Posts Tagged machine learning
[ARTICLE] Utilizing the intelligence edge framework for robotic upper limb rehabilitation in home – Full Text
Posted by Kostas Pantremenos in Paretic Hand, Rehabilitation robotics, Tele/Home Rehabilitation on February 4, 2024
Abstract
Robotic devices are gaining popularity for the physical rehabilitation of stroke survivors. Transition of these robotic systems from research labs to the clinical setting has been successful, however, providing robot-assisted rehabilitation in home settings remains to be achieved. In addition to ensure safety to the users, other important issues that need to be addressed are the real time monitoring of the installed instruments, remote supervision by a therapist, optimal data transmission and processing. The goal of this paper is to advance the current state of robot-assisted in-home rehabilitation. A state-of-the-art approach to implement a novel paradigm for home-based training of stroke survivors in the context of an upper limb rehabilitation robot system is presented in this paper. First, a cost effective and easy-to-wear upper limb robotic orthosis for home settings is introduced. Then, a framework of the internet of robotics things (IoRT) is discussed together with its implementation. Experimental results are included from a proof-of-concept study demonstrating that the means of absolute errors in predicting wrist, elbow and shoulder angles are 0.89180,2.67530 and 8.02580, respectively. These experimental results demonstrate the feasibility of a safe home-based training paradigm for stroke survivors. The proposed framework will help overcome the technological barriers, being relevant for IT experts in health-related domains and pave the way to setting up a telerehabilitation system increasing implementation of home-based robotic rehabilitation. The proposed novel framework includes:
- •A low-cost and easy to wear upper limb robotic orthosis which is suitable for use at home.
- •A paradigm of IoRT which is used in conjunction with the robotic orthosis for home-based rehabilitation.
- •A machine learning-based protocol which combines and analyse the data from robot sensors for efficient and quick decision making.
Graphical abstract


[ARTICLE] Unsupervised robot-assisted rehabilitation after stroke: feasibility, effect on therapy dose, and user experience
Posted by Kostas Pantremenos in Paretic Hand, REHABILITATION, Rehabilitation robotics on January 7, 2024
Abstract
Background
Unsupervised robot-assisted rehabilitation is a promising approach to increase the dose of therapy after stroke, which may help promote sensorimotor recovery without requiring significant additional resources and manpower. However, the unsupervised use of robotic technologies is not yet a standard, as rehabilitation robots often show low usability or are considered unsafe to be used by patients independently. In this paper we explore the feasibility of unsupervised therapy with an upper limb rehabilitation robot in a clinical setting, evaluate the effect on the overall therapy dose, and assess user experience during unsupervised use of the robot and its usability.
Methods
Subacute stroke patients underwent a four-week protocol composed of daily 45 minutes-sessions of robot-assisted therapy. The first week consisted of supervised therapy, where a therapist explained how to interact with the device. The second week was minimally supervised, i.e., the therapist was present but intervened only if needed. After this phase, if participants learnt how to use the device, they proceeded to two weeks of fully unsupervised training. Feasibility, dose of robot-assisted therapy achieved during unsupervised use, user experience, and usability of the device were the primary outcome measures. Questionnaires to evaluate usability and user experience were performed after the minimally supervised week and at the end of the study, to evaluate the impact of therapists’ absence.
Results
Unsupervised robot-assisted therapy was found to be feasible, as 12 out of the 13 recruited participants could progress to unsupervised training. During the two weeks of unsupervised therapy participants on average performed an additional 360 minutes of robot-assisted rehabilitation. Participants were satisfied with the device usability (mean System Usability Scale scores > 79), and no adverse events or device deficiencies occurred.
Conclusions
We demonstrated that unsupervised robot-assisted therapy in a clinical setting with an actuated device for the upper limb was feasible and can lead to a meaningful increase in therapy dose.

Participant performing a therapy exercise with the ReHapticKnob. To train with the device, participants need to fix their fingers to the handles with Velcro straps and log in to their therapy account with the fingerprint reader. A pushbutton keyboard is used to interact with the device and the virtual environment displayed on the screen. The view of the hand (visual feedback) is blocked by the hand cover, as the exercises require users to focus on the sensory feedback from the affected hand to solve the different tasks.
[Abstract + References] A new adaptive VR-based exergame for hand rehabilitation after stroke
Posted by Kostas Pantremenos in Paretic Hand, REHABILITATION, Video Games/Exergames, Virtual reality rehabilitation on October 18, 2023
Abstract
The aim of this work is to present an adaptive serious game based on virtual reality (VR) for functional rehabilitation of the hand after stroke. The game focuses on simulating the palmar grasping exercise commonly used in clinical settings. The system’s design follows a user-centered approach, involving close collaboration with functional rehabilitation specialists and stroke patients. It uses the Leap motion controller to enable patient interaction in the virtual environment, which was created using the Unity 3D game engine. The system relies on hand gestures involving opening and closing movements to interact with virtual objects. It incorporates parameters to objectively measure participants’ performance throughout the game session. These metrics are used to personalize the game’s difficulty to each patient’s motor skills. To do this, we implemented an approach that dynamically adjusts the difficulty of the exergame according to the patient’s performance during the game session. To achieve this, we used an unsupervised machine learning technique known as clustering, in particular using the K-means algorithm. By applying this technique, we were able to classify patients’ performance into distinct groups, enabling us to assess their skill level and adapt the difficulty of the game accordingly. To evaluate the system’s effectiveness and reliability, we conducted a subjective evaluation involving 11 stroke patients. The standardized System Usability Scale (SUS) questionnaire was used to assess the system’s ease of use, while the Intrinsic Motivation Inventory (IMI) was used to evaluate the participants’ subjective experience with the system. Evaluations showed that our proposed system is usable and acceptable on a C-level scale, with a good adjective score, and the patients perceived a high intrinsic motivation.
References
- Montoya, M.F., Munoz, J.E., Henao, O.A.: Enhancing virtual rehabilitation in upper limbs with biocybernetic adaptation: the effects of virtual reality on perceived muscle fatigue, game performance and user experience. IEEE Trans. Neural Syst. Rehabil. Eng. 28, 740–747 (2020). https://doi.org/10.1109/TNSRE.2020.2968869Article Google Scholar
- Then, J.W., Shivdas, S., Tunku Ahmad Yahaya, T.S., Ab Razak, N.I., Choo, P.T.: Gamification in rehabilitation of metacarpal fracture using cost-effective end-user device: a randomized controlled trial. J. Hand Therap. 33, 235–242 (2020). https://doi.org/10.1016/j.jht.2020.03.029
- Goršič, M., Cikajlo, I., Novak, D.: Competitive and cooperative arm rehabilitation games played by a patient and unimpaired person: effects on motivation and exercise intensity. J NeuroEng. Rehabil. 14, 23 (2017). https://doi.org/10.1186/s12984-017-0231-4Article Google Scholar
- Alarcón-Aldana, A.C., Callejas-Cuervo, M., Bo, A.P.L.: Upper limb physical rehabilitation using serious videogames and motion capture systems: a systematic review. Sensors. 20, 5989 (2020). https://doi.org/10.3390/s20215989Article Google Scholar
- Bortone, I., Leonardis, D., Solazzi, M., Procopio, C., Crecchi, A., Bonfiglio, L., Frisoli, A.: Integration of serious games and wearable haptic interfaces for Neuro Rehabilitation of children with movement disorders: A feasibility study. In: 2017 International Conference on Rehabilitation Robotics (ICORR). pp. 1094–1099. IEEE, London (2017)
- Avola, D., Cinque, L., Foresti, G.L., Marini, M.R.: An interactive and low-cost full body rehabilitation framework based on 3D immersive serious games. J. Biomed. Inform. 89, 81–100 (2019). https://doi.org/10.1016/j.jbi.2018.11.012Article Google Scholar
- Ribé, J.T., Serrancolí, G., Arriaga, I.A., Alpiste, F., Iriarte, A., Margelí, C., Mayans, B., García, A., Morancho, S., Molas, C.: Design and Development of Biomedical Applications for Post-Stroke Rehabilitation Subjects
- Ferreira, B., Menezes, P.: An Adaptive Virtual Reality-Based Serious Game for Therapeutic Rehabilitation. Int. J. Onl. Eng. 16, 63 (2020). https://doi.org/10.3991/ijoe.v16i04.11923Article Google Scholar
- Pillai, M., Yang, Y., Ditmars, C., Subhash, H.M.: Artificial intelligence-based interactive virtual reality-assisted gaming system for hand rehabilitation. In: Deserno, T.M. and Chen, P.-H. (eds.) Medical Imaging 2020: Imaging Informatics for Healthcare, Research, and Applications. p. 18. SPIE, Houston, United States (2020)
- Piette, P., Pasquier, J.: Réalité virtuelle et rééducation. Kinésithérapie, la Revue. 12, 38–41 (2012). https://doi.org/10.1016/j.kine.2012.07.003Article Google Scholar
- Kim, W.-S., Cho, S., Ku, J., Kim, Y., Lee, K., Hwang, H.-J., Paik, N.-J.: Clinical Application of Virtual Reality for Upper Limb Motor Rehabilitation in Stroke: Review of Technologies and Clinical Evidence. JCM. 9, 3369 (2020). https://doi.org/10.3390/jcm9103369Article Google Scholar
- Ning, H., Wang, Z., Li, R., Zhang, Y., Mao, L.: A Review on Serious Games for Exercise Rehabilitation. arXiv:2201.04984 [cs]. (2022)
- Amorim, P., Sousa Santos, B., Dias, P., Silva, S., Martins, H.: Serious Games for Stroke Telerehabilitation of Upper Limb – A Review for Future Research. Int J Telerehab. 12, 65–76 (2020). https://doi.org/10.5195/ijt.2020.6326Article Google Scholar
- Lawrence, E.S., Coshall, C., Dundas, R., Stewart, J., Rudd, A.G., Howard, R., Wolfe, C.D.A.: Estimates of the Prevalence of Acute Stroke Impairments and Disability in a Multiethnic Population. Stroke 32, 1279–1284 (2001). https://doi.org/10.1161/01.STR.32.6.1279Article Google Scholar
- Avola, D., Cinque, L., Pannone, D.: Design of a 3D Platform for Immersive Neurocognitive Rehabilitation. Information 11, 134 (2020). https://doi.org/10.3390/info11030134Article Google Scholar
- Zahabi, M., Abdul Razak, A.M.: Adaptive virtual reality-based training: a systematic literature review and framework. Virtual Reality 24, 725–752 (2020). https://doi.org/10.1007/s10055-020-00434-wArticle Google Scholar
- Debnath, B., O’Brien, M., Yamaguchi, M., Behera, A.: A review of computer vision-based approaches for physical rehabilitation and assessment. Multimedia Syst. 28, 209–239 (2022). https://doi.org/10.1007/s00530-021-00815-4Article Google Scholar
- Rincon, A.L., Yamasaki, H., Shimoda, S.: Design of a video game for rehabilitation using motion capture, EMG analysis and virtual reality. In: 2016 International Conference on Electronics. Communications and Computers (CONIELECOMP), pp. 198–204. IEEE, Cholula, Mexico (2016)
- Trombetta, M., Bazzanello Henrique, P.P., Brum, M.R., Colussi, E.L., De Marchi, A.C.B., Rieder, R.: Motion Rehab AVE 3D: A VR-based exergame for post-stroke rehabilitation. Computer Methods and Programs in Biomedicine. 151, 15-20 (2017). https://doi.org/10.1016/j.cmpb.2017.08.008
- Hashim, S.H.M., Ismail, M., Manaf, H., Hanapiah, F.A.: Development of Dual Cognitive Task Virtual Reality Game Addressing Stroke Rehabilitation. In: Proceedings of the 2019 3rd International Conference on Virtual and Augmented Reality Simulations. pp. 21-25. ACM, Perth WN Australia (2019
- Ma, M., Zheng, H.: Virtual Reality and Serious Games in Healthcare. In: Brahnam, S. and Jain, L.C. (eds.) Advanced Computational Intelligence Paradigms in Healthcare 6. Virtual Reality in Psychotherapy, Rehabilitation, and Assessment. pp. 169-192. Springer Berlin Heidelberg, Berlin, Heidelberg (2011)
- Gonçalves, A.R., Muñoz, J.E., Gouveia, É.R., Cameirão, M. da S., Bermúdez i Badia, S.: Effects of prolonged multidimensional fitness training with exergames on the physical exertion levels of older adults. Vis Comput. 37, 19-30 (2021). https://doi.org/10.1007/s00371-019-01736-0
- Hossain, M.S., Hardy, S., Alamri, A., Alelaiwi, A., Hardy, V., Wilhelm, C.: AR-based serious game framework for post-stroke rehabilitation. Multimedia Syst. 22, 659–674 (2016). https://doi.org/10.1007/s00530-015-0481-6Article Google Scholar
- Streicher, A., Smeddinck, J.D.: Personalized and Adaptive Serious Games. In: Dörner, R., Göbel, S., Kickmeier-Rust, M., Masuch, M., Zweig, K. (eds.) Entertainment Computing and Serious Games, pp. 332–377. Springer International Publishing, Cham (2016)Chapter Google Scholar
- Hocine, N., Gouaïch, A., Cerri, S.A., Mottet, D., Froger, J., Laffont, I.: Adaptation in serious games for upper-limb rehabilitation: an approach to improve training outcomes. User Model. User-Adap. Inter. 25, 65–98 (2015). https://doi.org/10.1007/s11257-015-9154-6Article Google Scholar
- Thielbar, K.O., Lord, T.J., Fischer, H.C., Lazzaro, E.C., Barth, K.C., Stoykov, M.E., Triandafilou, K.M., Kamper, D.G.: Training finger individuation with a mechatronic-virtual reality system leads to improved fine motor control post-stroke. J NeuroEngineering Rehabil. 11, 171 (2014). https://doi.org/10.1186/1743-0003-11-171Article Google Scholar
- Sinaga, K.P., Yang, M.-S.: Unsupervised K-Means Clustering Algorithm. IEEE. Access 8, 80716–80727 (2020). https://doi.org/10.1109/ACCESS.2020.2988796Article Google Scholar
- Colombo, R., Pisano, F., Micera, S., Mazzone, A., Delconte, C., Carrozza, M.C., Dario, P., Minuco, G.: Robotic techniques for upper limb evaluation and rehabilitation of stroke patients. IEEE Trans. Neural Syst. Rehabil. Eng. 13, 311–324 (2005). https://doi.org/10.1109/TNSRE.2005.848352Article Google Scholar
- Sunderland, A., Tinson, D., Bradley, L., Hewer, R.L.: Arm function after stroke. An evaluation of grip strength as a measure of recovery and a prognostic indicator. Journal of Neurology, Neurosurgery & Psychiatry. 52, 1267-1272 (1989). https://doi.org/10.1136/jnnp.52.11.1267
- Kim, H., Miller, L.M., Fedulow, I., Simkins, M., Abrams, G.M., Byl, N., Rosen, J.: Kinematic Data Analysis for Post-Stroke Patients Following Bilateral Versus Unilateral Rehabilitation With an Upper Limb Wearable Robotic System. IEEE Trans. Neural Syst. Rehabil. Eng. 21, 153–164 (2013). https://doi.org/10.1109/TNSRE.2012.2207462Article Google Scholar
- Kong, K.-H., Lee, J.: Temporal recovery and predictors of upper limb dexterity in the first year of stroke: A prospective study of patients admitted to a rehabilitation centre. NRE. 32, 345–350 (2013). https://doi.org/10.3233/NRE-130854Article Google Scholar
- Bigoni, M., Baudo, S., Cimolin, V., Cau, N., Galli, M., Pianta, L., Tacchini, E., Capodaglio, P., Mauro, A.: Does kinematics add meaningful information to clinical assessment in post-stroke upper limb rehabilitation? A case report. J Phys Ther Sci. 28, 2408–2413 (2016). https://doi.org/10.1589/jpts.28.2408
- Sucar, L.E., Luis, R., Leder, R., Hernáindez, J., Sáinchez, I.: Gesture therapy: A vision-based system for upper extremity stroke rehabilitation. In: 2010 Annual International Conference of the IEEE Engineering in Medicine and Biology. pp. 3690-3693. IEEE, Buenos Aires (2010)
- Housman, S.J., Scott, K.M., Reinkensmeyer, D.J.: A Randomized Controlled Trial of Gravity-Supported, Computer-Enhanced Arm Exercise for Individuals With Severe Hemiparesis. Neurorehabil. Neural Repair 23, 505–514 (2009). https://doi.org/10.1177/1545968308331148Article Google Scholar
- Kong, K.-H., Loh, Y.-J., Thia, E., Chai, A., Ng, C.-Y., Soh, Y.-M., Toh, S., Tjan, S.-Y.: Efficacy of a Virtual Reality Commercial Gaming Device in Upper Limb Recovery after Stroke: A Randomized. Controlled Study. Topics in Stroke Rehabilitation. 23, 333–340 (2016). https://doi.org/10.1080/10749357.2016.1139796Article Google Scholar
- Rand, D., Givon, N., Weingarden, H., Nota, A., Zeilig, G.: Eliciting Upper Extremity Purposeful Movements Using Video Games: A Comparison With Traditional Therapy for Stroke Rehabilitation. Neurorehabil. Neural Repair 28, 733–739 (2014). https://doi.org/10.1177/1545968314521008Article Google Scholar
- Broeren, J., Claesson, L., Goude, D., Rydmark, M., Sunnerhagen, K.S.: Virtual Rehabilitation in an Activity Centre for Community-Dwelling Persons with Stroke. Cerebrovasc. Dis. 26, 289–296 (2008). https://doi.org/10.1159/000149576Article Google Scholar
- Sin, H., Lee, G.: Additional Virtual Reality Training Using Xbox Kinect in Stroke Survivors with Hemiplegia. American Journal of Physical Medicine & Rehabilitation. 92, 871–880 (2013). https://doi.org/10.1097/PHM.0b013e3182a38e40Article Google Scholar
- Kwon, J.-S., Park, M.-J., Yoon, I.-J., Park, S.-H.: Effects of virtual reality on upper extremity function and activities of daily living performance in acute stroke: A double-blind randomized clinical trial. NRE. 31, 379–385 (2012). https://doi.org/10.3233/NRE-2012-00807Article Google Scholar
- Lee, S., Kim, Y., Lee, B.-H.: Effect of Virtual Reality-based Bilateral Upper Extremity Training on Upper Extremity Function after Stroke: A Randomized Controlled Clinical Trial: Bilateral Upper Extremity Training in Post Stroke. Occup. Ther. Int. 23, 357–368 (2016). https://doi.org/10.1002/oti.1437Article Google Scholar
- Subramanian, S.K., Lourenço, C.B., Chilingaryan, G., Sveistrup, H., Levin, M.F.: Arm Motor Recovery Using a Virtual Reality Intervention in Chronic Stroke: Randomized Control Trial. Neurorehabil. Neural Repair 27, 13–23 (2013). https://doi.org/10.1177/1545968312449695Article Google Scholar
- Zheng, C., Liao, W., Xia, W.: Effect of combined low-frequency repetitive transcranial magnetic stimulation and virtual reality training on upper limb function in subacute stroke: a double-blind randomized controlled trail. J. Huazhong Univ. Sci. Technol. [Med. Sci.]. 35, 248-254 (2015). https://doi.org/10.1007/s11596-015-1419-0
- Piron, L., Turolla, A., Agostini, M., Zucconi, C., Cortese, F., Zampolini, M., Zannini, M., Dam, M., Ventura, L., Battauz, M., Tonin, P.: Exercises for paretic upper limb after stroke: A combined virtual-reality and telemedicine approach. J. Rehabil. Med. 41, 1016–102 (2009). https://doi.org/10.2340/16501977-0459Article Google Scholar
- Brunner, I., Skouen, J.S., Hofstad, H., Aßmus, J., Becker, F., Sanders, A.-M., Pallesen, H., Qvist Kristensen, L., Michielsen, M., Thijs, L., Verheyden, G.: Virtual Reality Training for Upper Extremity in Subacute Stroke (VIRTUES): A multicenter RCT. Neurology 89, 2413–2421 (2017). https://doi.org/10.1212/WNL.0000000000004744Article Google Scholar
- Choi, Y.-H., Ku, J., Lim, H., Kim, Y.H., Paik, N.-J.: Mobile game-based virtual reality rehabilitation program for upper limb dysfunction after ischemic stroke. RNN. 34, 455–463 (2016). https://doi.org/10.3233/RNN-150626Article Google Scholar
- Kiper, P., Agostini, M., Luque-Moreno, C., Tonin, P., Turolla, A.: Reinforced Feedback in Virtual Environment for Rehabilitation of Upper Extremity Dysfunction after Stroke: Preliminary Data from a Randomized Controlled Trial. Biomed. Res. Int. 2014, 1–8 (2014). https://doi.org/10.1155/2014/752128Article Google Scholar
- Ma, M., Bechkoum, K.: Serious games for movement therapy after stroke. In: 2008 IEEE International Conference on Systems. Man and Cybernetics, pp. 1872–1877. IEEE, Singapore, Singapore (2008)
- Nasri, N., Orts-Escolano, S., Cazorla, M.: An sEMG-Controlled 3D Game for Rehabilitation Therapies: Real-Time Time Hand Gesture Recognition Using Deep Learning Techniques. Sensors. 20, 6451 (2020). https://doi.org/10.3390/s20226451Article Google Scholar
- Dhiman, A., Solanki, D., Bhasin, A., Das, A., Lahiri, U.: An intelligent, adaptive, performance-sensitive, and virtual reality-based gaming platform for the upper limb. Comput Anim Virtual Worlds. 29, e1800 (2018). https://doi.org/10.1002/cav.1800Article Google Scholar
- Vallejo, D., Gmez-Portes, C., Albusac, J., Glez-Morcillo, C., Castro-Schez, J.J.: Personalized Exergames Language: A Novel Approach to the Automatic Generation of Personalized Exergames for Stroke Patients. Appl. Sci. 10, 7378 (2020). https://doi.org/10.3390/app10207378Article Google Scholar
- Afyouni, I., Murad, A., Einea, A.: Adaptive Rehabilitation Bots in Serious Games. Sensors. 20, 7037 (2020). https://doi.org/10.3390/s20247037Article Google Scholar
- Aguilar-Lazcano, C.A., Rechy-Ramirez, E.J.: Performance analysis of Leap motion controller for finger rehabilitation using serious games in two lighting environments. Measurement 157, 107677 (2020). https://doi.org/10.1016/j.measurement.2020.107677Article Google Scholar
- Rechy-Ramirez, E.J., Marin-Hernandez, A., Rios-Figueroa, H.V.: A human-computer interface for wrist rehabilitation: a pilot study using commercial sensors to detect wrist movements. Vis Comput. 35, 41–55 (2019). https://doi.org/10.1007/s00371-017-1446-xArticle Google Scholar
- Burke, J.W., McNeill, M.D.J., Charles, D.K., Morrow, P.J., Crosbie, J.H., McDonough, S.M.: Optimising engagement for stroke rehabilitation using serious games. Vis Comput. 25, 1085–1099 (2009). https://doi.org/10.1007/s00371-009-0387-4Article Google Scholar
- de los Reyes-Guzmàin, A., Lozano-Berrio, V., Alvarez-Rodríguez, M., López-Dolado, E., Ceruelo-Abajo, S., Talavera-Díaz, F., Gil-Agudo, A.: RehabHand: Oriented-tasks serious games for upper limb rehabilitation by using Leap Motion Controller and target population in spinal cord injury. NeuroRehabilitation 48(3), 365–373 (2021). https://doi.org/10.3233/NRE-201598
- Harvey, C., Selmanović, E., O’Connor, J., Chahin, M.: A comparison between expert and beginner learning for motor skill development in a virtual reality serious game. Vis Comput. 37, 3–17 (2021). https://doi.org/10.1007/s00371-019-01702-wArticle Google Scholar
- David, L., Bouyer, G., Otmane, S.: Towards a low-cost interactive system for motor self-rehabilitation after stroke. IJVR. 17, 40-45 (2017). https://doi.org/10.20870/IJVR.2017.17.2.2890
- Li, C., Cheng, L., Yang, H., Zou, Y., Huang, F.: An Automatic Rehabilitation Assessment System for Hand Function Based on Leap Motion and Ensemble Learning. Cybern. Syst. 52, 3–25 (2021). https://doi.org/10.1080/01969722.2020.1827798Article Google Scholar
- de Souza, M.R.S.B., Gonçalves, R.S., Carbone, G.: Feasibility and Performance Validation of a Leap Motion Controller for Upper Limb Rehabilitation. Robotics 10, 130 (2021). https://doi.org/10.3390/robotics10040130Article Google Scholar
- Koter, K., Samowicz, M., Redlicka, J., Zubrycki, I.: Hand Measurement System Based on Haptic and Vision Devices towards Post-Stroke Patients. 23 (2022)
- David, L.: Conception et évaluation d’un système de réalité virtuelle pour l’assistance à l’auto-rééducation motrice du membre supérieur post-AVC. 165
- Orhan, U., Hekim, M., Ozer, M.: EEG signals classification using the K-means clustering and a multilayer perceptron neural network model. Expert Syst. Appl. 38, 13475–13481 (2011). https://doi.org/10.1016/j.eswa.2011.04.149Article Google Scholar
- Bangor, A.: Determining What Individual SUS Scores Mean: Adding an Adjective Rating Scale. 4, 10 (2009)Google Scholar
- Franck, J.A., Timmermans, A.A.A., Seelen, H.A.M.: Effects of a dynamic hand orthosis for functional use of the impaired upper limb in sub-acute stroke patients: A multiple single case experimental design study. TAD. 25, 177–187 (2013). https://doi.org/10.3233/TAD-130374Article Google Scholar
Source: https://link.springer.com/article/10.1007/s00530-023-01180-0
[Abstract] Machine learning and big data in precision medicine: what is the role of the Radiologist?
Posted by Kostas Pantremenos in Artificial intelligence, Radiology/Imaging technology on October 16, 2023
With the advent of artificial intelligence (AI) in the field of radiology, a new perspective opens up in terms of diagnosis and management of patients. There is a need to review the way radiologists work so as to rebuild the doctor-patient relationship that has been sidelined over the years to increase our productivity. It is precisely the improvement in productivity that will be made possible by AI that will be able to free the radiology physician from time-consuming activities that add little to the diagnostic value of our work; this “gift of time” will have to be used to have a direct relationship with the patient, who can be followed up directly by the radiology physician, and not just sent by other physicians. This will be all the more necessary since with the new methods of image analysis (deep learning, texture analysis) the radiologist physician will not only have the task of diagnosing a lesion as accurately as possible, but also of indicating its evolution and progression, what makes indispensable a new pact with the patient, who will have to not only “accept” the diagnosis of an existing lesion but, above all, will have to trust the prognosis of that lesion, a trust based on an immaterial datum (the advanced image analysis) but which weighs like a boulder on the psyche of the patient. Only a relationship of great trust with his new physician, the radiologist, can make him follow our directions.
Source: https://www.minervamedica.it/en/journals/radiologia-medica/article.php?cod=R24Y2023N03A0107
[News] Link Found Between Brain Age and Stroke Outcomes: International Study
Posted by Kostas Pantremenos in REHABILITATION on April 7, 2023
Keck School of Medicine of USC
A new study lead by a team of researchers at the Keck School of Medicine of USC shows that younger “brain age,” a neuroimaging-based assessment of global brain health, is associated with better post-stroke outcomes. The findings could lead to better ways to predict post-stroke outcomes and offer insight on new potential treatment targets to improve recovery.
Understanding why some stroke survivors show better recovery than others despite similar damage to the brain has been a critical goal in stroke research, since it could help researchers develop better stroke rehabilitation therapies. During a stroke, blood flow to part of the brain is cut off. Without oxygen, brain cells are damaged and eventually die, resulting in brain damage known as a lesion. Studies have shown that people with similar amounts of lesion damage can experience varying amounts of recovery. Much research in the past two decades has focused on the specific location of brain damage and how the lesion affects connected networks in the brain.
This study, published April 4, 2023 in Neurology®, takes into consideration global brain health, a new way of analyzing the health of the brain based on its cellular, vascular, and structural integrity. Although global brain health has been widely examined in aging and neurodegenerative disease such as Alzheimer’s disease, it had not previously been studied in relation to stroke rehabilitation outcomes. Led by Sook-Lei Liew, PhD, of the Keck School of Medicine’s Mark and Mary Stevens Neuroimaging and Informatics Institute (Stevens INI), the team of researchers focused on a specific measure of global brain health known as brain age, which examines the biology of the nervous system through whole brain structural neuroimaging, hypothesizing that the integrity of residual brain tissue, or what is left after the stroke, may be critical for post-stroke outcomes.
Brain age is a biomarker that predicts chronological age based on neuroimaging of structures such as regional thickness, surface area, and volumes, and is calculated using advanced machine learning algorithms, which have been widely studied at the Stevens INI. A higher brain predicted age difference, calculated as the difference between a person’s predicted brain age minus their chronological age, suggests that the brain appears to be older than the person’s chronological age. An older-appearing brain has been associated with Alzheimer’s disease, major depression, traumatic brain injury, and more.
“Brain age has not been widely explored in stroke. A lot of stroke research has focused on how damage to the brain results in negative health outcomes, but there has been less research on how the integrity of the remaining brain tissue supports recovery. We expected that younger-appearing brains would be buffered from the effects of the lesion damage and therefore have less impacts on behavior,” says Sook-Lei Liew, PhD, lead author of the study and associate professor with joint appointments at the Stevens INI, the Chan Division of Occupational Science and Occupational Therapy, the Division of Biokinesiology and Physical Therapy, and the USC Viterbi School of Engineering.
The research team conducted an observational study using a multi-site data set of 3D brain structural MRIs and clinical measures from ENIGMA Stroke Recovery, a collaborative working group of more than 100 experts worldwide who pool together post-stroke MRI data to create well-powered, diverse samples. The primary mission of the group is to create a worldwide network of stroke neuroimaging centers focused on understanding the mechanisms of stroke recovery.
The new study showed that younger brain age is associated with superior post-stroke outcomes. The researchers note that inclusion of imaging-based assessments of brain age and brain resilience may improve the prediction of post-stroke outcomes and open new possibilities for potential therapeutic targets.
“The health of your overall brain can protect you from the functional consequences of stroke. That is, the healthier your brain is, first, the less likely you are to have a stroke, and second, the less likely you are to have poor outcomes if you do have a stroke. There’s so much research on the aging brain right now, and therapeutics being developed to slow brain aging. This study ties brain aging to stroke outcomes, so any therapeutics developed to slow brain aging might also be helpful to improve outcomes after stroke,” notes Liew.
For this study, the team of experts used high-resolution MRI data from research studies. They plan to progress their brain age assessment work by applying it to routine clinical MRI data to determine if it can be an easily implemented biomarker for stroke rehabilitation outcomes. Researchers at the Stevens INI collaborate on a variety of stroke research, including the Stroke Pre-Clinical Assessment Network (SPAN), which was established to address a significant need in the scientific investigation of stroke treatment. Additionally, Liew and other USC collaborators recently released an expanded, open-source data set of brain scans from stroke patients in hopes of accelerating large-scale stroke recovery research.
/Public Release. This material from the originating organization/author(s) might be of the point-in-time nature, and edited for clarity, style and length. Mirage.News does not take institutional positions or sides, and all views, positions, and conclusions expressed herein are solely those of the author(s).View in full here.
[ARTICLE] Hand Exoskeleton Design and Human–Machine Interaction Strategies for Rehabilitation – Full Text
Posted by Kostas Pantremenos in Paretic Hand, REHABILITATION on November 19, 2022
Abstract
Stroke and related complications such as hemiplegia and disability create huge burdens for human society in the 21st century, which leads to a great need for rehabilitation and daily life assistance. To address this issue, continuous efforts are devoted in human–machine interaction (HMI) technology, which aims to capture and recognize users’ intentions and fulfil their needs via physical response. Based on the physiological structure of the human hand, a dimension-adjustable linkage-driven hand exoskeleton with 10 active degrees of freedom (DoFs) and 3 passive DoFs is proposed in this study, which grants high-level synergy with the human hand. Considering the weight of the adopted linkage design, the hand exoskeleton can be mounted on the existing up-limb exoskeleton system, which greatly diminishes the burden for users. Three rehabilitation/daily life assistance modes are developed (namely, robot-in-charge, therapist-in-charge, and patient-in-charge modes) to meet specific personal needs. To realize HMI, a thin-film force sensor matrix and Inertial Measurement Units (IMUs) are installed in both the hand exoskeleton and the corresponding controller. Outstanding sensor–machine synergy is confirmed by trigger rate evaluation, Kernel Density Estimation (KDE), and a confusion matrix. To recognize user intention, a genetic algorithm (GA) is applied to search for the optimal hyperparameters of a 1D Convolutional Neural Network (CNN), and the average intention-recognition accuracy for the eight actions/gestures examined reaches 97.1% (based on K-fold cross-validation). The hand exoskeleton system provides the possibility for people with limited exercise ability to conduct self-rehabilitation and complex daily activities.
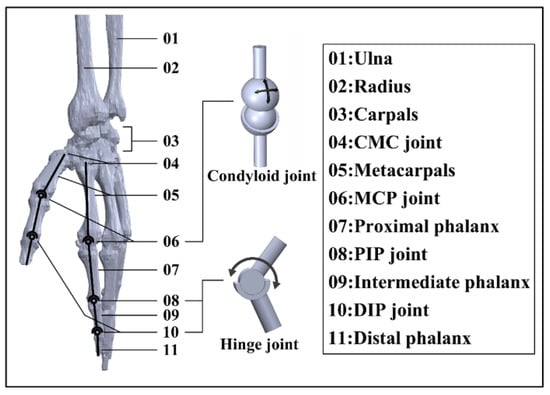
This is an open access article distributed under the Creative Commons Attribution License which permits unrestricted use, distribution, and reproduction in any medium, provided the original work is properly cited.
Supplementary Material
- Supplementary File 1:ZIP-Document (ZIP, 4037 KiB)
[Book Chapter] Virtual Reality, Robotics, and Artificial Intelligence: Technological Interventions in Stroke Rehabilitation
Posted by Kostas Pantremenos in Books, REHABILITATION, Rehabilitation robotics, Tele/Home Rehabilitation, Virtual reality rehabilitation on October 16, 2022
Abstract
Stroke is a leading cause of death in humans. In the US, someone has a stroke every 40 seconds. More than half of the stroke-affected patients over the age of 65 have reduced mobility. The prevalence of stroke in our society is increasing; however, since stroke comes with a lot of post-hospitalization care, a lot of infrastructure is lacking to cater to the demands of the increasing population of patients. In this chapter, the authors look at three technological interventions in the form of machine learning, virtual reality, and robotics. They look at how the research is evolving in these fields and pushing for easier and more reliable ways for rehabilitation. They also highlight methods that show promise in the area of home-based rehabilitation.
Chapter Preview
Introduction
Stroke is one of the leading causes of death in humans, and the recovery process is long and arduous. Patients once discharged from hospitals after primary treatment for stroke are advised to go on a long-term rehabilitation program. This includes routine visits to hospitals and a home-based exercise regime. Studies have pointed out that many patients drop out of the outpatient rehabilitation program due to recurring costs and the added discomfort of traveling to and from the rehabilitation centres. There is also a large disparity in the distribution of rehabilitation centres. A large number of centres are usually located in big cities. These are some of the major obstacles that patients who want to participate in a rehabilitation program face. The ability of technology to bring standardized rehabilitation care to the comfort of their homes with little clinical intervention is the need of this age. In this chapter, we look at virtual reality, machine learning, and robotic assistance devices that have successfully been applied to the rehabilitation of stroke patients. This chapter is divided into three components: 1) Machine Learning based Rehabilitation Techniques, 2) VR based Rehabilitation, 3) Robotic Devices based Rehabilitation. In the first component, we look at how machine learning models can help with movement evaluation, which can improve home-based therapy while also lowering recurring costs. Such systems, on the other hand, require extensive training and testing. Any machine learning system must be trained using a reliable source of unbiased data. We will look at a technique that requires a few well-executed workout sequences. A depth camera will be used to capture the data. These movements provide first-order statistics, which are then used to provide feedback for future movements given to the model. This method also presents a novel way for aligning temporal data, which is critical for calculating accurate movement statistics over numerous video sequences. In the second component, we’ll look into virtual-reality (VR) assisted rehabilitation options. One of VR’s most important characteristics is its ability to gamify the repetitive training plans prescribed to patients at home. VR games can help patients establish a self-interest in playing the game, which can lead to better rehabilitation outcomes. For post-stroke therapy, we look at a virtual reality-assisted motor training system. The technology has been shown to actively alter human kinetic behaviour based on patient-specific rehabilitation goals. Patients’ kinetic performance can also be accurately collected with this method. Five post-stroke patients were investigated over the course of three months. To track patient progress over time, three parameters were used: performance time, movement efficiency, and moving speed. Another VR assisted rehabilitation method recreates a virtual version of the buzz-wire game. The technology is intended to assist people with upper-body rehabilitation and fine motor skills improvement following a stroke. There were five different wires to choose from the game. Stability and fine motor abilities are required to navigate the game’s wires. Patients can benefit from playing this game on a regular basis to help them improve in this area. The system was tested on six stroke patients with hemiparesis. The patients reported no negative side effects from playing the game. In the third component, we will look at robotic devices that aid in rehabilitation. In this section, we will look at a variety of technological solutions that provide various types of assistance, such as active, passive, haptic, and coaching. We go over the benefits and drawbacks of each system, as well as a few commercially available systems. The ability of such systems to engage the patient through touch and force is their primary advantage. These systems can aid in the delivery of more precise treatments. However, because these are highly complex systems. Their high costs make home-based rehabilitation impractical and what has been done to address this issue. We looked at a variety of techniques developed in the fields of machine learning, virtual reality, and robotic assistance devices. These technologies have been successfully demonstrated. Large-scale deployment in home-based rehabilitation scenarios, on the other hand, is still lacking. One possible explanation is that few companies are converting this technology into usable products that people can buy and use at home. A proactive collaboration between the medical and engineering communities, on the other hand, has the potential to turn much of the technology into a product that patients can use at home. We believe the reader will be well informed after reading the contents of this chapter, which aims to outline major innovations in the field of stroke rehabilitation.
[ARTICLE] A Simple and Effective Way to Study Executive Functions by Using 360° Videos – Full Text
Posted by Kostas Pantremenos in REHABILITATION on August 16, 2022
Executive dysfunctions constitute a significant public health problem due to their high impact on everyday life and personal independence. Therefore, the identification of early strategies to assess and rehabilitate these impairments appears to be a priority. The ecological limitations of traditional neuropsychological tests and the numerous difficulties in administering tests in real-life scenarios have led to the increasing use of virtual reality (VR) and 360° environment-based tools for assessing executive functions (EFs) in real life. This perspective aims at proposing the development and implementation of Executive-functions Innovative Tool 360° (EXIT 360°), an innovative, enjoyable, and ecologically valid tool for a multidimensional and multicomponent evaluation of executive dysfunctions. EXIT 360° allows a complete and integrated executive functioning assessment through an original task for EFs delivered via a mobile-powered VR headset combined with eye tracker (ET) and electroencephalograms (EEG). Our tool is born as a 360°-based instrument, easily accessible and clinically usable, that will radically transform clinicians’ and patient’s assessment experience. In EXIT 360°, patients are engaged in a “game for health,” where they must perform everyday subtasks in 360° daily life environments. In this way, the clinicians can obtain quickly more ecologically valid information about several aspects of EFs (e.g., planning, problem-solving). Moreover, the multimodal approach allows completing the assessment of EFs by integrating verbal responses, reaction times, and physiological data (eye movements and brain activation). Overall, EXIT 360° will allow obtaining simultaneously and in real time more information about executive dysfunction and its impact in real life, allowing clinicians to tailor the rehabilitation to the subject’s needs.
Introduction
The executive dysfunctions in psychiatric and neurological pathologies constitute significant public health problems due to their high impact on personal independence, ability to work, educational success, social relationships, and cognitive and psychological development (Goel et al., 1997; Green et al., 2000; Diamond, 2013). Consequently, the assessment and the rehabilitation of these deficits must be early and adequate (Van der Linden et al., 2000). However, the evaluation of executive functions (EFs) represents a challenge due not only to the complexity of the construct (Stuss and Alexander, 2000) but also to the methodological difficulties (Chaytor and Schmitter-Edgecombe, 2003; Barker et al., 2004).
In the following paragraphs, we will briefly analyze the complex construct of EFs and the main tools for their evaluation.[…]

[ARTICLE] Machine learning predicts clinically significant health related quality of life improvement after sensorimotor rehabilitation interventions in chronic stroke – Full Text
Posted by Kostas Pantremenos in REHABILITATION on July 10, 2022
Abstract
Health related quality of life (HRQOL) reflects individuals perceived of wellness in health domains and is often deteriorated after stroke. Precise prediction of HRQOL changes after rehabilitation interventions is critical for optimizing stroke rehabilitation efficiency and efficacy. Machine learning (ML) has become a promising outcome prediction approach because of its high accuracy and easiness to use. Incorporating ML models into rehabilitation practice may facilitate efficient and accurate clinical decision making. Therefore, this study aimed to determine if ML algorithms could accurately predict clinically significant HRQOL improvements after stroke sensorimotor rehabilitation interventions and identify important predictors. Five ML algorithms including the random forest (RF), k-nearest neighbors (KNN), artificial neural network, support vector machine and logistic regression were used. Datasets from 132 people with chronic stroke were included. The Stroke Impact Scale was used for assessing multi-dimensional and global self-perceived HRQOL. Potential predictors included personal characteristics and baseline cognitive/motor/sensory/functional/HRQOL attributes. Data were divided into training and test sets. Tenfold cross-validation procedure with the training data set was used for developing models. The test set was used for determining model performance. Results revealed that RF was effective at predicting multidimensional HRQOL (accuracy: 85%; area under the receiver operating characteristic curve, AUC-ROC: 0.86) and global perceived recovery (accuracy: 80%; AUC-ROC: 0.75), and KNN was effective at predicting global perceived recovery (accuracy: 82.5%; AUC-ROC: 0.76). Age/gender, baseline HRQOL, wrist/hand muscle function, arm movement efficiency and sensory function were identified as crucial predictors. Our study indicated that RF and KNN outperformed the other three models on predicting HRQOL recovery after sensorimotor rehabilitation in stroke patients and could be considered for future clinical application.
Introduction
Health related quality of life (HRQOL) refers to the way an individual feels and reacts to his/her health status affected by medical conditions1. Compared to quality of life that covers all aspects of well-beings of human life, HRQOL focuses more on well-beings related to health domains such as physical, functional and mental health and it has been regarded as an important outcome of treatments1,2.
Stroke remains a leading cause of long-term disability3. It has a wide-ranging impact not only on physical and daily function but also on HRQOL4. Most patients still suffered from deteriorated HRQOL even in the chronic phase of stroke, which makes HRQOL an crucial target for stroke rehabilitation4,5. To improve patients’ HRQOL, healthcare professionals have to provide rehabilitation interventions that are most effective for each patient based on his/her responses to that rehabilitation therapy. Building accurate prediction models for forecasting patients’ HRQOL improvements after rehabilitation interventions and identifying predictors relevant for HRQOL improvements in stroke patients are thus imperative for providing insights to healthcare professionals on making accurate clinical decision.
Machine learning (ML) has become a popular prediction analytic approach. Machine learning uses automatic computerized algorithms to discover patterns in the data and builds prediction models to forecast future events. Machine learning is particularly suitable for predicting health outcomes because it can process large volumes of data, analyze the complex relationship between various different features/variables and easily incorporate new variables into prediction models without re-adjusting the preprogrammed rules6. In addition, the feature selection procedure can be incorporated into machine learning procedures to help identify important predictors7. These advantages make machine learning a potentially ideal tool for realizing accurate outcome prediction in patient populations.
In stroke, machine learning has been primarily used for predicting motor and activities of daily living (ADL) recovery and has achieved an overall positive result8,9,10,11,12. However, to our knowledge, only one study to date has applied machine learning algorithms in predicting stroke-specific HRQOL recovery13. In that study, the authors incorporated six demographic factors into machine learning models and built a preliminary system to forecast HRQOL changes of chronic stroke patients. Small prediction errors (i.e., the root mean square errors) were found between the data derived from the prediction model and the actual data collected from the patient, suggesting that machine learning might be feasible for predicting HRQOL changes in chronic stroke patients13.
Despite this positive evidence, the previous study only included demographic attributes into the machine learning prediction model13; nevertheless, HRQOL has been shown to be affected by factors across multiple domains including demographic as well as health-related domains such as physical and functional domains4,5,14. Including only demographic attributes in the machine learning model may not be sufficient for optimizing prediction accuracy. In addition, the previous study only examined prediction errors (e.g., the mean squared error) of the machine learning model13. Important clinical performance metrics such as prediction accuracy and the ability of machine learning models to distinguish between responders and non-responders to rehabilitation interventions remain largely unexplored15. A comprehensive examination of machine learning prediction performance along with factors across health domains is required for determining the efficacy of machine learning on predicting HRQOL recovery of stroke patients after rehabilitation interventions.
Stroke sensorimotor rehabilitation interventions including the robot-assisted therapy (RT), mirror therapy (MT) and transcranial direct current stimulation (tDCS) have become popular approaches for improving stroke recovery in the recent decade. These three approaches (i.e., RT, MT and tDCS) use modern equipment/modalities (e.g., robotic arms, mirror boxes and electrical stimulators) to modulate peripheral and/or central sensorimotor systems (e.g., visuomotor and sensorimotor systems and cortical areas) to augment stroke recovery16,17,18. Several studies have demonstrated that these three sensorimotor interventions (i.e., RT, MT and tDCS) not only facilitated functional recovery but also improved participation and HRQOL in stroke patients19,20,21,22,23,24,25. The rationale of why these three sensorimotor interventions (i.e., RT, MT and tDCS) could improve HRQOL is that these interventions could reduce arm/hand impairment, restore arm/hand function, which would allow stroke patients to participate in daily activities and accomplish essential daily tasks19,20,21,22,23,24,25. Most daily tasks such as bathing, dressing, dining and grocery shopping all involve use of the arm/hand to manipulate objects to accomplish tasks. Good arm/hand function would lead to successful participation in daily tasks and subsequently may increase stroke patients’ subjective feeling of well-beings and satisfaction toward daily life19,20,21,22,23,24,25. Thus, these three interventions (i.e., RT, MT and tDCS) may have potentials to be incorporated into current clinical practice to facilitate not only functional recovery but also HRQOL in stroke patients. Machine learning may be a potentially useful tool for predicting HRQOL changes after these three interventions, which may help identify responders to these three interventions and facilitate clinical application6,7.
Therefore, the purpose of this study was to determine the performance of machine learning algorithms on predicting clinically significant HRQOL improvements of chronic stroke patients after stroke sensorimotor rehabilitation interventions including the RT, MT and tDCS. We examined the performance of five commonly used machine learning algorithms and identified important predictors for building machine learning prediction models. […]
[WEB] Artificial Intelligence and Mental Health
Posted by Kostas Pantremenos in Artificial intelligence on June 11, 2022

One of the primary challenges faced by researchers and clinicians seeking to study mental health is that direct observation of indicators of mental health issues can be challenging, as a diagnosis often relies on either self-reporting of specific feelings or actions, or direct observation of a subject (which can be difficult due to time and cost considerations). That is why there has been a specific focus over the past two decades on deploying technology to help human clinicians identify and assess mental health issues.
Between 2000 and 2019, 54 academic papers focused on the development of machine learning systems to help diagnose and address mental health issues were published, according to a 2020 article published in ACM Transactions on Computer-Human Interaction. Of the 54 papers, 40 focused on the development of a machine learning (ML) model based on specific data as their main research contribution, while seven were proposals of specific concepts, data methods, models, or systems, and three applied existing ML algorithms to better understand and assess mental health, or improve the communication of mental health providers. A few of the papers described the conduct of empirical studies of an end-to-end ML system or assessed the quality of ML predictions, while one paper specifically discusses design implications for user-centric, deployable ML systems.
Despite the voluminous amount of research being conducted, challenges remain with relying on ML to identify mental health issues, given the significant amount of patient data that traditionally has been required to train these ML models. Previous studies cited in an April 2020 article published in Translational Psychiatry have indicated neuroimages can record evidence of neuropsychiatric disorders, with two common types of neuroimage data being used to identify changes in the brain that could indicate mental health issues. Functional magnetic resonance imaging (fMRI) can be used to identify the changes associated with blood flow in the brain, based on the fact that cerebral blood flow and neuronal activation are coupled. Using structural magnetic resonance imaging (sMRI) data, the neurological aspect of a brain is described based on the structural textures, which show some information in terms of the spatial arrangements of voxel intensities in three dimensions.
However, a study led by researcher Denis Engemann of France-based research institute Inria Saclay, which was published in October 2021 in the open-access research journal GigaScience, found that applying ML to brain scans, medical data, and the results of a questionnaire about personal circumstances, habits, moods, and demographic data from large population cohorts can yield “proxy measures” for brain-related health issues without the need for a specialist’s assessment.
These so-called “proxy measures,” indirect measurements that strongly correlate with specific diseases or outcomes that cannot be measured directly, were developed by the Inria research team by tapping two data sources held by the U.K. Biobank, a large long-term study investigating the respective contributions of genetic predisposition and environmental exposure to the development of disease. The first source included biological and medical data, including magnetic resonance imaging (MRI) data, from 10,000 participants. The team then incorporated U.K. Biobank’s questionnaire data about personal conditions and habits, such as age, education, tobacco and alcohol use, sleep duration, and physical exercise, as well as sociodemographic and behavioral data such as the moods and sentiments of the individuals covered in the study.
The Inria team combined these data sources to build ML models that approximated measures for brain age, and scientifically defined intelligence and neuroticism traits. According to lead researcher Engemann, this methodology of creating proxy measures via predictions of “brain age” from MRI scans, along with other sociodemographic and behavioral data, can be used to identify mental health markers that can be useful to both psychologists and end users.
According to a Q&A with Engemann released concurrently with the study, “Given the brain image of a person, the resulting model will provide a prediction by returning the most probable questionnaire result by extrapolating from people whose brains ‘looked’ similar,” said Engemann. “Thus, the predicted questionnaire result can become a proxy for the construct measured by the questionnaire. This reflects the statistical link between questionnaire data and brain images, and therefore can enrich the original questionnaire measure.”
Engemann noted ML is likely to become a tool to help psychologists conduct personalized mental health assessments, with clients or patients granting an ML model secure access to their social media accounts or mobile phone data, which would return the proxy measures useful to both the client and the mental health or education expert.
Furthermore, once a model has been constructed, a proxy measure can be obtained even if a mental health questionnaire has not specifically been assessed. “This is a promising method for finding large-scale statistical patterns of health within the general population,” said Engemann, which can be used to enhance smaller clinical studies if there isn’t sufficient training data on which to run a bespoke ML model.
A cohort for which proxy measures might be useful in assessing their mental health states is social media users, particularly children and adolescents. In mid-2021, internal research documents leaked by former Facebook employee Frances Haugen that were provided to The Wall Street Journal showed Instagram exacerbated body-image issues for one in three teenage girls who used that social media service. The release of this internal data played into the commonly accepted narrative that social media negatively impacts its users. However, a closer inspection of the research indicates the Facebook internal research was neither peer reviewed nor designed to be nationally representative, and some of the statistics that have received the most attention in the popular press were based on very small numbers.
That said, a combination of the research approach detailed by Engemann, combined with longitudinal studies that follow the same subjects over time, may help researchers and clinicians get a better handle on the actual impact of social media on users’ mental states and well-being.
“I think the hard part of the social media stuff is we don’t have the right inputs,” says Dr. John Torous, a researcher and co-author of “Artificial Intelligence for Mental Health Care: Clinical Applications, Barriers, Facilitators, and Artificial Wisdom,” a research article published last year that explored the use of AI to assist psychologists with clinical diagnosis, prognosis, and treatment of mental health issues.
Applying ML models to these robust datasets is likely where significant benefits to researchers will be found, says Torous. “If you look at almost every current [research] paper, it’s just going to be [focused on] cross-sectional data with exposure,” he says. However, longitudinal studies, which can encompass following individual subjects for years, are often very complex, with many temporal interactions and lots of dependencies. “That’s where I think the machine learning models become very useful,” Torous says, adding, “we have to be capturing longitudinal data on both social media exposure and the state of [the subject’s] mood.”
Another key challenge, according to Torous, is the cultural aspect surrounding the reporting of mental health issues. “The cultural part just makes it really tricky, because people from different cultures may be reporting symptoms differently.”
One approach that may help to mitigate the cultural and individual issues of self-reporting is being evaluated by Sonde Health (www.sondehealth.com), a technology company that uses markers within a user’s voiceprint as indicators of several physical and mental health conditions. The company developed biomarkers by focusing on the acoustic aspects of the human voice, taking speech samples ranging in length from six to 30 seconds, then breaking down each sample into its most basic elements. Sonde developed algorithms to match more than 4,000 acoustic voice features with a database of normal voices and the voices of those that have been diagnosed with a specific disease or condition. As a result, specific voice features can be used as predictors of mental or physical health conditions.
Sonde CEO David Liu says his company has isolated four or five dozen acoustic features indicative that something may be amiss with a person’s mental health, indicating the person should consider being evaluated, though Liu says the technology is not designed to make actual mental health diagnoses.
“We have six specific vocal features that give us a read on if something needs to be paid attention to,” Liu says. “Now, we’re not diagnosing for depression; we’re not diagnosing for anxiety, but these six features—voice smoothness, control, liveliness, energy, range, and clarity—are well-studied vocal features, and we understand what is in the normal range and what is not, based upon prior published research. What we’re doing now is taking that product and putting it into clinical trial research, so that we can then see if we can align it to some of these other assessments that are well-accepted.”
The company is working closely with mobile device chipset manufacturers such as Qualcomm to have the technology embedded into the firmware of a device, so it can be activated or deactivated within specific applications. This would allow the capture of voice information during the normal flow of a day, which Liu says is more natural and may result in more data being captured across not only social media sites, but also from multiplayer games, chats, or other communications.
“When you’re speaking on TikTok, or when you’re on Zoom or any IP-driven applications, you need to be able to capture voice samples while people are doing whatever they’re doing; you don’t want to stop somebody and say, ‘hey, tell me about your day’, because that’s a little bit artificial,” Liu says. “If we have enough of those voice samples, we can begin to produce these insights and can give them indications that, because you’ve been on this [service], and it’s having an impact on your mental health, you’re not going to be feeling as good because you’re spending 10 hours straight on social media.”
Longitudinal data indicating changes in a person’s mental state can be indicative of a mental health problem, rather than simply having a bad day.
Liu notes that Sonde does not capture or analyze the content of the voice samples, just the acoustic markers; from a privacy standpoint, that might make the technology more palatable to both manufacturers and users. Further, while Liu says that Sonde’s technology does not rely on longitudinal data as it has gathered voice data across a wide swath of people from different countries and cultures, the use of longitudinal data when making a longer-term health assessment can be beneficial.
“Now, when we have longitudinal data, meaning I get a baseline for a user, and then we take a measurement again, a day later, two days later, and a week later, then we can understand the changes and it makes our technologies even more powerful from a monitoring standpoint when we have that baseline,” Liu says, noting that longitudinal data indicating changes in a person’s mental state can be indicative of a mental health problem, rather than someone simply having a bad day.
One of the key challenges with any sort of mental health assessment is getting people to agree either to be tracked or to be studied, given their potential huge privacy concerns, as well as the social stigma surrounding mental health.
“How are we going to build these really big, big datasets?” asks Torous, who notes that as a researcher and clinician, he needs to go to the companies that amass such data (such as Facebook) to capture data on a scale that will yield meaningful research results. Torous says lack of trust remains a huge issue, and getting people to willingly participate in any sort of mental health monitoring may require the development of an independent, health-focused platform.
“I wonder if we’re going to have to build new systems that are really just built for health; that’s not going to be a social platform, an advertising platform, or a shopping platform,” Torous says, noting that researchers would need to offer clinically actionable insight from such data that is useful and focused on a very targeted problem. Further, focusing on a symptom of mental health, such as cognition, may prove useful, as there isn’t the same stigma attached to asking about cognition, compared with asking about hot-button issues such as depression, suicide, or other more direct mental health indicators or conditions.
Further Reading
Dadi, K., Engemann, D. et. al.
Population modeling with machine learning can enhance measures of mental health. GigaScience, Volume 10, Issue 10, October 2021, giab071, https://doi.org/10.1093/gigascience/giab071
Lee, E.E., Torous, J. et al.
Artificial Intelligence for Mental Health Care: Clinical Applications, Barriers, Facilitators, and Artificial Wisdom, Biological Psychiatry: Cognitive Neuroscience and Neuroimaging, February 2021. DOI: https://doi.org/10.1016/j.bpsc.2021.02.001
Su, C., Xu, Z., Pathak, J., and Wang, F.
Deep learning in mental health outcome research: a scoping review, Transl Psychiatry 10, 116 (2020). https://doi.org/10.1038/s41398-020-0780-3
Thieme, A., Belgrave, D., and Doherty, G.
Machine Learning in Mental Health: A Systematic Review of the HCI Literature to Support the Development of Effective and Implementable ML Systems, ACM Transactions on Computer-Human Interaction, Volume 27, Issue 5, October 2020 Article No.: 34, pp 1–53, https://doi.org/10.1145/3398069
Zauner, H.
AI for mental health assessment: Author Q&A, Giga Science, October 14, 2021, http://gigasciencejournal.com/blog/ai-for-mental-health/
Author
Keith Kirkpatrick is Principal of 4K Research & Consulting, LLC, based in New York, NY, USA.
©2022 ACM 0001-0782/22/5
Permission to make digital or hard copies of part or all of this work for personal or classroom use is granted without fee provided that copies are not made or distributed for profit or commercial advantage and that copies bear this notice and full citation on the first page. Copyright for components of this work owned by others than ACM must be honored. Abstracting with credit is permitted. To copy otherwise, to republish, to post on servers, or to redistribute to lists, requires prior specific permission and/or fee. Request permission to publish from permissions@acm.org or fax (212) 869-0481.
The Digital Library is published by the Association for Computing Machinery. Copyright © 2022 ACM, Inc.