-dependent neuroplasticity and motor learning. Further, psychological constructs related to motivation, including salience, goal setting, and rewards are commonly utilized in VR to optimize motivation during rehabilitation activities. Hence, virtually simulated activities are considered to be ideal for [1] the delivery of specific feedback, [2] the ability to perform large volumes of training, and [3] the presentation of precisely calibrated difficulty levels, which maintain a high level of challenge throughout long training sessions. These underlying principles are contrasted with a growing body of research comparing the efficacy of VR with traditionally presented rehabilitation activities in persons with stroke that demonstrate comparable or better outcomes for VR. In addition, a small body of literature has utilized direct assays of neuroplasticity to evaluate the effects of virtual rehabilitation interventions in persons with stroke. Promising developments and findings also arise from the use of off-the-shelf video game systems for virtual rehabilitation purposes and the integration of VR with robots and brain-computer interfaces. Several challenges limiting the translation of virtual rehabilitation into routine rehabilitation practice need to be addressed but the field continues to hold promise to answer key issues faced by modern healthcare.
References
Burdea GC, Coiffet P. Virtual reality technology. Presence. 2003;12(6):663–4.
Wilson PN, Foreman N, Stanton D. Virtual reality, disability and rehabilitation. Disabil Rehabil. 1997;19(6):213–20.
CrossRef CAS PubMed Google Scholar
Adamovich SV, et al. Sensorimotor training in virtual reality: a review. NeuroRehabil. 2009;25(1):29–44.
Slater M. Place illusion and plausibility can lead to realistic behaviour in immersive virtual environments. Philos Trans R Soc B Biol Sci. 2009;364(1535):3549–57.
Slater M, Wilbur S. A framework for immersive virtual environments (FIVE): Speculations on the role of presence in virtual environments. Presence. 1997;6(6):603–16.
Baños RM, et al. Immersion and emotion: their impact on the sense of presence. Cyberpsychol Behav. 2004;7(6):734–41.
CrossRef PubMed Google Scholar
Llorens R, et al. Tracking systems for virtual rehabilitation: objective performance vs subjective experience: a practical scenario. Sensors. 2015;15(3):6586–606.
CrossRef PubMed PubMed Central Google Scholar
Stanney KM, Mourant RR, Kennedy RS. Human factors issues in virtual environments: a review of the literature. Presence. 1998;7(4):327–51.
Arzy S, et al. Neural mechanisms of embodiment: asomatognosia due to premotor cortex damage. Arch Neurol. 2006;63(7):1022–5.
CrossRef PubMed Google Scholar
Legrand D. The bodily self: the sensori-motor roots of pre-reflective self-consciousness. Phenom Cog Sci. 2006;5(1):89–118.
For more Download references
[ARTICLE] Human-machine-human interaction in motor control and rehabilitation: a review – Full Text
Posted by Kostas Pantremenos in REHABILITATION on September 25, 2022
Abstract
Background
Human-human (HH) interaction mediated by machines (e.g., robots or passive sensorized devices), which we call human-machine-human (HMH) interaction, has been studied with increasing interest in the last decade. The use of machines allows the implementation of different forms of audiovisual and/or physical interaction in dyadic tasks. HMH interaction between two partners can improve the dyad’s ability to accomplish a joint motor task (task performance) beyond either partner’s ability to perform the task solo. It can also be used to more efficiently train an individual to improve their solo task performance (individual motor learning). We review recent research on the impact of HMH interaction on task performance and individual motor learning in the context of motor control and rehabilitation, and we propose future research directions in this area.
Methods
A systematic search was performed on the Scopus, IEEE Xplore, and PubMed databases. The search query was designed to find studies that involve HMH interaction in motor control and rehabilitation settings. Studies that do not investigate the effect of changing the interaction conditions were filtered out. Thirty-one studies met our inclusion criteria and were used in the qualitative synthesis.
Results
Studies are analyzed based on their results related to the effects of interaction type (e.g., audiovisual communication and/or physical interaction), interaction mode (collaborative, cooperative, co-active, and competitive), and partner characteristics. Visuo-physical interaction generally results in better dyadic task performance than visual interaction alone. In cases where the physical interaction between humans is described by a spring, there are conflicting results as to the effect of the stiffness of the spring. In terms of partner characteristics, having a more skilled partner improves dyadic task performance more than having a less skilled partner. However, conflicting results were observed in terms of individual motor learning.
Conclusions
Although it is difficult to draw clear conclusions as to which interaction type, mode, or partner characteristic may lead to optimal task performance or individual motor learning, these results show the possibility for improved outcomes through HMH interaction. Future work that focuses on selecting the optimal personalized interaction conditions and exploring their impact on rehabilitation settings may facilitate the transition of HMH training protocols to clinical implementations.
Introduction
Human-human interaction
Humans often interact with each other while performing motor tasks, either to improve performance by working as a group or to learn from each other. Some motor tasks, such as exercising an injured joint, can be accomplished by a single person, but also allow two or more people (e.g., the therapist and the patient) to interact during performance of the task. Other motor tasks require multiple people to interact and cannot be performed by a single person (e.g., carrying a large table). Human-human (HH) interaction in tasks such as these can take the form of audiovisual communication and/or physical interaction.
In recent years, many studies have compared motor task performance under solo conditions and under different types of interaction among groups of two or more [1,2,3,4,5]. For tasks that can be performed with one or more people, we refer to task performance as the individual ability to accomplish a motor task during or without interaction. Similarly, for tasks that require multiple people, task performance is the ability to perform the task as a group.
There has been an increasing interest also for the effects of HH interaction on individual motor learning [2, 6,7,8] for motor tasks. In this article, we refer to individual motor learning as the solo task performance difference before and after a training period. This training phase can be performed individually or by interacting with someone else. In this review, we are interested in the learning of only individuals but not groups. Therefore, we do not analyze motor learning for tasks that require multiple people.
Studies that have demonstrated better outcomes of HH interaction than solo performance or training often attributed these positive effects to (1) increased motivation and interest [3, 9, 10]; (2) the ability to estimate partner’s motion, [11, 12]; and (3) the summing of physical effort, or the ability of partners to communicate and adopt specialized roles in performing a physical task [1, 13].
Even though important results have been obtained showing the potential benefits of human-human interaction, it is unclear how different kinds of interactions impact task performance and individual motor learning. A better understanding of how humans interact with each other in motor control tasks will have a significant impact on rehabilitation robotics, industrial collaborative robotics, and social robotics.[..]

[ARTICLE] Virtual Feedback for Arm Motor Function Rehabilitation after Stroke: A Randomized Controlled Trial – Full Text
Posted by Kostas Pantremenos in Paretic Hand, REHABILITATION, Virtual reality rehabilitation on July 14, 2022
Abstract
A single-blind randomized controlled trial was conducted to compare whether the continuous visualization of a virtual teacher, during virtual reality rehabilitation, is more effective than the same treatment provided without a virtual teacher visualization, for the recovery of arm motor function after stroke. Teacher and no-teacher groups received the same amount of virtual reality therapy (i.e., 1 h/d, 5 dd/w, 4 ww) and an additional hour of conventional therapy. In the teacher group, specific feedback (“virtual-teacher”) showing the correct kinematic to be emulated by the patient was always displayed online during exercises. In the no-teacher group patients performed the same exercises, without the virtual-teacher assistance. The primary outcome measure was Fugl-Meyer Upper Extremity after treatment. 124 patients were enrolled and randomized, 62 per group. No differences were observed between the groups, but the same number of patients (χ2 = 0.29, p = 0.59) responded to experimental and control interventions in each group. The results confirm that the manipulation of a single instant feedback does not provide clinical advantages over multimodal feedback for arm rehabilitation after stroke, but combining 40 h conventional therapy and virtual reality provides large effect of intervention (i.e., Cohen’s d 1.14 and 0.92 for the two groups, respectively).
1. Introduction
Impairments of the upper limb are the most disabling after stroke [1]. Among stroke survivors, 73% to 88% have acute hemiparesis [2]. Motor impairment are related to diverse aspects of movement, such as motor planning, execution, learning, and control [3]. Additionally, loss of sensation can contribute to motor control impairment due to inaccurate feedback affecting both task planning and voluntary motor output [4]. Plasticity in the motor cortex is more learning-dependent than use-dependent, since mere repetition of known movements does not induce neurophysiological and neuroanatomical changes, instead occurring when new motor skills are acquired [5,6]. Theoretical models propose different learning-paradigms (i.e., unsupervised, supervised, reinforced) [7] based on neuroplasticity, all mediated by a high number of movement repetitions, amplification of results, and multimodal augmented feedback (e.g., visual, verbal, haptic, auditory, kinematic) provided to subjects as coherently as possible with action execution [7,8,9]. Evidence on healthy subjects demonstrated that emulating the correct kinematic of a virtual teacher (augmented feedback), presented in a virtual environment, can promote learning of motor tasks better than training the same skill in a real environment, or in a virtual scenario without the virtual teacher. This evidence supports the hypothesis that reinforced learning-paradigms can enhance motor learning more than supervised and unsupervised learning-paradigms [10]. Virtual reality (VR) is a computer-based technology that is used as a therapeutic modality to provide an enriched training environment, aimed to improve motor learning by augmented feedback. Virtual tasks can be graded by clinicians for progressively challenging practice [7,11], with the advantage of being interesting, enjoyable, and motivating for patients [11]. In the last 15 years, the use of “reinforced feedback in virtual environment” for the recovery of upper limb after stroke, has been extensively investigated at the IRCCS San Camillo Hospital (Venice, Italy). A first longitudinal pilot study demonstrated that 20 h of intensive training induced significant changes in motor function and autonomy [12]. Then, the following controlled studies provided evidence that effects of VR were better than the same amount of conventional therapy (CT), both under experimental conditions [13] and in real clinical settings [14]. Recent evidence from extended meta-analyses in patients after stroke confirmed that VR is more effective than CT for the recovery of arm motor function measured by the Fugl-Meyer Assessment (FMA) scale, both as a single treatment and in addition to CT, when delivered for at least 15 h of intervention [15,16]. However, most of the factors influencing therapy outcome are still unknown, including the contextual factors (e.g., relational style of the therapist, expectations, mindset, and personality traits) composing the clinical setting [17,18]. To date, no studies have compared different VR settings displaying or not a virtual teacher as feedback. Based on these assumptions, we designed this clinical trial to transfer feasible theoretical models into clinical practice, to assess their potential relevance.
The purpose of the study was to assess whether the continuous visualization of a virtual teacher is effective in improving arm motor function in patients after stroke.
2. Materials and Methods
2.1. Trial Design and Population
A single-blind randomized controlled trial (RCT) was carried out on stroke patients with upper limb impairment, to compare the effects of displaying (i.e., Teacher group) or not (i.e., No-Teacher group) a continuous virtual teacher during a VR treatment. All consecutive inpatients admitted to the Stroke Rehabilitation Unit at the San Camillo IRCCS hospital in Venice (Italy) from April 2013 to March 2020 were considered eligible for the study. For better reporting of interventions, CONsolidated Standards of Reporting Trials (CONSORT) 2010 guidelines and its extension CONSORT Statement for Randomized Trials of Nonpharmacologic Treatments and Template for Intervention Description and Replication (TIDieR) checklist have been used [19,20,21]. All patients were informed on the aim of the study, and signed an informed consent form. Informed consent includes also procedures to withdraw at any time from the study, explicating that the decision will not affect the quality of health care received during hospitalization. Patients enrolled were randomly assigned to experimental or control groups according to a computer generated (Microsoft Excel) block random sequence. The allocation sequence was concealed from the principal investigator enrolling patients in sequentially numbered, opaque, and sealed envelopes. The researcher responsible for the randomization was independent of the assessors, assuring blindness to treatment allocation and randomization procedures.
Inclusion criteria for study population were: patients diagnosed with the first unilateral cortical-subcortical stroke, (ischemic or hemorrhagic); older than 18 years; a score between 0 (excluded) and 4 points (excluded) at the Italian version of the National Institutes of Health Stroke Scale [NIHSS-IT] upper limb subitem. Exclusion criteria were: bilateral or cerebellum infarcts; unstable medical conditions; fractures; major depressive disorders; traumatic brain injury; other neurological conditions; epilepsy; severe neglect; severe apraxia affecting evaluation; severe impairment of verbal comprehension hampering communication, not released informed consent, or lack of compliance.
2.2. The Virtual Reality Rehabilitation System
The Virtual Reality Rehabilitation System (VRRS®—Khymeia Group, Ltd., Noventa Padovana, Italy) was used to provide the virtual tasks. It consists of a computer connected to a 6-degrees of freedom electromagnetic motion tracking system (G4, Polhemus Ltd., Colchester, VT, USA) and a high-resolution screen displaying the virtual scenarios (Figure 1). The patient sits on a chair in front of the screen and wears a sensorized glove on the paretic arm (Figure 2). The glove does not provide haptic feedback, but only contains a motion tracking sensor, matched to the virtual object representing the end-effector of the biomechanical system. The virtual object is displayed in a shape similar to the real one and its displacement is synchronized in time and space between real and virtual environments.
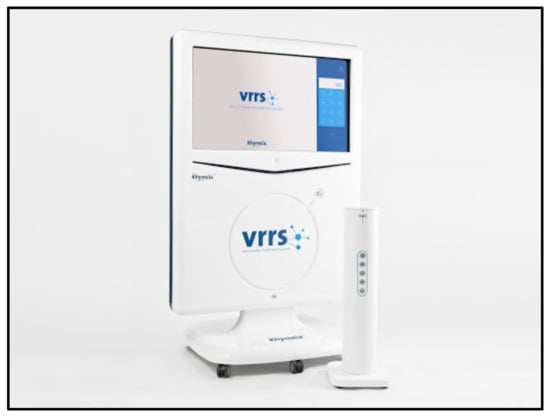
Figure 1. The Virtual Reality Rehabilitation System (VRRS®).
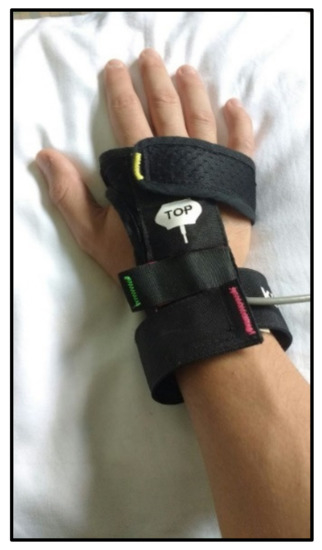
Figure 2. Sensorized electromagnetic glove
[…]
[ARTICLE] Assessing Impairments in Visuomotor Adaptation After Stroke – Full Text
Posted by Kostas Pantremenos in REHABILITATION on June 2, 2022
Abstract
Background:
Motor impairment in the arms is common after stroke and many individuals participate in therapy to improve function. It is assumed that individuals with stroke can adapt and improve their movements using feedback that arises from movement or is provided by a therapist. Here we investigated visuomotor adaptation in individuals with sub-acute and chronic stroke.
Objective:
We examined the impact of the stroke-affected arm (dominant or non-dominant), time post-stroke, and relationships with clinical measures of motor impairment and functional independence.
Methods:
Participants performed reaching movements with their arm supported in a robotic exoskeleton. We rotated the relationship between the motion of the participant’s hand and a feedback cursor displayed in their workspace. Outcome measures included the amount that participants adapted their arm movements and the number of trials they required to adapt.
Results:
Participants with stroke (n = 36) adapted less and required more trials to adapt than controls (n = 29). Stroke affecting the dominant arm impaired the amount of adaptation more than stroke affecting the non-dominant arm. Overall, 53% of participants with stroke were impaired in one or more measures of visuomotor adaptation. Initial adaptation was weakly correlated with time post-stroke, and the amount of adaptation correlated moderately with clinical measures of motor impairment and functional independence.
Conclusion:
Our findings reveal impairments in visuomotor adaptation that are associated with motor impairment and function after stroke. Longitudinal studies are needed to understand the relationship between adaptation and recovery attained in a therapy setting.
Introduction
Humans perform a variety of movements and activities while interacting with different objects and environments (e.g., bathing, dressing, and feeding). While most people perform activities of daily living with ease and proficiency, even healthy adults have to adapt when changes in properties of the task, environment, or body disrupt accuracy and produce movement errors. These errors can arise from transient disturbances, like a bus traveling over a bump when we are sipping from a drink, or systematic changes in properties of the body or environment, such as a loss of muscle strength, wearing a cast, or adjusting to new eyeglasses. Motor adaptation enables the nervous system to accommodate for changes in the body or environment that would otherwise produce errors and jeopardize task performance.1
Approximately 70% of individuals with stroke have motor impairments that compromise their ability to perform everyday tasks.2 Stroke rehabilitation often involves working with therapists to practice arm movements that are important for activities of daily living. It is assumed that individuals with stroke are capable of modifying their arm movements using error feedback that arises from movement or is provided by a therapist.3–6 This may be problematic as motor adaptation is reduced, on average, in chronic stroke and impedes the ability to use error feedback to interact with different objects and environments.7–11
Visuomotor rotations are a common method for assessing how the nervous system uses error feedback to adapt arm movements. The rotation creates a systematic discrepancy between the motion of the participant’s arm and a virtual feedback cursor displayed in their workspace. The resulting errors engage the nervous system in adapting arm movements.12 Adapting to visual transformations may also capture challenges encountered in daily life after stroke. Many tasks, like using a computer mouse to move a cursor or a mirror to facilitate grooming or dressing, alter the relationship between arm movements and their visual consequences.
Lesions in the left hemisphere of the brain reduce average levels of visuomotor adaptation in participants with chronic stroke, whereas lesions in the right hemisphere seem to have little influence on adaptation.8,10 It remains unclear if motor adaptation is impaired in the weeks and months after stroke (i.e., early to late sub-acute phases of recovery),13 when many individuals participate in rehabilitation. Beyond this, we have yet to establish the relationship between visuomotor adaptation and motor impairment measured by common clinical scales (e.g., Fugl-Meyer Assessment – Upper Extremity, FMA-UE), as well as how adaptation relates to difficulties performing activities of daily living (e.g., Funtional Independence Measure, FIM). Answering these questions will broaden our understanding of stroke impairment within the time period that many therapists work with patients.
Here we used a robotic task to assess visuomotor adaptation in individuals with sub-acute to chronic stroke. First, we hypothesized that participants with stroke would adapt less and require more trials to adapt than controls. Second, based on past studies,8,10 we expected that participants affected in their dominant arm would adapt less and slower than participants affected in their non-dominant arm. Finally, we examined how adaptation related to time post-stroke, and clinical assessments of motor impairment and functional independence.
Methods
Participants
We recruited a convenience sample of participants with stroke from rehabilitation units at the Foothills Medical Centre and Dr. Vernon Fanning Centre in Calgary, AB, Canada. Participants with stroke were also recruited from the community after spending time at these facilities and consenting to be contacted for research post-discharge.14,15 Our inclusion criteria were as follows: 18 years or older with clinical diagnosis of first-time ischemic or hemorrhagic stroke. Our exclusion criteria were as follows: prior diagnosis of secondary neurological conditions (e.g., Parkinson’s disease), musculoskeletal injuries that could interfere with the ability to perform the task, apraxia as identified by clinical assessment,16 or the inability to understand instructions for the task and clinical assessments.17 Control participants were recruited from the University of Calgary and surrounding community.14,15 Controls were considered eligible if they had no history of neurological conditions or recent upper limb musculoskeletal injuries. The control and stroke samples were matched for overall age and sex. The study protocol was approved by the Conjoint Health Research Ethics Board at the University of Calgary. Participants provided written informed consent before the experiment.
Experimental Apparatus and Task
Participants made planar reaching movements while seated with their arm supported against gravity by a robotic exoskeleton (Kinarm, Kingston, ON, Canada; Figure 1A). The robot recorded shoulder and elbow joint motion and was paired with a visual display that enabled participants to interact with virtual targets while moving their arm in a near frictionless environment. A small feedback cursor (0.8 cm diameter) was displayed in the participant’s workspace throughout the task (Figure 1B). Direct vision of the arm and hand was occluded by a metal shutter.

[…]
[ARTICLE] Effectiveness of action observation therapy based on virtual reality technology in the motor rehabilitation of paretic stroke patients: a randomized clinical trial – Full Text
Posted by Kostas Pantremenos in Paretic Hand, Virtual reality rehabilitation on April 20, 2022
Abstract
Background
The rehabilitation of paretic stroke patients uses a wide range of intervention programs to improve the function of impaired upper limb. A new rehabilitative approach, called action observation therapy (AOT) is based on the discovery of mirror neurons and has been used to improve the motor functions of adult stroke patients and children with cerebral palsy. Recently, virtual reality (VR) has provided the potential to increase the frequency and effectiveness of rehabilitation treatment by offering challenging and motivating tasks.
Methods
The purpose of the present project is to design a randomized controlled six-month follow-up trial (RCT) to evaluate whether action observation (AO) added to standard VR (AO + VR) is effective in improving upper limb function in patients with stroke, compared with a control treatment consisting of observation of naturalistic scenes (CO) without any action content, followed by VR training (CO + VR).
Discussion
AO + VR treatment may provide an addition to the rehabilitative interventions currently available for recovery after stroke and could be utilized within standard sensorimotor training or in individualized tele-rehabilitation.
Background
Stroke is the leading cause of disability among adults, and more than 60% of stroke survivors have motor deficits, particularly those related to the upper limb [1]. Stroke rehabilitation usually involves intensive motor training to promote adaptive plasticity by reducing motor deficits and developing new motor learning strategies [2, 3]. It has recently been proposed that the systematic use of action observation (AO) followed by imitation (known as action observation therapy [AOT]) is an effective way to improve motor function and to promote upper limb recovery in patients with motor disorders [4–7]. During a typical AOT session, a series of daily life actions (e.g., grasping a key and inserting it into a lock) are practiced for two to five weeks, with a frequency of three to five sessions per week. During each rehabilitation session, the patient is instructed by the therapist to observe a specific action performed by an actor, presented in a short video-clip on a monitor, and afterwards to reproduce the previously observed action with the paretic limb. The videos usually present a single motor act observed from different perspectives (e.g., subjective, front, or side view). This therapy is based on the neural model of the mirror neuron system (MNS), originally discovered in the monkey premotor and parietal cortex, which is formed by visuomotor neurons that become active both when a monkey performs a goal-directed motor act and when it simply observes the same or a similar motor act performed by the experimenter [8, 9]. A comparable MNS has been identified in humans using different electrophysiological and neuroimaging techniques [10]. In humans, the two main nodes of the MNS are the inferior parietal lobule (IPL) and the ventral premotor cortex (PMv), plus the caudal part of the inferior frontal gyrus (IFG) [11, 12].
In the first clinical trial focusing on, Ertelt and colleagues [13] found a significant improvement, evaluated by clinical functional scales, in adult patients with moderate upper limb motor deficits following the application of 18 sessions of AOT, corroborated by a significant increase in activation of the cortical MNS. This improvement was maintained for at least eight weeks after the conclusion of the treatment. More recently, Franceschini and colleagues [14, 15] performed two randomized controlled observer-blinded trials (RCTs) to evaluate the effectiveness of AOT as an add-on treatment to standard upper-limb rehabilitation in acute stroke patients, in which several measures of motor impairment and functional ability demonstrated an improvement over time. The efficacy of AOT has been confirmed in patients with Parkinson’s disease [16, 17], unilateral cerebral palsy (UCP) [18–22] and post-surgical orthopedic patients [23]. AOT is considered particularly useful for activating the motor system in conditions in which intensive motor training is not feasible because of the severity of the impairment of motor function or due to the presence of pain, inflammation, or muscle fatigue [7].
In recent years, new virtual reality-based (VR) rehabilitation treatments have been introduced in order to present rehabilitation exercises in a more practical and friendly setting [24]. These treatments are generally well accepted by patients because they offer several advantages: relatively low cost (in particular for semi-immersive versions), an engaging environment, the real-time personalization of exercises, greater adaptability to the patient’s clinical features and progress, and the opportunity for patients to record their motor performance and get feedback in real time. Furthermore, VR exercises usually require minimal supervision from a therapist, thereby facilitating home-based rehabilitation [25].
Several studies support the application of VR methods in the rehabilitation of the hemiplegic upper limb in stroke patients [26]. The largest study conducted to date involved 376 patients and demonstrated a positive effect of VR-based rehabilitation [27]. Moreover, recent literature reviews [28] have provided evidence for improvement in upper limb motor function and daily life activity after VR-based training compared with standard interventions. However, clinical evidence based on rigorous RCTs on the effect of the combined use of observation of actions followed by their immediate imitation in a VR environment (AO + VR therapy) is lacking, especially in the case of rehabilitation applied during the chronic phase after a stroke.
The main hypothesis of the study is that, for the recovery of motor function of hemiplegic stroke patients in the chronic phase, a combined rehabilitation treatment (AO + VR therapy) is more effective than a control treatment (control observation – CO) based on the observation of videos without motor content (e.g., environmental natural scenes), followed by the execution of actions in VR (CO + VR control therapy).
In sum, the planned trial will examine the following hypotheses:
- AO + VR is an effective tool for promoting upper limb control in paretic stroke patients, and its effects are greater than CO + VR control treatment.
- Motor performance, cognitive level, and structural brain damage assessed before treatment are correlated to the degree of improvement resulting from the AO + VR intervention.
- The AO + VR intervention results in greater plastic functional changes of the MNS activity compared with the effects of the CO + VR control treatment.[…]

(A) Experimental setting for both AO + VR and CO + VR interventions. (B1) Static frame showing an example of video-clip to be used for the experimental treatment AO + VR based on observation of actions performed by a model followed by subsequent imitation. (B2) Static frame of a video-clip illustrating natural scene, to be used in the CO + VR control treatment
[Abstract] A Control Framework for Adaptation of Training Task and Robotic Assistance for Promoting Motor Learning With an Upper Limb Rehabilitation Robot
Posted by Kostas Pantremenos in Paretic Hand, Rehabilitation robotics on April 20, 2022
Abstract
Robot-assisted rehabilitation has been a promising solution to improve motor learning of neurologically impaired patients. State-of-the-art control strategies are typically limited to the ignorance of heterogeneous motor capabilities of poststroke patients and therefore intervene suboptimally. In this article, we propose a control framework for robot-assisted motor learning, emphasizing the detection of human intention, generation of reference trajectories, and modification of robotic assistance. A real-time trajectory generation algorithm is presented to extract the high-level features in active arm movements using an adaptive frequency oscillator (AFO) and then integrate the movement rhythm with the minimum-jerk principle to generate an optimal reference trajectory, which synchronizes with the motion intention in the patient as well as the motion pattern in healthy humans. In addition, a subject-adaptive assistance modification algorithm is presented to model the patient’s residual motor capabilities employing spatially dependent radial basis function (RBF) networks and then combining the RBF-based feedforward controller with the impedance feedback controller to provide only necessary assistance while simultaneously regulating the maximum-tolerated error during trajectory tracking tasks. We conduct simulation and experimental studies based on an upper limb rehabilitation robot to evaluate the overall performance of the motor-learning framework. A series of results showed that the difficulty level of reference trajectories was modulated to meet the requirements of subjects’ intended motion, furthermore, the robotic assistance was compliantly optimized in response to the changing performance of subjects’ motor abilities, highlighting the potential of adopting our framework into clinical application to promote patient-led motor learning.
[ARTICLE] Bimanual motor skill learning with robotics in chronic stroke: comparison between minimally impaired and moderately impaired patients, and healthy individuals – Full Text
Posted by Kostas Pantremenos in Paretic Hand, Rehabilitation robotics on March 22, 2022
Abstract
Background
Most activities of daily life (ADL) require cooperative bimanual movements. A unilateral stroke may severely impair bimanual ADL. How patients with stroke (re)learn to coordinate their upper limbs (ULs) is largely unknown. The objectives are to determine whether patients with chronic supratentorial stroke could achieve bimanual motor skill learning (bim-MSkL) and to compare bim-MSkL between patients and healthy individuals (HIs).
Methods
Twenty-four patients and ten HIs trained over 3 consecutive days on an asymmetrical bimanual coordination task (CIRCUIT) implemented as a serious game in the REAplan® robot. With a common cursor controlled by coordinated movements of the ULs through robotic handles, they performed as many laps as possible (speed constraint) on the CIRCUIT while keeping the cursor within the track (accuracy constraint). The primary outcome was a bimanual speed/accuracy trade-off (biSAT), we used a bimanual coordination factor (biCO) and bimanual forces (biFOP) for the secondary outcomes. Several clinical scales were used to evaluate motor and cognitive functions.
Results
Overall, the patients showed improvements on biSAT and biCO. Based on biSAT progression, the HI achieved a larger bim-MSkL than the patients with mild to moderate impairment (Fugl-Meyer Assessment Upper Extremity (FMA-UE): 28–55, n = 15) but not significantly different from those with minimal motor impairment (FMA-UE: 66, n = 9). There was a significant positive correlation between biSAT evolution and the FMA-UE and Stroke Impact Scale.
Conclusions
Both HI and patients with chronic stroke training on a robotic device achieved bim-MSkL, although the more impaired patients were less efficient. Bim-MSkL with REAplan® may be interesting for neurorehabilitation after stroke.
Background
Stroke is a major cause of long-term disability worldwide [1, 2], and one of the most frequent impairments is hemiparesis, which is characterized by weakness, lack of control, increased muscle tone on the contralesional upper limb (UL) and lower limb or hemibody, and deteriorating independence in activities of daily life (ADL), especially walking, dressing or eating [3]. Critically, other impairments (e.g., somatosensory, visual, and cognitive), whether isolated or combined, also significantly deteriorate ADL. Most ADL require skilled bimanual coordination that can be impaired by a stroke, thus leading to a loss of independence that may in turn lead to a 50% reduction in quality of life [4]. Despite rehabilitative care provided during the acute phase of stroke, 30% of patients still suffer from participation restrictions after four years [5]. It has been suggested that neurorehabilitation should not focus exclusively on impairments of the paretic arm or hand and should instead consider more bimanual actions and activities [6,7,8]. After a unilateral stroke, impairments of the contralesional UL can deteriorate bimanual actions [9], thus supporting the importance of training both ULs to achieve better functional recovery in (bimanual) ADL [8, 10]. Interestingly, during bilateral cooperative movements (e.g., opening a bottle), neural coupling from the ipsilesional to the contralesional (impaired) UL is preserved in most patients with stroke, suggesting the relevance of bilateral training that supports cooperative hand movements for ADL [11]. During bimanual training, various tasks can be utilized to promote intensive and repetitive coordinated movement of the ULs. A classification of bimanual tasks has been proposed for different bimanual actions. Grossly, two types of tasks can be distinguished: those with symmetrical movements that engage homologous muscles (e.g., picking up a box simultaneously with both hands) and those with asymmetrical movements that engage nonhomologous muscles nonsimultaneously (e.g., cutting a piece of steak). Similarly, two types of task goals can be distinguished: independent goals (e.g., one hand lifting a cup and the other hand lifting a glass simultaneously) versus common goals (e.g., both hands working together to accomplish a common task) [8]. Many bilateral actions, such as arms swinging during bipedal locomotion, seem to depend on “default-mode” neural coupling. However, in most skilled ADL, bimanual actions are accomplished through asymmetrical movements that cooperate to achieve a common goal, e.g., buttoning a skirt or changing the gear while steering a car. Such complex bimanual, cooperative, asymmetrical skills have to be learned.
After a stroke, motor skill learning (MSkL) plays a key role in recovery by compensating for activity limitations and participation restrictions. MSkL is a fundamental ability that allows for the acquisition of unimanual or bimanual skills (i.e., writing, playing the piano) and adaptation of these skills to changing environments. It has been suggested that procedural learning, including MSkL, proceeds over three phases [12]: (i) an early “strategic/cognitive” phase, which presents rapid performance improvement, especially in the dorsolateral prefrontal cortex and posterior parietal cortex (PPC); (ii) a consolidation phase, which involves the stabilization of the learned skill based principally through a corticostriatal loop (striatum and supplementary motor area); and (iii) a retention phase, which is also called the “automatization phase”, during which the performance of the skill is optimized due to the increased activity in the primary motor cortex (M1), the premotor cortex and PPC [13]. Improvement of a skill is linked to practice-dependent training: the more we practice a skill, the better we perform it, with smoother movements and reduced variability [14, 15]. Sensorimotor skill acquisition (or MSkL) represents the ability to select and refine the movements needed to attain a goal in which the sensory stimuli for selecting and correcting our actions are considered and then the skill is executed consistently with both speed and accuracy (i.e., with motor acuity). Once learned, a motor skill can be retained for long periods of time, thus leading to lasting performance improvements, which is the aim of neurorehabilitation [16].
Robotic devices have long been expected to enhance recovery after a brain injury, such as a stroke [17,18,19,20], because they offer the possibility of providing intensive task-specific training to regulate task parameters, quantify and monitor improvements, and continuously adapt the task’s difficulty [21, 22]. E.g., Keeling and al. [20] showed that the use of the bimanual robotic tasks for rehabilitation in subacute stroke is feasible and suggested that the use of robotic devices added to standard of care therapy could augment recovery. Moreover, the proprioceptive feedback during active movements, delivered through a robotic therapy improved sensorimotor function in chronic stroke patients [23]. In fact, the proprioceptive training could enhance somatosensory and motor functions and induce cortical reorganization [24]. Interestingly, robotics has the potential to formally implement the principles of motor learning in neurorehabilitation. Cuppone et al. [25] found that somatosensory learning is linked to motor learning because these processes similar features of memory formation. Robotic devices can provide four main training modalities: (i) active mode (where the subject fully performs the task), (ii) active-assisted mode (where the robot provides assistance either at a fixed rate or “as needed”), (iii) passive mode (where the robot fully performs the task), and (iv) resistive mode (where the robot perturbs the subject’s attempts); these modalities allow for valuable interactions with patients [26]. In a meta-analysis, Kwakkel et al. showed significant improvement in UL impairment (but not in ADL) with robot-assisted training (RAT) [27]. However, a recent Cochrane review showed that RAT enhances both UL impairments and ADL in stroke survivors [18], and another team showed that RAT improved motor coordination compared to unilateral training in patients with stroke with severe impairments [28].
More recently, we used a custom system with computer mice and showed that patients with stroke were able to learn, retain and generalize a complex bimanual skill after a single session of real and sham transcranial direct current stimulation (tDCS) [29]. Determining whether patients with stroke could achieve bimanual MSkL (bim-MSkL) and identifying the underlying mechanisms and extent of learning are crucial for the development of efficient neurorehabilitation approaches targeting independence in (bimanual) ADL.
To explore how patients (re)learn to coordinate their hands after a stroke, we developed a complex asymmetrical bimanual coordination task (CIRCUIT) that was implemented as a serious game in the bimanual version of the REAplan® robot (AXINESIS, Wavre, Belgium). With a common cursor controlled by coordinated movements of the ULs interacting with robotic handles, one hand exclusively controlled lateral displacements of the common cursor while the other hand exclusively controlled the sagittal displacements. It has been suggested that stroke recovery studies should include quantitative measures, such as speed, accuracy, path length metrics and smoothness of movement [26]. By analyzing kinematic parameters and providing such real-time quantitative measures of movement, REAplan® can be used for training UL movements [30, 31]. Our hypotheses were that (i) patients in the chronic phase of stroke would show improvements in a new complex bimanual coordination skill and be able to retain and generalize this skill, i.e., they would be able to achieve complex bim-MSkL and/or improve on other bimanual or unimanual performances; (ii) patients would show similar improvements in bim-MSkL as healthy individuals; and (iii) poorer baseline clinical scales in patients would correlate with poorer bim-MSkL indices. […]

Bimanual tasks on the REAplan®. A General setup of the bimanual version of the REAplan® robot. Note that each hand slid exclusively along one axis and thus controlled a different direction of the common cursor (small arrowhead) displayed on the REAplan® screen. The forearms rested in gutters and were strapped in, and handles were adapted if needed. B Different circuit of identical length and difficulty for the generalization. C Cursor displacement with regard to the ideal trajectory defined as the center of the circuit track (surface = error), D REACHING task: four eccentric targets designated in a pseudorandomized order (16 trials/target)
[ARTICLE] Bimanual Motor Skill Learning With Robotics in Chronic Stroke – Full Text
Posted by Kostas Pantremenos in Paretic Hand, Rehabilitation robotics, Video Games/Exergames on December 7, 2021
Abstract
Background. Most activities of daily life (ADL) require cooperative bimanual movements. A unilateral stroke may severely impair bimanual ADL. How patients with stroke (re)learn to coordinate their upper limbs (ULs) is largely unknown.
The objectives are to determine whether patients with chronic supratentorial stroke could achieve bimanual motor skill learning (bim-MSkL). To compare bim-MSkL between patients and healthy individuals (HIs).
Methods. Twenty-four patients and ten HIs trained over 3 consecutive days on an asymmetrical bimanual coordination task (CIRCUIT) implemented as a serious game in the REAplan® robot. With a common cursor controlled by coordinated movements of the ULs through robotic handles, they performed as many laps as possible (speed constraint) on the CIRCUIT while keeping the cursor within the track (accuracy constraint). The primary outcome was a bimanual speed/accuracy trade-off (biSAT), we used a bimanual coordination factor (biCO) and bimanual forces (biFOP) for the secondary outcomes. Several clinical scales were used to evaluate motor and cognitive functions.
Results. Overall, the patients showed improvements on biSAT and biCO. Based on biSAT progression, the HI achieved a larger bim-MSkL than the patients with mild to moderate impairment (Fugl-Meyer Assessment Upper Extremity (FMA-UE): 28-55, n=15) but not significantly different from those with minimal motor impairment (FMA-UE: 66, n=9). There was a significant positive correlation between biSAT evolution and the FMA-UE and Stroke Impact Scale.
Conclusions. Both HI and patients with chronic stroke training on a robotic device achieved bim-MSkL, although the more impaired patients were less efficient. Bim-MSkL with REAplan® may be interesting for neurorehabilitation after stroke.
Background
Stroke is a major cause of long-term disability worldwide (1, 2), and one of the most frequent impairments is hemiparesis, which is characterized by weakness, lack of control, increased muscle tone on the contralesional upper limb (UL) and lower limb or hemibody, and deteriorating independence in activities of daily life (ADL), especially walking, dressing or eating (3). Critically, other impairments (e.g., somatosensory, visual, and cognitive), whether isolated or combined, also significantly deteriorate ADL. Most ADL require skilled bimanual coordination that can be impaired by a stroke, thus leading to a loss of independence that may in turn lead to a 50% reduction in quality of life (4). Despite rehabilitative care provided during the acute phase of stroke, 30% of patients still suffer from participation restrictions after four years (5). It has been suggested that neurorehabilitation should not focus exclusively on impairments of the paretic arm or hand and should instead consider more bimanual actions and activities (6-8). After a unilateral stroke, impairments of the contralesional UL can deteriorate bimanual actions (9), thus supporting the importance of training both ULs to achieve better functional recovery in (bimanual) ADL (8, 10). Interestingly, during bilateral cooperative movements (e.g., opening a bottle), neural coupling from the ipsilesional to the contralesional (impaired) UL is preserved in most patients with stroke, suggesting the relevance of bilateral training that supports cooperative hand movements for ADL (11). During bimanual training, various tasks can be utilized to promote intensive and repetitive coordinated movement of the ULs. A classification of bimanual tasks has been proposed for different bimanual actions. Grossly, two types of tasks can be distinguished: those with symmetrical movements that engage homologous muscles (e.g., picking up a box simultaneously with both hands) and those with asymmetrical movements that engage nonhomologous muscles nonsimultaneously (e.g., cutting a piece of steak). Similarly, two types of task goals can be distinguished: independent goals (e.g., one hand lifting a cup and the other hand lifting a glass simultaneously) versus common goals (e.g., both hands working together to accomplish a common task) (8). Many bilateral actions, such as arms swinging during bipedal locomotion, seem to depend on “default-mode” neural coupling. However, in most skilled ADL, bimanual actions are accomplished through asymmetrical movements that cooperate to achieve a common goal, e.g., buttoning a skirt or changing the gear while steering a car. Such complex bimanual, cooperative, asymmetrical skills have to be learned.
After a stroke, motor skill learning (MSkL) plays a key role in recovery by compensating for activity limitations and participation restrictions. MSkL is a fundamental ability that allows for the acquisition of unimanual or bimanual skills (i.e., writing, playing the piano) and adaptation of these skills to changing environments. It has been suggested that procedural learning, including MSkL, proceeds over three phases (12): (i) an early “strategic/cognitive” phase, which presents rapid performance improvement, especially in the dorsolateral prefrontal cortex and posterior parietal cortex (PPC); (ii) a consolidation phase, which involves the stabilization of the learned skill based principally through a corticostriatal loop (striatum and supplementary motor area); and (iii) a retention phase, which is also called the “automatization phase”, during which the performance of the skill is optimized due to the increased activity in the primary motor cortex (M1), the premotor cortex and PPC (13). Improvement of a skill is linked to practice-dependent training: the more we practice a skill, the better we perform it, with smoother movements and reduced variability (14, 15). Sensorimotor skill acquisition (or MSkL) represents the ability to select and refine the movements needed to attain a goal in which the sensory stimuli for selecting and correcting our actions are considered and then the skill is executed consistently with both speed and accuracy (i.e., with motor acuity). Once learned, a motor skill can be retained for long periods of time, thus leading to lasting performance improvements, which is the aim of neurorehabilitation (16).
Robotic devices have long been expected to enhance recovery after a brain injury, such as a stroke (17-19), because they offer the possibility of providing intensive task-specific training to regulate task parameters, quantify and monitor improvements, and continuously adapt the task’s difficulty (20, 21). Interestingly, robotics has the potential to implement the principles of motor learning in neurorehabilitation. Robotic devices can provide four main training modalities: (i) active mode (where the subject fully performs the task), (ii) active-assisted mode (where the robot provides assistance either at a fixed rate or “as needed”), (iii) passive mode (where the robot fully performs the task), and (iv) resistive mode (where the robot perturbs the subject’s attempts); these modalities allow for valuable interactions with patients (22). In a meta-analysis, Kwakkel et al. showed significant improvement in UL impairment (but not in ADL) with robot-assisted training (RAT) (23). However, a recent Cochrane review showed that RAT enhances both UL impairments and ADL in stroke survivors (18), and another team showed that RAT improved motor coordination compared to unilateral training in patients with stroke with severe impairments (24).
More recently, we used a custom system with computer mice and showed that patients with stroke were able to learn, retain and generalize a complex bimanual skill after a single session of real and sham transcranial direct current stimulation (tDCS) (25). Determining whether patients with stroke could achieve bimanual MSkL (bim-MSkL) and identifying the underlying mechanisms and extent of learning are crucial for the development of efficient neurorehabilitation approaches targeting independence in (bimanual) ADL.
To explore how patients (re)learn to coordinate their hands after a stroke, we developed a complex asymmetrical bimanual coordination task (CIRCUIT) that was implemented as a serious game in the bimanual version of the REAplan® robot (AXINESIS, Wavre, Belgium). With a common cursor controlled by coordinated movements of the ULs interacting with robotic handles, one hand exclusively controlled lateral displacements of the common cursor while the other hand exclusively controlled the sagittal displacements. It has been suggested that stroke recovery studies should include quantitative measures, such as speed, accuracy, path length metrics and smoothness of movement (Carpinella et al., 2020). By analyzing kinematic parameters and providing such real-time quantitative measures of movement, REAplan® can be used for training UL movements (26, 27). Our hypotheses were that (i) patients in the chronic phase of stroke would show improvements in a new complex bimanual coordination skill and be able to retain and generalize this skill, i.e., they would be able to achieve complex bim-MSkL; (ii) patients would show similar improvements in bim-MSkL as healthy individuals; and (iii) poorer baseline clinical scales in patients would correlate with poorer bim-MSkL indices.[…]

[ARTICLE] Study Paradigms and Principles Investigated in Motor Learning Research After Stroke: A Scoping Review – Full Text
Posted by Kostas Pantremenos in Neuroplasticity, REHABILITATION on October 17, 2021
Abstract
Objectives
To (1) characterize study paradigms used to investigate motor learning (ML) poststroke and (2) summarize the effects of different ML principles in promoting skill acquisition and retention. Our secondary objective is to evaluate the clinical utility of ML principles on stroke rehabilitation.
Data Sources
Medline, Excerpta Medica Database, Allied and Complementary Medicine, Cumulative Index to Nursing and Allied Health Literature, and Cochrane Central Register of Controlled Trials were searched from inception on October 24, 2018 and repeated on June 23, 2020. Scopus was searched on January 24, 2019 and July 22, 2020 to identify additional studies.
Study Selection
Our search included keywords and concepts to represent stroke and “motor learning. An iterative process was used to generate study selection criteria. Three authors independently completed title, abstract, and full-text screening.
Data Extraction
Three reviewers independently completed data extraction.
Data Synthesis
The Preferred Reporting Items for Systematic Reviews and Meta-Analyses extension guidelines for scoping reviews were used to guide our synthesis. Thirty-nine studies were included. Study designs were heterogeneous, including variability in tasks practiced, acquisition parameters, and retention intervals. ML principles investigated included practice complexity, feedback, motor imagery, mental practice, action observation, implicit and explicit information, aerobic exercise, and neurostimulation. An additional 2 patient-related factors that influence ML were included: stroke characteristics and sleep. Practice complexity, feedback, and mental practice/action observation most consistently promoted ML, while provision of explicit information and more severe strokes were detrimental to ML. Other factors (ie, sleep, practice structure, aerobic exercise, neurostimulation) had a less clear influence on learning.
Conclusions
Improved consistency of reporting in ML studies is needed to improve study comparability and facilitate meta-analyses to better understand the influence of ML principles on learning poststroke. Knowledge of ML principles and patient-related factors that influence ML, with clinical judgment can guide neurologic rehabilitation delivery to improve patient motor outcomes.
——————————————–
One of the most debilitating deficits poststroke is motor impairment.1,2 To address this, rehabilitation therapists help individuals practice movements, enabling them to relearn functions affected by their stroke.3,4 It is important for the individual’s recovery and function that this “relearning” leads to a permanent change in motor behavior. For example, clinicians may facilitate a better sit-to-stand during therapy, yet the ultimate goal is for the person to stand up independently in their daily life. This rehabilitation process exemplifies motor learning (ML) that is defined as a group of internal processes associated with practice that leads to a relatively permanent change in the capacity for skilled movement.5 ML is a proposed model for stroke rehabilitation.6
Since 2010, a surge of randomized controlled trials has evaluated the effectiveness of different interventions for stroke recovery,7 leading to best practice guidelines recommending the use of specific therapies (eg, virtual reality, task-specific practice) to improve motor recovery.8 Although there is strong support for these interventions,8 it remains unclear if there are optimal conditions in which they can be implemented to maximize effectiveness. Consideration of not only the type of therapy but also the conditions under which the therapy is delivered has the potential to improve motor recovery poststroke. These conditions can be referred to as ML principles.
We define ML principles as conditions during task practice that influence learning and can be applied by rehabilitation clinicians; they are not targeted for a specific movement or therapeutic approach/intervention. As principles of ML can affect learning, they should be used to guide the implementation of neurorehabilitation to promote long-term recovery. The influence of these principles on learning can be scientifically evaluated using ML paradigms. ML paradigms typically have 3 distinct phases: acquisition session(s) when individuals practice a motor skill, retention session(s) where motor skill performance is evaluated after a period of no practice, and transfer testing whereby one tests whether improvements in the practiced skill translates to another similar skill or the same skill under different conditions. Conditions of acquisition sessions (ie, ML principles) influence the trajectory, magnitude, and permanence of learning (assessed by retention testing)4,9 and have physiological effects through neuroplasticity and neural recovery.10 Furthermore, ML principles that promote motor acquisition (eg, blocked practice) may be different than features that are beneficial for motor retention (eg, random practice).4,11
The methods used to investigate ML can affect the interpretation of study results which in turn influences clinical application. However, knowledge about the methods or paradigms used to investigate ML in stroke is limited. A detailed understanding of ML study protocols is necessary to inform stroke rehabilitation. In addition, although previous papers have summarized ML findings poststroke,4,12,13 and Maier et al14 have recently published a conceptual analysis demonstrating how some ML principles may influence neurorehabilitation efforts, there has been no systematic or comprehensive synthesis of the ML literature involving persons poststroke. For clinicians to be able to use the evidence to inform their practice, they need to be able to easily identify and compare all factors that can influence learning. Therefore, the primary objectives of our study are to (1) summarize and describe the approaches and methods used to investigate ML poststroke and (2) summarize the effects of different ML principles in promoting skill acquisition and retention in individuals’ poststroke. To facilitate the translation of ML research into clinical practice, our secondary objective was to evaluate the clinical utility15 of each ML principle for stroke rehabilitation.
Methods
The methodology for this review is based on the recommendations of Levac et al16 and the Preferred Reporting Items for Systematic Reviews and Meta-Analyses extension guidelines for scoping reviews.17 Three authors (S.G., T.S., L.C.) performed article screening, data extraction, quality assessment, and determined ease of clinical application. Discrepancies were resolved through discussion, and a fourth author (K.P.) was consulted if needed.
Data sources
We searched 5 databases for studies investigating ML in individuals poststroke on October 24, 2018 and again on June 23, 2020 from inception: Medline (1946 to present), Excerpta Medica Database (1947 to June 22, 2020), Allied and Complementary Medicine (1985 to June 2020), Cumulative Index to Nurisng and Allied Health Litrerature (1981 to present), and the Cochrane Central Register of Controlled Trials (2014 to present). Keywords and Medical Subjects Headings terms for the concepts of “stroke” and “motor learning” were searched using the AND operator, with human and English filters applied. The initial search strategy was created for Medline (table 1) in collaboration with an information specialist and translated for the other databases. A second information specialist evaluated the initial and translated searches and made suggestions to improve precision and sensitivity.18 […]
[ARTICLE] Effects of Transcranial Direct Current Stimulation Combined With Physical Training on the Excitability of the Motor Cortex, Physical Performance, and Motor Learning: A Systematic Review – Full Text
Posted by Kostas Pantremenos in tDCS/rTMS on September 23, 2021
Purpose: This systematic review aims to examine the efficacy of transcranial direct current stimulation (tDCS) combined with physical training on the excitability of the motor cortex, physical performance, and motor learning.
Methods: A systematic search was performed on PubMed, Web of Science, and EBSCO databases for relevant research published from inception to August 2020. Eligible studies included those that used a randomized controlled design and reported the effects of tDCS combined with physical training to improve motor-evoked potential (MEP), dynamic posture stability index (DPSI), reaction time, and error rate on participants without nervous system diseases. The risk of bias was assessed by the Cochrane risk of bias assessment tool.
Results: Twenty-four of an initial yield of 768 studies met the eligibility criteria. The risk of bias was considered low. Results showed that anodal tDCS combined with physical training can significantly increase MEP amplitude, decrease DPSI, increase muscle strength, and decrease reaction time and error rate in motor learning tasks. Moreover, the gain effect is significantly greater than sham tDCS combined with physical training.
Conclusion: tDCS combined with physical training can effectively improve the excitability of the motor cortex, physical performance, and motor learning. The reported results encourage further research to understand further the synergistic effects of tDCS combined with physical training.
Introduction
Transcranial direct current stimulation (tDCS) is a non-invasive brain stimulation technique that modulates the neural activities of cortical brain regions by applying a constant weak current (e.g., current intensity of one electrode is usually smaller than 2 mA) via the scalp electrodes (Nitsche and Paulus, 2000). More and more research using tDCS has emerged in the fields of sports and rehabilitative medicine these days (Chang et al., 2017; Xiao et al., 2020). Two types of tDCS are commonly used, that is, anodal tDCS (a-tDCS) aiming to increase the excitability of the targeting cortical regions by depolarizing the resting membrane potentials of neurons, and cathodal tDCS (c-tDCS) that often induces inhibitory effects of neural excitability in the targeted brain regions (Nitsche and Paulus, 2000, 2001; Bastani and Jaberzadeh, 2012). Recent studies in the field of sports sciences have shown that using tDCS can significantly enhance physical performance, such as the toe abduction strength (Tanaka et al., 2009) and reaction time (Tseng et al., 2020) in healthy people and the knee extensor force in patients with hemiparetic stroke. Several previous systematic reviews have also confirmed that using tDCS can induce benefits to important functionalities, such as motor control, in different populations (e.g., people with Parkinson’s disease (PD) (Broeder et al., 2015) and healthy cohorts (Machado et al., 2019).
More recently, researchers have started to combine tDCS with other types of interventions, such as physical (e.g., exercise, physiotherapy, etc.) and cognitive training (Beretta et al., 2020), and explore the effects of this mixed type of intervention. Studies have shown that both tDCS and exercise (e.g., strength training) can increase the excitability of cortical (e.g., primary motor cortex, M1) as measured by the amplitude of motor-evoked potential (MEP) (Kidgell et al., 2010; Mazzoleni et al., 2019). Therefore, it is speculated that this mixed-type intervention may induce greater improvements as compared to tDCS-or exercise/training-only intervention. However, a large variance in the results of the studies exploring the effects of such mixed-type intervention was observed in previous studies. For example, Kim and Ko (2013) observed that tDCS combined with grip strength training induced a greater increase in MEP amplitude as compared to tDCS applied alone, and Jafarzadeh et al. (2019) also observed that tDCS combined with physical training induced greater improvement in dynamic stability. However, Zandvliet et al. (2018) reported that in healthy people, tDCS combined with posture training did not induce significant improvement in the center of pressure (CoP) parameters when standing quietly with eyes open or closed, including the mean and variability of the amplitude of the CoP displacement and the mean and variability of the velocity of CoP fluctuation.
Therefore, the effects of this mixed-type intervention consisting of tDCS with physical training remain unclear due to the inconsistent results of previous publications. This systematic review here thus aims to examine the efficacy of tDCS combined with physical training on the excitability of M1, physical performance and motor learning function in populations without any major neurological diseases by critically evaluating and comparing the results in the publications, which will ultimately provide important knowledge to this field and informing the design of future studies.[…]