Posts Tagged Hand
[Abstract + References] Research progress and development trend of flexible hand rehabilitation gloves
Posted by Kostas Pantremenos in Paretic Hand, REHABILITATION, Rehabilitation robotics on February 20, 2024
Abstract
The flexible hand rehabilitation glove is proposed to solve the problems of long rehabilitation training time for patients and high workload for doctors, and to make the treatment more effective. With the advances in robotics, robotic-assisted therapy has developed rapidly and has become an essential complement to conventional treatment. To understand flexible hand rehabilitation glove devices, with the different construction types of actuators and drive methods as the mainline, the corresponding study of these structures as the auxiliary lines. The characteristics and the current state of research have been discussed. A brief introduction to manufacturing actuators and rehabilitation systems is also given. Through the analysis of hand rehabilitation gloves, some current advantages and disadvantages are summarized, and future directions and functional diversification are envisaged. Certain feasible research suggestions have been proposed for future development regarding structure, functional diversity, and a combination of driving methods. These include that there will be more combinations of pneumatic and motor driven, combining the advantages of both methods to overcome the disadvantages of each. The structural design will be more in line with anatomy and ergonomics, make it more esthetically pleasing. More innovative controls methods will be adopted to achieve more complex rehabilitation functions.
References
1. Taylor CL, Schwarz RJ. The anatomy and mechanics of the human hand. Artif Limbs 1955; 2(2): 22–35.
2. Chim H. Hand and wrist anatomy and biomechanics: a comprehensive guide. Plast Reconstr Surg 2017; 140(4): 865–865.
3. Nas K, Yazmalar L, Şah V, et al. Rehabilitation of spinal cord injuries. World J Orthop 2015; 6(1): 8–16.
4. Silva NA, Sousa N, Reis RL, et al. From basics to clinical: a comprehensive review on spinal cord injury. Prog Neurobiol 2014; 114: 25–57.
5. Davis A. Medical, psychosocial and vocational aspects of disability, 4th edition.J Rehabil 2015; 81(3): 58–59.
6. Gassert R, Dietz V. Rehabilitation robots for the treatment of sensorimotor deficits: a neurophysiological perspective. J Neuroeng Rehabil 2018; 15(1): 46.
7. Mlinac ME, Feng MC. Assessment of activities of daily living, self-care, and independence. Arch Clin Neuropsychol 2016; 31(6): 506–516.
8. Rudd AG, Bowen A, Yang GR. The latest national clinical guideline for stroke. Clin Med 2017; 17(4): 382–383.
9. O’Driscoll SW, Giori NJ. Continuous passive motion (CPM): theory and principles of clinical application. J Rehabil Res Dev 2000; 37(2): 179–188.
10. Bütefisch C, Hummelsheim H, Denzler P, et al. Repetitive training of isolated movements improves the outcome of motor rehabilitation of the centrally paretic hand. J Neurol Sci 1995; 130(1): 59–68.
11. Iqbal J, Baizid K. Stroke rehabilitation using exoskeleton-based robotic exercisers: mini review. Biomed Res 2014; 26(26): 197–201.
12. Rotella M, Reuther K, Hofmann C, et al. An orthotic hand-assistive exoskeleton for actuated pinch and grasp. In: 2009 IEEE 35th annual northeast bioengineering conference, Cambridge, MA, 3–5 April 2009, pp.338–339. New York: IEEE.
13. Li G, Cheng L, Gao Z, et al. Development of an untethered adaptive thumb exoskeleton for delicate rehabilitation assistance. IEEE Trans Robot 2022; 38(6): 3514–3529.
14. Kang B, In H, Cho K. Modeling of tendon driven soft wearable robot for the finger. In: 2013 10th international conference on ubiquitous robots and ambient intelligence (URAI), Jeju, South Korea, 30 October–2 November 2013, pp.459–460. New York: IEEE.
15. Yap HK, Lim JH, Goh JCH, et al. Design of a soft robotic glove for hand rehabilitation of stroke patients with clenched fist deformity using inflatable plastic actuators. J Med Device 2016; 10(4): 044504.
16. Yap HK, Lim JH, Nasrallah F, et al. Characterisation and evaluation of soft elastomeric actuators for hand assistive and rehabilitation applications. J Mar Eng Technol 2016; 40(4): 199–209.
17. Shahid T, Gouwanda D, Nurzaman SG, et al. Moving toward soft robotics: a decade review of the design of hand exoskeletons. Biomimetics 2018; 3(3): 17.
18. Ahmed Y, Ai-Neami, Lateef S. Robotic glove for rehabilitation purpose: review. In: The 3rd scientific conference of electrical and electronic engineering researches (SCEEER), Basrah, Iraq, 15–16 June 2020, pp.15–16. New York: IEEE.
19. du Plessis T, Djouani K, Oosthuizen C. A review of active hand exoskeletons for rehabilitation and assistance. Robotics 2021; 10(1): 40.
20. Tran P, Jeong S, Herrin KR, et al. Review: hand exoskeleton systems, clinical rehabilitation practices, and future prospects. IEEE Trans Med Robot Bionics 2021; 3(3): 606–622.
21. Pérez Vidal AF, Rumbo Morales JY, Ortiz Torres G, et al. Soft exoskeletons: development, requirements, and challenges of the last decade. Actuators 2021; 10(7): 166.
22. Tiboni M, Amici C. Soft gloves: a review on recent developments in actuation, sensing, control and applications. Actuators 2022; 11(8): 232.
23. Heo P, Gu GM, Lee SJ, et al. Current hand exoskeleton technologies for rehabilitation and assistive engineering. Int J Precis Eng Manuf 2012; 13(5): 807–824.
24. Balasubramanian S, Klein J, Burdet E. Robot-assisted rehabilitation of hand function. Curr Opin Neurol 2010; 23(6): 661–670.
25. Fonseca MCR, Elui VMC, Lalone E, et al. Functional, motor, and sensory assessment instruments upon nerve repair in adult hands: systematic review of psychometric properties. Syst Rev 2018; 7(1): 175.
26. Pataky TC, Latash ML, Zatsiorsky VM. Multifinger Ab- and adduction strength and coordination. J Hand Ther 2008; 21(4): 377–385.
27. Hunter S, Crome P. Hand function and stroke. Rev Clin Gerontol 2002; 12(1): 68–81.
28. Boser QA, Dawson MR, Schofield JS, et al. Defining the design requirements for an assistive powered hand exoskeleton: a pilot explorative interview study and case series. Prosthet Orthot Int 2021; 45(2): 161–169.
29. Aubin PM, Sallum H, Walsh C, et al. A pediatric robotic thumb exoskeleton for at-home rehabilitation: the isolated orthosis for thumb actuation (IOTA). Int J Intell Comput Cybern 2014; 7(3): 233–252.
30. Hines AE, Crago PE, Billian C. Hand opening by electrical stimulation in patients with spastic hemiplegia. IEEE Trans Rehabil Eng 1995; 3(2): 193–205.
31. Ilievski F, Mazzeo AD, Shepherd RF, et al. Soft robotics for chemists. Angew Chem 2011; 50(8): 1890–1935.
32. Polygerinos P, Lyne S, Wang Z, et al. Towards a soft pneumatic glove for hand rehabilitation. In: 2013 IEEE/RSJ international workshop on intelligent robots and systems (IROS), Tokyo, Japan, 3–7 November 2013, pp.1512–1517. New York: IEEE.
33. Tiboni M, Loda D. Monolithic PneuNets soft actuators for robotic rehabilitation: methodologies for design, production and characterization. Actuators 2023; 12(7): 299.
34. Yang Y, Chen Y, Li Y, et al. Bioinspired robotic fingers based on pneumatic actuator and 3D printing of smart material. Soft Robot 2017; 4(2): 147–162.
35. Yap H, Lim J, Nasrallah F, et al. A soft exoskeleton for hand assistive and rehabilitation application using pneumatic actuators with variable stiffness. In: 2015 IEEE international conference on robotics and automation (ICRA), Seattle, WA, 26–30 May 2015, pp.4967–4972. New York: IEEE.
36. Wang J, Fei Y, Pang W. Design, modeling, and testing of a soft pneumatic glove with segmented pneunets bending actuators. IEEE/ASME Trans Mechatron 2019; 24(3): 990–1001.
37. Guo S, Zhao F, Wei W, et al. Soft actuator for hand rehabilitation. In: 2015 IEEE international conference on mechatronics and automation (ICMA), Beijing, China, 2–5 August 2015. New York: IEEE.
38. Mosadegh B, Polygerinos P, Keplinger C, et al. Pneumatic networks for soft robotics that actuate rapidly. Adv Funct Mater 2014; 24(15): 2163–2170.
39. Lalegani Dezaki M, Bodaghi M, Serjouei A, et al. Soft pneumatic actuators with controllable stiffness by bio-inspired lattice chambers and fused deposition modeling 3D printing. Adv Eng Mater 2023; 25(6): 2200797.
40. Li X, Lin Y, Jia J. Design and implementation of a hand rehabilitation device for stroke. Mech Eng Technol 2021; 50(3): 104–105.
41. Jiang Y, Chen D, Liu P, et al. Fishbone-inspired soft robotic glove for hand rehabilitation with multi-degrees-of-freedom. In: 2018 IEEE international conference on soft robotics (RoboSoft), Livorno, Italy, 24–28 April 2018, pp.394–399. New York: IEEE.
42. Meng F, Liu C, Li Y, et al. Personalized and safe soft glove for rehabilitation training. Electronics 2023; 12(11): 2531.
43. Zhang J, Hu D, Hong J, et al. A user-defined passive pneumatic soft body manipulator. CN Patent 110 236 880. September17, 2019.
44. Haghshenas-Jaryani M, Patterson RM, Bugnariu N, et al. A pilot study on the design and validation of a hybrid exoskeleton robotic device for hand rehabilitation. J Hand Ther 2020; 33(2): 198–208.
45. Fan S, Jin M, Li B, et al. Rigid-flexible coupled extensible articulated soft exoskeleton glove and method. CN Patent 111 150 602, 15 May 2020.
46. Yang K, Mao Z, Yamamoto K. Finger joint rehabilitation device. US Patent 10 751 244, 25 August 2020.
47. Yang R, Shi H, Yuan L, et al. A wearable soft hand functional rehabilitation glove. CN Patent 111 821 140, 27 October 2020.
48. Liu D, Wang M, Bi C, et al. A wearable finger rehabilitation device with a combination of rigid and soft activation and its manufacturing method. CN Patent 110 772 402, 11 February 2020.
49. Hu D, Zhang J, Yang Y, et al. A novel soft robotic glove with positive-negative pneumatic actuator for hand rehabilitation. In: 2020 IEEE/ASME international conference on advanced intelligent mechatronics (AIM), Boston, MA, 6–9 July 2020. New York: IEEE.
50. Yun SS, Kang BB, Cho KJ. Exo-Glove PM: an easily customizable modularized pneumatic assistive glove. IEEE Robot Autom Lett 2017; 2(3): 1725–1732.
51. Heung KHL, Tong RKY, Lau ATH, et al. Robotic glove with soft-elastic composite actuators for assisting activities of daily living. Soft Robot 2019; 6(2): 289–304.
52. Heung K, Tong Z, Ho L, et al. Design of a 3D printed soft robotic hand for stroke rehabilitation and daily activities assistance. In: 2019 IEEE 16th international conference on rehabilitation robotics (ICORR), Toronto, ON, 24–28 June 2019, pp.65–70. New York: IEEE.
53. Connolly F, Polygerinos P, Walsh CJ, et al. Mechanical programming of soft actuators by varying fiber angle. Soft Robot 2015; 2(1): 26–32.
54. Polygerinos P, Wang Z, Overvelde JTB, et al. Modeling of soft fiber-reinforced bending actuators. IEEE Trans Robot 2015; 31(3): 778–789.
55. Wang B, McDaid A, Aw K, et al. Design and development of a skinny bidirectional soft glove for post-stroke hand rehabilitation. In: 2017 intelligent systems conference (IntelliSys), London, 7–8 September 2017, pp.979–987. New York: IEEE.
56. Ramos O, Múnera M, Moazen M, et al. Assessment of soft actuators for hand exoskeletons: pleated textile actuators and fiber-reinforced silicone actuators. Front Bioeng Biotechnol 2022; 10: 924888.
57. Lin M, Paul R, Abd M, et al. Feeling the beat: a smart hand exoskeleton for learning to play musical instruments. Front Rob AI 2023; 10: 1212768.
58. Wang Y, Kokubu S, Zhou Z, et al. Designing soft pneumatic actuators for thumb movements. IEEE Robot Autom Lett 2021; 6(4): 8450–8457.
59. Tarvainen T, Fernandez-Vargas J, Yu W. New layouts of fiber reinforcements to enable full finger motion assist with pneumatic multi-chamber elastomer actuators. Actuators 2018; 7(2): 31.
60. Meng M. A hand-assisted rehabilitation drive. CN Patent 110 840 706, 28 February 2020.
61. Cao X, Ma K, Jiang Z, et al. A soft robotic glove for hand rehabilitation using pneumatic actuators with jamming structure. In: 2021 40th Chinese control conference (CCC), Shanghai, China, 26-28 July 2021. New York: IEEE.
62. Zhang H, Kumar AS, Chen F, et al. Topology optimized multimaterial soft fingers for applications on grippers, rehabilitation, and artificial hands. IEEE/ASME Trans Mechatron 2019; 24(1): 120–131.
63. Meng M. Structural design of a soft hand functional rehabilitation robot glove. CN Patent 112 999 014, 22 June 2021.
64. Kladovasilakis N, Kostavelis I, Sideridis P, et al. A novel soft robotic exoskeleton system for hand rehabilitation and assistance purposes. Appl Sci 2022; 13(1): 553.
65. Meng N, Kun W, Mingxin L, et al. Design, analysis and experiment of finger soft actuator with nested structure for rehabilitation training. Adv Mech Eng 2020; 12(11): 1–15.
66. Sun ZS, Guo ZH, Tang W. Design of wearable hand rehabilitation glove with soft hoop-reinforced pneumatic actuator. J Central South Univ 2019; 26: 106–119.
67. Polygerinos P, Wang Z, Galloway KC, et al. Soft robotic glove for combined assistance and at-home rehabilitation. Robot Auton Syst 2015; 73: 135–143.
68. Li Z, Lv H, Li Q. An articulated soft body rehabilitation robot glove. CN Patent 113 332 104, 3 September 2021.
69. Gaylord R. Fluid actuated motor system and stroking device. US Patent 2 844 126, 22 July 1958.
70. Daerden F, Lefeber D. Pneumatic artificial muscles: actuators for robotics and automation. Eur J Mech Env Eng 2002; 47: 11–21.
71. Baldwin HA. Realizable models of muscle function. In Biomechanics: Proceedings of the first rock island arsenal biomechanics symposium april 5–6, 1967. Springer, New York, 1969, pp.139–147.
72. Andrikopoulos G, Nikolakopoulos G, Manesis S. Pneumatic artificial muscles: a switching model predictive control approach. Control Eng Pract 2013; 21(12): 1653–1664.
73. Andrikopoulos G, Nikolakopoulos G, Manesis S. Design and development of an exoskeletal wrist prototype via pneumatic artificial muscles. Meccanica 2015; 50(11): 2709–2730.
74. Takosoglu JE, Laski PA, Blasiak S, et al. Determining the static characteristics of pneumatic muscles. Meas Control 2016; 49(2): 62–71.
75. Al-Fahaam H, Davis S, Nefti-Meziani S. The design and mathematical modelling of novel extensor bending pneumatic artificial muscles (EBPAMs) for soft exoskeletons. Robot Auton Syst 2018; 99: 63–74.
76. Peng G, Fan X, Liu X, et al. Design and control of flexible wearable rehabilitation gloves. Med Biomech 2019; 34(6): 637–643.
77. Takahashi N, Furuya S, Koike H. Soft exoskeleton glove with human anatomical architecture: production of dexterous finger movements and skillful piano performance. IEEE Trans Haptics 2020; 13(4): 679–690.
78. Wang J, Liu Z, Fei Y. Design and testing of a soft rehabilitation glove integrating finger and wrist function. J Mech Robot 2019; 11(1): 011015.
79. Maldonado-Mejía JC, Múnera M, Diaz CAR, et al. A fabric-based soft hand exoskeleton for assistance: the exhand exoskeleton. Front Neurorobot 2023; 17: 1091827.
80. Elmoughni HM, Yilmaz AF, Ozlem K, et al. Machine-knitted seamless pneumatic actuators for soft robotics: design, fabrication, and characterization. Actuators 2021; 10(5): 94.
81. Yap HK, Khin PM, Koh TH, et al. A fully fabric-based bidirectional soft robotic glove for assistance and rehabilitation of hand impaired patients. IEEE Robot Autom Lett 2017; 2(3): 1383–1390.
82. Feng M, Yang D, Gu G. High-force fabric-based pneumatic actuators with asymmetric chambers and interference-reinforced structure for soft wearable assistive gloves. IEEE Robot Autom Lett 2021; 6(2): 3105–3111.
83. Ge L, Chen F, Wang D, et al. Design, modeling, and evaluation of fabric-based pneumatic actuators for soft wearable assistive gloves. Soft Robot 2020; 7(5): 583–596.
84. Jeong U, In HK, Cho KJ. Implementation of various control algorithms for hand rehabilitation exercise using wearable robotic hand. Intell Serv Robot 2013; 6(4): 181–189.
85. Aoun A, Lliovits A, Kassem A, et al. Arthro-Glove a hybrid bionic glove for patients diagnosed with arthritis, als and/or dysmorphia. In: 2018 9th Cairo international biomedical engineering conference (CIBEC), Cairo, Egypt, 20–22 December 2018, pp.106–109. New York: IEEE.
86. Xu D, Wu Q, Zhu Y. Development of a soft cable-driven hand exoskeleton for assisted rehabilitation training. Ind Rob 2021; 48(2): 189–198.
87. Alnajjar F, Umari H, Ahmed WK, et al. CHAD: compact hand-assistive device for enhancement of function in hand impairments. Robot Auton Syst 2021; 142: 103784.
88. Biggar S, Yao W. Design and evaluation of a soft and wearable robotic glove for hand rehabilitation. IEEE Trans Neural Syst Rehabil Eng 2016; 24(10): 1071–1080.
89. Serrano D, Copaci D, Arias J, et al. SMA-based soft exo-glove. IEEE Robot Autom Lett 2023; 8(9): 5448–5455.
90. Abdelhafiz MH, Andreasen Struijk LNS, Dosen S, et al. Biomimetic tendon-based mechanism for finger flexion and extension in a soft hand exoskeleton: design and experimental assessment. Sensors 2023; 23(4): 2272.
91. Chen W, Li G, Li N, et al. Soft exoskeleton with fully actuated thumb movements for grasping assistance. IEEE Trans Robot 2022; 38(4): 2194–2207.
92. Tran P, Jeong S, Lyu F, et al. FLEXotendon Glove-iii: voice-controlled soft robotic hand exoskeleton with novel fabrication method and admittance grasping control. IEEE/ASME Trans Mechatron 2022; 27(5): 3920–3931.
93. Kang B, Lee H, In H, et al. Development of a polymer-based tendon-driven wearable robotic hand. In: 2016 IEEE International Conference on Robotics and Automation (ICRA), Stockholm, 16–21 May 2016, pp.3750–3755. New York: IEEE.
94. Kin D, Heo S, Park H. Biomimetic finger extension mechanism for soft wearable hand rehabilitation devices. In: 2017 international conference on rehabilitation robotics (ICORR), London, 17–20 July 2017, pp.1326–1330. New York: IEEE.
95. Setiawan JD, Ariyanto M, Nugroho S, et al. Fuzzy logic control for a soft exoskeleton glove using a motor-tendon actuator. Ing Invest 2021; 41(1): e81531.
96. Tang D, Lv X, Qi L, et al. A tendon wire driven exoskeleton for functional hand rehabilitation. CN Patent 115 284 261, 4 November 2022.
97. Mohammadi A, Lavranos J, Choong P, et al. Flexo-glove: a 3D printed soft exoskeleton robotic glove for impaired hand rehabilitation and assistance. In: 2018 40th annual international conference of the IEEE engineering in medicine and biology society (EMBC), Honolulu, HI, 18–21 July 2018, pp.2120–2123. New York: IEEE.
98. Liu A, Qiu J, Wang F, et al. An adaptive and jointless hand exoskeleton system design. In: 2018 15th international conference on control, automation, robotics and vision (ICARCV), Singapore, 18–21 November 2018, pp.579–584. New York: IEEE.
99. Arata J, Ohmoto K, Gassert R, et al. A new hand exoskeleton device for rehabilitation using a three-layered sliding spring mechanism. In: 2013 IEEE international conference on robotics and automation, Karlsruhe, Germany, 6–10 May 2013, pp.3902–3907. New York: IEEE.
100. Nazari V, Pouladian M, Zheng YP, et al. A compact and lightweight rehabilitative exoskeleton to restore grasping functions for people with hand paralysis. Sensors 2021; 21(20): 6900.
101. Lin L, Zhang F, Yang L, et al. Design and modeling of a hybrid soft-rigid hand exoskeleton for poststroke rehabilitation. Int J Mech Sci 2021; 212: 106831.
102. Bützer T, Lambercy O, Arata J, et al. Fully wearable actuated soft exoskeleton for grasping assistance in everyday activities. Soft Robot 2021; 8(2): 128–143.
103. Gerez L, Gao G, Dwivedi A, et al. A hybrid, wearable exoskeleton glove equipped with variable stiffness joints, abduction capabilities, and a telescopic thumb. IEEE Access 2020; 8: 173345–173358.
104. Stilli A, Cremoni A, Bianchi M, et al. Airexglove – a novel pneumatic exoskeleton glove for adaptive hand rehabilitation in post-stroke patients. In: 2018 IEEE international conference on soft robotics (RoboSoft), Livorno, Italy, 24–28 April 2018, pp.579–584. New York: IEEE.
105. Tang D, Qi L, Shen C, et al. A flexible hand rehabilitation exoskeleton and its control method. CN Patent 116 650 285, 29 August 2023.
106. Cho KJ, Koh JS, Kim S, et al. Review of manufacturing processes for soft biomimetic robots. Int J Precis Eng Manuf 2009; 10: 171–181.
107. Yap H, Goh J, Yeow R. Design and characterization of soft actuator for hand rehabilitation application. In: 6th European conference of the international federation for medical and biological engineering, Dubrovnik, Croatia, 7–11 September 2014, vol. 45, pp.367–370.
108. Yirmibesoglu O, Menguc Y. Hybrid soft sensor with embedded imus to measure motion. In: 2016 IEEE international conference on automation science and engineering (CASE), Fort Worth, TX, 21–25 August 2016, pp.798–804. New York: IEEE.
109. Sun Y, Song S, Liang X, et al. A miniature soft robotic manipulator based on novel fabrication methods. IEEE Robot Autom Lett 2016; 1(2): 617–623.
110. Gong Z, Xie Z, Yang X, et al. Design, fabrication and kinematic modeling of a 3D-motion soft robotic arm. In: 2016 IEEE international conference on robotics and biomimetics (ROBIO), Qingdao, China, 3–7 December 2016, pp.509–514. New York IEEE.
111. Wallin TJ, Pikul J, Shepherd RF. 3D printing of soft robotic systems. Nat Rev Mater 2018; 3: 84–100.
112. Hinton TJ, Jallerat Q, Palchesko RN, et al. Three-dimensional printing of complex biological structures by freeform reversible embedding of suspended hydrogels. Sci Adv 2015; 1(9): e1500758.
113. Nyberg EL, Farris AL, Hung BP, et al. 3D-printing technologies for craniofacial rehabilitation, reconstruction, and regeneration. Ann Biomed Eng 2017; 45(1): 45–57.
114. Wolf SL, Blanton S, Baer H, et al. Repetitive task practice: a critical review of constraint-induced movement therapy in stroke. Neurologist 2002; 8(6): 325–338.
115. Altschuler EL, Wisdom SB, Stone L, et al. Rehabilitation of hemiparesis after stroke with a mirror. Lancet 1999; 353(9169): 2035–2036.
116. Boos A, Qiu Q, Fluet G, et al. Haptically facilitated bimanual training combined with augmented visual feedback in moderate to severe hemiplegia. In: 2011 annual international conference of the IEEE engineering in medicine and biology society, Boston, MA, 30 August–3 September 2011, pp.3111–3114. New York: IEEE.
117. Kim SY, Kim YY. Mirror therapy for phantom limb pain. Korean J Pain 2012; 25(4): 272–274.
118. Chen X, Gong L, Wei L, et al. A wearable hand rehabilitation system with soft gloves. IEEE Trans Ind Inform 2021; 17(2): 943–952.
119. Li M, Wang T, Zhou Y, et al. A soft robotic glove for hand rehabilitation training controlled by movements of the healthy hand. In: 2020 17th International Conference on Ubiquitous Robots (UR), Kyoto, Japan, 22–26 June 2020. New York: IEEE.
120. Moraru E, Onose G. Current issues and considerations about the central role of rehabilitation therapies in the functional recovery of neurological impairments after stroke in adults. J Med Life 2014; 7(3): 368–372.
121. Harvey RL. Improving poststroke recovery: neuroplasticity and task-oriented training. Curr Treat Options Cardiovasc Med 2009; 11(3): 251–259.
122. Hoang CL, Salle JY, Mandigout S, et al. Physical factors associated with fatigue after stroke: an exploratory study. Top Stroke Rehabil 2012; 19(5): 369–376.
123. Bates B, Choi JY, Duncan PW, et al.; US Department of Defense and Department of Veterans Affairs. Veterans affairs/department of defense clinical practice guideline for the management of adult stroke rehabilitation care: executive summary. Stroke 2005; 36(9): 2049–2056.
124. Merians AS, Jack D, Boian R, et al. Virtual reality–augmented rehabilitation for patients following stroke. Phys Ther Rehabil 2002; 82(9): 898–915.
125. Holden MK. Virtual environments for motor rehabilitation: review. Cyberpsychol Behav 2005; 8(3): 187–211.
126. Connelly L, Jia Y, Toro ML, et al. A pneumatic glove and immersive virtual reality environment for hand rehabilitative training after stroke. IEEE Trans Neural Syst Rehabil Eng 2010; 18(5): 551–559.
127. Merians AS, Fluet GG, Qiu Q, et al. Robotically facilitated virtual rehabilitation of arm transport integrated with finger movement in persons with hemiparesis. J Neuroeng Rehabil 2011; 8(1): 27–10.
128. Mitsopoulos K, Fiska V, Tagaras K, et al. Neurosuitup: system architecture and validation of a motor rehabilitation wearable robotics and serious game platform. Sensors 2023; 23(6): 3281.
[WEB] Smart Glove Technology: Revolutionizing Stroke Recovery
Posted by Kostas Pantremenos in Assistive Technology, Paretic Hand on January 17, 2024

By Zara Nwosu
Stroke can have devastating effects on patients, leading to mobility, functionality, and communication challenges. A key part of stroke recovery is rehabilitation, particularly aimed at restoring the use of affected limbs and hands. Innovative technology is now contributing to this field, with a group of stroke survivors in B.C. set to test a new smart glove designed to aid their recovery.
Understanding the Smart Glove Technology
Developed by engineers and researchers at the University of British Columbia, the smart glove integrates highly sensitive sensors and pressure sensors into a comfortable fabric. These sensors work to track hand and finger movements during rehabilitation exercises, providing precise and fast data. This data can be wirelessly transmitted, enabling remote monitoring and analysis of exercise programs, and potentially transforming stroke recovery procedures.
According to data available on ScienceDaily, and MedicalXpress, the smart glove not only aids with movements but also provides feedback. This makes it a powerful tool for stroke recovery, as it helps patients develop better control over their hand movements, leading to increased mobility and functionality.
Applications Beyond Stroke Recovery
While the primary goal of the smart glove is to aid in stroke recovery, its potential applications extend beyond this. Given its ability to accurately track hand movements and interactions with objects, it can be utilized in the fields of augmented reality, robotics, and virtual reality.
Moreover, the smart glove has the potential to translate American Sign Language into written speech in real-time. This can significantly benefit individuals who are deaf or hard of hearing, opening up new avenues for communication.
The Future of Smart Glove Technology
As reported by MedRiva, and a product review on Amazon, the smart glove technology is in its development and testing phase. Clinical trials are ongoing to evaluate its effectiveness further, and results so far have been promising.
With the advancement of technology, recovery from stroke could become faster and more efficient. Smart glove technology could become a standard part of rehabilitation, helping stroke survivors regain control over their lives.
Conclusion
Stroke recovery is a challenging journey, but innovations like the smart glove are making it more manageable. As technology continues to evolve, we can hope for more such breakthroughs that can significantly improve the quality of life for stroke survivors and other individuals facing mobility challenges.
[WEB] Revolutionizing Stroke Recovery: The Smart Glove Aid
Posted by Kostas Pantremenos in Artificial intelligence, Assistive Technology, Paretic Hand on January 17, 2024

In recent years, technology has played a crucial role in transforming the healthcare sector. Among the exciting innovations, the ‘smart glove’ stands out as a groundbreaking tool designed to aid stroke survivors in their recovery process. Created by a team at Texavie, this innovative technology is set to revolutionize stroke rehabilitation by providing precise and personalized therapy for each individual.
The Concept Behind the Smart Glove
The smart glove is a technological marvel that incorporates a network of highly sensitive sensor yarns and pressure sensors woven into a comfortable, stretchy fabric. This innovative design enables the glove to track even the minutest hand and finger movements during rehabilitation exercises. It’s much like a wearable motion-capture camera, capturing movements with unparalleled precision and speed.
What sets the glove apart is its ability to wirelessly transmit this data, allowing for remote monitoring and analysis of exercise programs. This feature is especially crucial in times where social distancing is the norm, enabling therapists to track their patients’ progress remotely. It also allows patients to engage in rehabilitation exercises from the comfort of their homes, making the recovery process more flexible and less stressful.
The Role of Artificial Intelligence
Artificial Intelligence (AI) plays a pivotal role in the functionality of the smart glove. AI technology not only helps track movements but also analyses the data to provide personalized therapy for each individual. This aspect of individualization is vital in stroke rehabilitation, where each person’s recovery process is unique and requires a tailored approach.
AI-driven technology in the glove adapts the rehabilitation program to the individual’s recovery pace and progress. It can adjust the difficulty level of exercises, making them more challenging as the patient’s hand function and mobility improve. This adaptive capability ensures that the rehabilitation process is efficient and effective, promoting faster recovery.
Testimonials and Success Stories
Stroke survivors who have used this innovative smart glove technology have shared their success stories, providing real-life evidence of the glove’s effectiveness. Users have reported significant improvements in hand function and mobility, contributing to an overall better quality of life post-stroke.
The smart glove has not only helped stroke survivors regain their independence but also boosted their confidence. The ability to track progress in real-time has been highly motivating, encouraging patients to stay committed to their rehabilitation program.
Future Applications
While currently focused on aiding stroke rehabilitation, the smart glove’s potential applications extend beyond this. The technology’s precision and speed match the performance of costly motion-capture cameras, making it a potential tool for use in virtual reality, augmented reality, animation, and robotics. The smart glove technology could significantly impact these fields, making it a truly transformative invention.
In conclusion, the smart glove is a promising leap forward in stroke rehabilitation. It not only offers a personalized approach to recovery but also enables remote therapy, making the process more accessible and convenient for stroke survivors. With its potential to expand into other fields, the smart glove is indeed a game-changer in healthcare technology.
[BOOK Chapter] Dynamic Difficulty Adjustment (DDA) on a Serious Game Used for Hand Rehabilitation
Posted by Kostas Pantremenos in Books, Paretic Hand, Video Games/Exergames on January 14, 2024
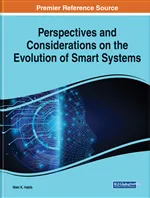
Abstract
Serious games have been used for assisting people in physical rehabilitation for hands. People might have different degrees of mobility in their hands; consequently, it would be convenient that the game could be adapted according to the range-of-motion in performing hand movements. This study implemented a serious game for hand rehabilitation with two play modes. Mode one does not adjust the game difficulty; whereas mode two adjusts the game difficulty according to the player’s range-of-motion in performing flexion, extension, ulnar, and radial deviations. The game difficulty was adjusted using fuzzy logic to compute positions at which the rewards will be displayed at the game scene (easy, medium, and difficult positions to collect the rewards). Four participants played both modes. Two-tailed t-tests revealed that there were no significant differences between both modes in terms of rewards collected (p = 0.6621), play time (p = 0.8178), and “game engagement questionnaire” score (p = 0.1383).
Chapter Preview
Introduction
Hands play a key role in daily activities. People use them to interact with the world. Nevertheless, due to accidents or medical conditions, people might lose mobility in their hands; consequently, they might require physical rehabilitation.
According to Walsh et al., (2002), “exercise forms a crucial part of a patient’s motor rehabilitation in terms of upper and lower limb function as well as prevention of muscle atrophy” (p. 2).
The main problem in the traditional rehabilitation method is the lack of motivation in patients; therefore, the performances of the rehabilitation exercises might become frustrated and boring. To cope with this issue, robots have been used to assist people during their motor rehabilitation exercises. For instance, a review on robots employed as assistive technologies for rehabilitation on upper limb can be found in (Narayan et al., 2021). In the same vein, robots have been employed for lower limb motor rehabilitation (Alvarez-Perez et al., 2020; Hussain et al., 2017, Hussain et al., 2021).
On the other hand, researchers (Lohse et al., 2013) have studied that video games can be used as a therapeutic tool in physical rehabilitation due to their motivational and engagement properties (e.g., optimal challenge, rewards and feedback provided to the players). As can be seen, these games are focused on assisting people in their rehabilitation processes. These types of games are called serious games. According to Zyda (2005), a serious game can be defined as “a mental contest, played with a computer in accordance with specific rules, that uses entertainment to further government or corporate training, education, health, public policy, and strategic communication objectives” (p. 26).
Figure 1.
Wrist joints

It is important to remark that serious games have been used to assist therapists in the rehabilitation processes of patients on emotional health aspects (e.g., anxiety and depression (Abd-Alrazaq et al., 2022; Barnes & Prescott, 2018; Dias et al., 2018), autism spectrum disorder (Silva et al., 2021; Tsikinas & Xinogalos, 2019), phobias: acrophobia (Sharmili & Kanagaraj, 2020), spider phobia (Lindner et al., 2020)) and motor rehabilitation (e.g. ankle rehabilitation (Hendrickx et al., 2021, Feng et al., 2018), finger rehabilitation (Rahman, 2017; Aguilar-Lazcano & Rechy-Ramirez, 2020), shoulder rehabilitation (Viglialoro et al., 2020; Steiner et al., 2020)), so that the patients could be engaged to the rehabilitation and therapy. Additionally, virtual reality has been used in serious games for upper limb rehabilitation. For instance, Wang et al., (2022) conducted a review on game-based virtual reality systems for upper limb rehabilitation on people that have suffered a stroke to assess the effectiveness of these systems. As a result, authors found that games based on virtual reality for upper limb rehabilitation are more effective than traditional rehabilitation on people suffering cerebral apoplexy.
In terms of wrist motor rehabilitation, the majority of these games are controlled using rehabilitation exercises for the wrist (e.g., flexion, extension, ulnar and radial deviations, pronation and supination of the wrist). The wrist has two main joints, radiocarpal joint and midcarpal joint, that are involved in these rehabilitation exercises (see Figure 1). The intensities of the movements depend on the range-of-motion (ROM) that the patients might have in their hands. According to the American Physical Therapy Association (2001), the range-of-motion “is the arc of motion that occurs at a joint or a series of joints”.
Key Terms in this Chapter
Engagement: it is the feeling of enjoying playing the game (i.e., the involvement in the game).
Fuzzy Logic: It was introduced by Lotfi Zadeh in 1965. Fuzzy logic could be employed to tackle data uncertainty through mainly three processes: fuzzification, fuzzy inference, and defuzzification.
Range-of-Motion: The number of degrees – arc – that a joint could achieve when it is moved from one position to another.
Serious Game: It is a video game played against a computer, where its main purpose is beyond the entertainment (i.e., it is designed to tackle health problems or train people mainly).
Dynamic Difficulty Adjustment (DDA): The game difficulty is modified continuously in the game in order to avoid boredom or frustration on the player.
Leap Motion Controller: It is a sensor based on infrared cameras and leds, which can identify X, Y, Z coordinates of the positions of the finger phalanges, palm, wrist and elbow.
Rehabilitation: A process that is needed in order to recover mobility. This could be done using body movements or exercises.
Complete Chapter List
Search this Book:
Reset
Table of Contents
Detailed Table of Contents
Preface
Maki K. Habib
Chapter 1
Alessandro Massaro
$37.50
Add to Cart
Chapter 2
The Explainable Model to Multi-Objective Reinforcement Learning Toward an Autonomous Smart System (pages 18-34)
Tomohiro Yamaguchi
$37.50
Add to Cart
Chapter 3
Dynamic Difficulty Adjustment (DDA) on a Serious Game Used for Hand Rehabilitation (pages 35-66)
Juan Ignacio Vargas-Bustos, Ericka Janet Rechy-Ramirez
$37.50
Add to Cart
Chapter 4
Evolution of Blockchain Technology: Principles, Research Trends and Challenges, Applications, and Future Directions (pages 67-104)
Oluwaleke Umar Yusuf, Maki K. Habib
$37.50
Add to Cart
Chapter 5
Mind of a Portfolio Investor: Which Strategies Should I Use as a Basis for My Investment Decisions (pages 105-119)
Chabi Gupta
$37.50
Add to Cart
Chapter 6
Modelling of Engineering Systems With Small Data: A Comparative Study (pages 120-136)
Morteza Mohammadzaheri, Mojtaba Ghodsi, Hamidreza Ziaiefar, Issam Bahadur, Musaab Zarog, Mohammadreza Emadi, Payam Soltani, Amirhosein Amouzadeh
$37.50
Add to Cart
Chapter 7
The Theory and Applications of the Software-Based PSK Method for Solving Intuitionistic Fuzzy Solid Transportation Problems (pages 137-186)
P. Senthil Kumar
$37.50
Add to Cart
Chapter 8
Metrics for Project Management Methodologies Elicitation (pages 187-212)
Patricia R. Cristaldo, Daniela Lopez De Luise, Lucas La Pietra
$37.50
Add to Cart
Chapter 9
An Overview of Security Issues in Cognitive Radio Ad Hoc Networks (pages 213-246)
Noman Islam, Muhammad Furqan Zia, Darakhshan Syed
$37.50
Add to Cart
Chapter 10
Energy Harvesting Systems: A Detailed Comparison of Existing Models (pages 247-295)
Afnan Khaled Elhamshari, Maki K. Habib
$37.50
Add to Cart
Chapter 11
Detecting Phishing URLs With Word Embedding and Deep Learning (pages 296-319)
Ali Selamat, Nguyet Quang Do, Ondrej Krejcar
$37.50
Add to Cart
Chapter 12
Prospects of Deep Learning and Edge Intelligence in Agriculture: A Review (pages 320-341)
Ali Shaheen, Omar F. El-Gayar
$37.50
Add to Cart
Chapter 13
Study on Healthcare Security System-Integrated Internet of Things (IoT) (pages 342-362)
S. A. Karthik, R. Hemalatha, R. Aruna, M. Deivakani, R. Vijaya Kumar Reddy, Sampath Boopathi
$37.50
Add to Cart
About the Contributors View Full PDF
Index View Full PDF
[ARTICLE] Brain oscillations in reflecting motor status and recovery induced by action observation-driven robotic hand intervention in chronic stroke – Full Text
Posted by Kostas Pantremenos in Paretic Hand, REHABILITATION, Rehabilitation robotics on December 17, 2023
Hand rehabilitation in chronic stroke remains challenging, and finding markers that could reflect motor function would help to understand and evaluate the therapy and recovery. The present study explored whether brain oscillations in different electroencephalogram (EEG) bands could indicate the motor status and recovery induced by action observation-driven brain–computer interface (AO-BCI) robotic therapy in chronic stroke. The neurophysiological data of 16 chronic stroke patients who received 20-session BCI hand training is the basis of the study presented here. Resting-state EEG was recorded during the observation of non-biological movements, while task-stage EEG was recorded during the observation of biological movements in training. The motor performance was evaluated using the Action Research Arm Test (ARAT) and upper extremity Fugl–Meyer Assessment (FMA), and significant improvements (p < 0.05) on both scales were found in patients after the intervention. Averaged EEG band power in the affected hemisphere presented negative correlations with scales pre-training; however, no significant correlations (p > 0.01) were found both in the pre-training and post-training stages. After comparing the variation of oscillations over training, we found patients with good and poor recovery presented different trends in delta, low-beta, and high-beta variations, and only patients with good recovery presented significant changes in EEG band power after training (delta band, p < 0.01). Importantly, motor improvements in ARAT correlate significantly with task EEG power changes (low-beta, c.c = 0.71, p = 0.005; high-beta, c.c = 0.71, p = 0.004) and task/rest EEG power ratio changes (delta, c.c = −0.738, p = 0.003; low-beta, c.c = 0.67, p = 0.009; high-beta, c.c = 0.839, p = 0.000). These results suggest that, in chronic stroke, EEG band power may not be a good indicator of motor status. However, ipsilesional oscillation changes in the delta and beta bands provide potential biomarkers related to the therapeutic-induced improvement of motor function in effective BCI intervention, which may be useful in understanding the brain plasticity changes and contribute to evaluating therapy and recovery in chronic-stage motor rehabilitation.
1 Introduction
Stroke has been the leading cause of acquired disability in adults globally for decades (Mendis, 2013). Although the mortality rate declined with improved healthcare, approximately 80% of stroke victims still experience motor impairment, and more than 30% of patients suffer despite intensive rehabilitation (Lai et al., 2002; Young and Forster, 2007). It is worse for the chronic group with severe motor impairments in the upper limbs. On the one hand, effective interventions like constraint-induced movement therapy (CIMT) may not be applicable to those patients without enough residual active movement (Thrasher et al., 2008). On the other hand, motor recovery in chronic stroke is more challenging due to the decreasing plasticity of spontaneous recovery (Cassidy and Cramer, 2017). Since the upper limbs, especially the hands, play a significant role in daily activity, exploring novel rehabilitation therapies for hand motor recovery in this group is essential (Neumann, 2016). Robot-assisted therapy (RAT) and motor imagery (MI) have been introduced to enhance motor recovery for stroke patients through passive motion or mental practice. However, although these interventions benefit training without requiring patients’ residual ability, rehabilitation effectiveness is still limited by a lack of active engagement (Kwakkel et al., 2008; Ietswaart et al., 2011). Recent advances in brain–computer interface (BCI) technology offer a novel method that could extract the motor intention of patients executing MI to support active rehabilitation training. Related studies have shown promising results that MI-actuated BCI improves motor ability more than pure MI or sham BCI (Ramos-Murguialday et al., 2013; Ang et al., 2014; Pichiorri et al., 2015). However, this intervention still faces limitations in practical use (Mulder, 2007; Baniqued et al., 2021). First, BCI may not be easy for everyone due to the “BCI illiteracy” phenomenon or the limited training schedule in clinical environments (Blankertz et al., 2009; Horowitz et al., 2021). In addition, most stroke subjects show more difficulty executing MI tasks than healthy subjects because of brain impairment in motor-related areas (Mulder, 2007). Worse situations occur in severe patients because they can hardly perform effective MI or fall into fatigue quickly under effortful attempts. Recent studies found that action observation (AO) could also activate sensorimotor features, as in MI and motor execution tasks (Friesen et al., 2017; Hardwick et al., 2017). In addition, repeated AO could induce plasticity changes by activating the mirror neuron system (MNS) (Rizzolatti and Sinigaglia, 2010; Agosta et al., 2017). These inspired studies combined AO in the BCI system, where stronger event-related desynchronization (ERD) responses are found than in pure MI-BCI (Kondo et al., 2015; Ono et al., 2018; Nagai and Tanaka, 2019). However, most of these studies focused on healthy subjects, while related endeavors in the clinical rehabilitation of stroke subjects are still insufficient.
Another major concern in exploring novel interventions in chronic stroke is better evaluating the motor deficits and understanding the therapeutic-induced improvement during rehabilitation neurologically. On the one hand, the recovery in post-stroke motor rehabilitation is usually heterogeneous. Except for individual factors such as age, time since stroke, and related complications, a variety of neuro-clinical factors, such as the degree of brain lesion and neural status, would also affect the patient’s recovery (Riley et al., 2011; Chang et al., 2013; Feng et al., 2015; Kim and Winstein, 2017). On the other hand, chronic stroke recovery is more challenging with the decreasing plasticity of spontaneous recovery and depends more on intervention-induced plasticity (Cassidy and Cramer, 2017). The routinely used assessment of motor recovery is on clinical scales, which are semi-objective and limited in monitoring the underlying neural factors. Hence, recent studies have focused on finding neural biomarkers that could serve as an additional physiological approach to probe brain status and reflect the extent of post-stroke functional recovery (Kim and Winstein, 2017). Potential biomarkers have been found in physiological measuring tools such as Functional magnetic resonance imaging (fMRI) and magnetoencephalograms (MEG) (Várkuti et al., 2013; Kim and Winstein, 2017).
Compared with these tools, electroencephalography (EEG) offers another economical and widely available choice, making it a more practical approach in clinical environments for rehabilitation (Gerloff et al., 2006; Ang and Guan, 2016). In addition, the EEG is easy to implement in EEG-based BCI interventions. However, most related investigations of EEG markers focused on acute or subacute-stage patients, and studies concerned with chronic patients are still lacking (Foreman and Claassen, 2012; Assenza et al., 2017; Trujillo et al., 2017; Bentes et al., 2018). Notably, EEG oscillations in different bands themselves play roles in reflecting the physiological and pathological status of the neural systems. For example, the increasing low-frequency power (delta and theta bands) and decreasing high-frequency power (alpha and beta bands) are believed to reflect the severity of acute neurological deficits (Rabiller et al., 2015; Assenza et al., 2017). Apart from reflecting the motor status, the EEG features may also promote an understanding of varied recovery resulting from additional factors during rehabilitation. For instance, a previous study found that patients under different interventions have different EEG indicators (Mane et al., 2019). We infer that patients with varying degrees of recovery may also differ in EEG features after experiencing different neural processes in training. Overall, how these EEG oscillations would act in chronic stroke and whether related EEG features could reflect therapeutic-induced improvement in effective interventions remains to be determined.
To fill this gap, the present study aimed to explore whether brain oscillations in different EEG bands can reflect the motor status and recovery induced by novel BCI therapy in chronic stroke. Specifically, an AO-BCI robotic hand training intervention was studied in a clinical environment, and the motor scales were assessed before and after the training. The correlations between EEG band power and motor scales both before and after the intervention were analyzed to study their feasibility in reflecting motor status by EEG band power in chronic stroke patients. In addition, we presented the difference in EEG variation during an intervention on patients with and without effective recovery [whether the minimal clinically important difference (MCID) was reached] (van der Lee et al., 2001; Wagner et al., 2008). Moreover, we examined which EEG rhythm variations correlate with motor function improvement and their potential as markers in reflecting therapeutic-induced neuroplasticity changes and guiding rehabilitation intervention in chronic stroke patients. […]

[Abstract + References] Development of a Finger Rehabilitation Device – Conference paper
Posted by Kostas Pantremenos in Paretic Hand on November 17, 2023
Abstract
This paper aims to develop a device for the rehabilitation of the fingers of the human hand. With the daily use of hands, they can suffer different types of injuries in accidents or injuries caused by different diseases. The rehabilitation of the human hand is fundamental for the recovery of its motor capacity. Hand rehabilitation procedures are generally performed by healthcare professionals who lack specialized equipment. The proposed device consists of an exoskeleton to be placed on the back of the human hand and can be used for a specific finger. This device must be capable of performing the flexion and extension movements of each finger joint independently or in a coupled manner.
References
- Alves, T., Gonçalves, R.S., Carbone, G.: Serious games strategies with cable-driven robots for bimanual rehabilitation: a randomized controlled trial with post-stroke patients. Front. Robot. AI 9, 1–22 (2022)CrossRef Google Scholar
- Rodrigues, L.A.O., Gonçalves, R.S.: Development of a novel body weight support system for gait rehabilitation. Robotica 1, 1–20 (2022)Google Scholar
- Gonçalves, R.S., Rodrigues, L.A.O.: Development of nonmotorized mechanisms for lower limb rehabilitation. Robotica 1, 1–18 (2021)Google Scholar
- Gonçalves, R.S., Furtado, L.S., Moraes, L.P., Carbone, G., Ceccarelli, M.: A fairly simple mechatronic device for training human wrist motion. Int. J. Adv. Rob. Syst. 1, 1–15 (2020)Google Scholar
- Kapandji, A.I.: The Physiology of the Joints, Volume 1: Upper Limb, vol. 1, 6th edn. Churchill Livingstone, London (2007)Google Scholar
- Liu, C., Lu, J., Yang, H., Guo, K.: Current state of robotics in hand rehabilitation after stroke: a systematic review. Appl. Sci. 12, 4540 (2022). https://doi.org/10.3390/app12094540CrossRef Google Scholar
- Ceccarelli, M., Morales-Cruz, C.: A prototype characterization of ExoFinger, a finger exoskeleton. Int. J. Adv. Robot. Syst. 18(3) (2021). https://doi.org/10.1177/17298814211024880
- Cafolla, D., Carbone, G.: A study of feasibility of a human finger exoskeleton. In: Borangiu, T., Trentesaux, D., Thomas, A. (eds.) Service Orientation in Holonic and Multi-Agent Manufacturing and Robotics, pp. 355–364. Springer, Cham (2014). ISBN: 978-3-319-04735-5. https://doi.org/10.1007/978-3-319-04735-5_24
- Carbone, G., Gerding, E.C., Corves, B., Cafolla, D., Russo, M., Ceccarelli, M.: Design of a two-DOFs driving mechanism for a motion-assisted finger exoskeleton. Appl. Sci. 10, 2619 (2020). https://doi.org/10.3390/app10072619CrossRef Google Scholar
- Carbone, G., Ceccarelli, M., Capalbo, C., Caroleo, G., Morales-Cruz, C.: Numerical and experimental performance estimation for a ExoFing – 2 DOFs finger exoskeleton. Robotica 40(6), 1820–1832 (2022). https://doi.org/10.1017/S0263574721001375CrossRef Google Scholar
- Rodríguez-León, J.F., Castillo-Castañeda, E., Aguilar-Pereyra, J.F., Carbone, G.: Experimental characterization of A-AFiM, an adaptable assistive device for finger motions. Machines 10, 280 (2022). https://doi.org/10.3390/machines10040280CrossRef Google Scholar
- Proulx, C.E., et al.: Review of the effects of soft robotic gloves for activity-based rehabilitation in individuals with reduced hand function and manual dexterity following a neurological event. J. Rehabil. Assist. Technol. Eng. 13(7), 2055668320918130 (2020). https://doi.org/10.1177/2055668320918130CrossRef Google Scholar
- du Plessis, T., Djouani, K., Oosthuizen, C.: A review of active hand exoskeletons for rehabilitation and assistance. Robotics 10, 40 (2021). https://doi.org/10.3390/robotics10010040CrossRef Google Scholar
- Fu, Y., Wang, P., Wang, S.: Development of a multi-DOF exoskeleton based machine for injured fingers. In: 2008 IEEE/RSJ International Conference on Intelligent Robots and Systems, France (2008)Google Scholar
- Levangie, P.K., Norkin, C.C.: Joint Structure and Function: A Comprehensive Analysis, 4th edn. F. A. Davis Company, Philadelphia (2005)Google Scholar
- Wu, J.Z., Dong, R.G., Mcdowell, T.W., Welcome, D.E.: Modeling the finger joint moments in a hand at the maximal isometric grip: the effects of friction. Med. Eng. Phys. 31(10), 1214–1218 (2009)CrossRef Google Scholar
- Tsai, L.W.: Robot Analysis – The Mechanics of Serial and Parallel Manipulators. Wiley, USA (1999)Google Scholar
- Silva, A.L.: Development of a system for finger rehabilitation. M.Sc. dissertation, Federal University of Uberlandia, Uberlandia, Brasil, 2011. https://repositorio.ufu.br/bitstream/123456789/14883/1/Diss%20Ana.pdf. Accessed 23 Apr 2023
- Ceccarelli, M.: Fundamentals of Mechanics of Robotic Manipulation. Springer (2004). ISBN-10: 140201810XGoogle Scholar
[Abstract] Preliminary Results from a Six-Week Home-Based Evaluation of a Rehabilitation Device for Hand and Wrist Therapy After Stroke
Posted by Kostas Pantremenos in Paretic Hand, REHABILITATION, Rehabilitation robotics, Tele/Home Rehabilitation on November 17, 2023
Abstract
While many robot-aided solutions have been proposed for the rehabilitation of the distal segment of the upper limb, very few take into account the synergy between the wrist and fingers to allow them to train simultaneously in a home environment. WiGlove is a passive robotic orthosis designed to address this need. This wearable, wireless device enables stroke survivors to perform flexion/extension exercises of both the wrist and fingers while performing ADL or playing therapeutic computer games. As a part of its user-centred design process, this paper presents a case study of a 6-week feasibility evaluation of the WiGlove conducted at a stroke survivor’s home without assistance from the therapists. The participant trained with the device for an average of 48 minutes a day and showed a noticeable reduction in the spasticity of the fingers and improved performance in the box and block test. He expressed satisfaction with its usability and suitability for the home environment. These results show overwhelmingly positive outcomes in terms of its acceptance, usability and effectiveness in offering home-based rehabilitation of the wrist and fingers.
[Abstract] Functional MRI Assessment of Brain Activity During Hand Rehabilitation with an MR-Compatible Soft Glove in Chronic Stroke Patients: A Preliminary Study
Posted by Kostas Pantremenos in Neuroplasticity, Paretic Hand, Radiology/Imaging technology, Rehabilitation robotics on November 17, 2023
Abstract:
Brain plasticity plays a significant role in functional recovery after stroke, but the specific benefits of hand rehabilitation robot therapy remain unclear. Evaluating the specific effects of hand rehabilitation robot therapy is crucial in understanding how it impacts brain activity and its relationship to rehabilitation outcomes. This study aimed to investigate the brain activity pattern during hand rehabilitation exercise using functional magnetic resonance imaging (fMRI), and to compare it before and after 3-week hand rehabilitation robot training. To evaluate it, an fMRI experimental environment was constructed to facilitate the same hand posture used in rehabilitation robot therapy. Two stroke survivors participated and the conjunction analysis results from fMRI scans showed that patient 1 exhibited a significant improvement in activation profile after hand rehabilitation robot training, indicative of improved motor function in the bilateral motor cortex. However, activation profile of patient 2 exhibited a slight decrease, potentially due to habituation to the rehabilitation task. Clinical results supported these findings, with patient 1 experiencing a greater increase in FMA score than patient 2. These results suggest that hand rehabilitation robot therapy can induce different brain activity patterns in stroke survivors, which may be linked to patient-specific training outcomes. Further studies with larger sample sizes are necessary to confirm these findings.
[Abstract] Understanding Characteristics of User Adherence to Optimize the Use of Home Hand Rehabilitation Technology
Posted by Kostas Pantremenos in Paretic Hand, Tele/Home Rehabilitation on November 17, 2023
Abstract:
Home-based rehabilitation can serve as an adjunct to in-clinic rehabilitation, encouraging users to engage in more practice. However, conventional home-based rehabilitation programs suffer from low adherence and high drop-out rates. Wearable movement sensors coupled with computer games can be more engaging, but have highly variable adherence rates. Here we examined characteristics of user adherence by analyzing unsupervised, wearable grip sensor-based home-hand rehabilitation data from 1,587 users. We defined three different classes of users based on activity level: low users (<2 days), medium users (2 – 9 days), and power users (> 9 days). The probability of using the device more than two days was positively correlated with first day game success (p = 0.91, p<. 001), and number of sessions played on the first day (p = 0.87, p<. 001) but negatively correlated with parameter exploration (total number of game adjustments / total number of sessions played) on the first day (p = – 0.31, p= 0.05). Compared to low users, power users on the first day had more game success (65.18 ± 25.76 %vs. 54.94 ± 30.31 %,p <. 001), parameter exploration (25.47 ± 22.78 % vs. 12.05 ± 20.56 %, p <. 001), and game sessions played (7.60 ± 6.59 sessions vs. 4.04 ± 3.56 sessions, p <. 001). These observations support the premise that initial game success which is modulated by strategically adjusting parameters when necessary is a key determinant of adherence to rehabilitation technology.
[Abstract + References] A new adaptive VR-based exergame for hand rehabilitation after stroke
Posted by Kostas Pantremenos in Paretic Hand, REHABILITATION, Video Games/Exergames, Virtual reality rehabilitation on October 18, 2023
Abstract
The aim of this work is to present an adaptive serious game based on virtual reality (VR) for functional rehabilitation of the hand after stroke. The game focuses on simulating the palmar grasping exercise commonly used in clinical settings. The system’s design follows a user-centered approach, involving close collaboration with functional rehabilitation specialists and stroke patients. It uses the Leap motion controller to enable patient interaction in the virtual environment, which was created using the Unity 3D game engine. The system relies on hand gestures involving opening and closing movements to interact with virtual objects. It incorporates parameters to objectively measure participants’ performance throughout the game session. These metrics are used to personalize the game’s difficulty to each patient’s motor skills. To do this, we implemented an approach that dynamically adjusts the difficulty of the exergame according to the patient’s performance during the game session. To achieve this, we used an unsupervised machine learning technique known as clustering, in particular using the K-means algorithm. By applying this technique, we were able to classify patients’ performance into distinct groups, enabling us to assess their skill level and adapt the difficulty of the game accordingly. To evaluate the system’s effectiveness and reliability, we conducted a subjective evaluation involving 11 stroke patients. The standardized System Usability Scale (SUS) questionnaire was used to assess the system’s ease of use, while the Intrinsic Motivation Inventory (IMI) was used to evaluate the participants’ subjective experience with the system. Evaluations showed that our proposed system is usable and acceptable on a C-level scale, with a good adjective score, and the patients perceived a high intrinsic motivation.
References
- Montoya, M.F., Munoz, J.E., Henao, O.A.: Enhancing virtual rehabilitation in upper limbs with biocybernetic adaptation: the effects of virtual reality on perceived muscle fatigue, game performance and user experience. IEEE Trans. Neural Syst. Rehabil. Eng. 28, 740–747 (2020). https://doi.org/10.1109/TNSRE.2020.2968869Article Google Scholar
- Then, J.W., Shivdas, S., Tunku Ahmad Yahaya, T.S., Ab Razak, N.I., Choo, P.T.: Gamification in rehabilitation of metacarpal fracture using cost-effective end-user device: a randomized controlled trial. J. Hand Therap. 33, 235–242 (2020). https://doi.org/10.1016/j.jht.2020.03.029
- Goršič, M., Cikajlo, I., Novak, D.: Competitive and cooperative arm rehabilitation games played by a patient and unimpaired person: effects on motivation and exercise intensity. J NeuroEng. Rehabil. 14, 23 (2017). https://doi.org/10.1186/s12984-017-0231-4Article Google Scholar
- Alarcón-Aldana, A.C., Callejas-Cuervo, M., Bo, A.P.L.: Upper limb physical rehabilitation using serious videogames and motion capture systems: a systematic review. Sensors. 20, 5989 (2020). https://doi.org/10.3390/s20215989Article Google Scholar
- Bortone, I., Leonardis, D., Solazzi, M., Procopio, C., Crecchi, A., Bonfiglio, L., Frisoli, A.: Integration of serious games and wearable haptic interfaces for Neuro Rehabilitation of children with movement disorders: A feasibility study. In: 2017 International Conference on Rehabilitation Robotics (ICORR). pp. 1094–1099. IEEE, London (2017)
- Avola, D., Cinque, L., Foresti, G.L., Marini, M.R.: An interactive and low-cost full body rehabilitation framework based on 3D immersive serious games. J. Biomed. Inform. 89, 81–100 (2019). https://doi.org/10.1016/j.jbi.2018.11.012Article Google Scholar
- Ribé, J.T., Serrancolí, G., Arriaga, I.A., Alpiste, F., Iriarte, A., Margelí, C., Mayans, B., García, A., Morancho, S., Molas, C.: Design and Development of Biomedical Applications for Post-Stroke Rehabilitation Subjects
- Ferreira, B., Menezes, P.: An Adaptive Virtual Reality-Based Serious Game for Therapeutic Rehabilitation. Int. J. Onl. Eng. 16, 63 (2020). https://doi.org/10.3991/ijoe.v16i04.11923Article Google Scholar
- Pillai, M., Yang, Y., Ditmars, C., Subhash, H.M.: Artificial intelligence-based interactive virtual reality-assisted gaming system for hand rehabilitation. In: Deserno, T.M. and Chen, P.-H. (eds.) Medical Imaging 2020: Imaging Informatics for Healthcare, Research, and Applications. p. 18. SPIE, Houston, United States (2020)
- Piette, P., Pasquier, J.: Réalité virtuelle et rééducation. Kinésithérapie, la Revue. 12, 38–41 (2012). https://doi.org/10.1016/j.kine.2012.07.003Article Google Scholar
- Kim, W.-S., Cho, S., Ku, J., Kim, Y., Lee, K., Hwang, H.-J., Paik, N.-J.: Clinical Application of Virtual Reality for Upper Limb Motor Rehabilitation in Stroke: Review of Technologies and Clinical Evidence. JCM. 9, 3369 (2020). https://doi.org/10.3390/jcm9103369Article Google Scholar
- Ning, H., Wang, Z., Li, R., Zhang, Y., Mao, L.: A Review on Serious Games for Exercise Rehabilitation. arXiv:2201.04984 [cs]. (2022)
- Amorim, P., Sousa Santos, B., Dias, P., Silva, S., Martins, H.: Serious Games for Stroke Telerehabilitation of Upper Limb – A Review for Future Research. Int J Telerehab. 12, 65–76 (2020). https://doi.org/10.5195/ijt.2020.6326Article Google Scholar
- Lawrence, E.S., Coshall, C., Dundas, R., Stewart, J., Rudd, A.G., Howard, R., Wolfe, C.D.A.: Estimates of the Prevalence of Acute Stroke Impairments and Disability in a Multiethnic Population. Stroke 32, 1279–1284 (2001). https://doi.org/10.1161/01.STR.32.6.1279Article Google Scholar
- Avola, D., Cinque, L., Pannone, D.: Design of a 3D Platform for Immersive Neurocognitive Rehabilitation. Information 11, 134 (2020). https://doi.org/10.3390/info11030134Article Google Scholar
- Zahabi, M., Abdul Razak, A.M.: Adaptive virtual reality-based training: a systematic literature review and framework. Virtual Reality 24, 725–752 (2020). https://doi.org/10.1007/s10055-020-00434-wArticle Google Scholar
- Debnath, B., O’Brien, M., Yamaguchi, M., Behera, A.: A review of computer vision-based approaches for physical rehabilitation and assessment. Multimedia Syst. 28, 209–239 (2022). https://doi.org/10.1007/s00530-021-00815-4Article Google Scholar
- Rincon, A.L., Yamasaki, H., Shimoda, S.: Design of a video game for rehabilitation using motion capture, EMG analysis and virtual reality. In: 2016 International Conference on Electronics. Communications and Computers (CONIELECOMP), pp. 198–204. IEEE, Cholula, Mexico (2016)
- Trombetta, M., Bazzanello Henrique, P.P., Brum, M.R., Colussi, E.L., De Marchi, A.C.B., Rieder, R.: Motion Rehab AVE 3D: A VR-based exergame for post-stroke rehabilitation. Computer Methods and Programs in Biomedicine. 151, 15-20 (2017). https://doi.org/10.1016/j.cmpb.2017.08.008
- Hashim, S.H.M., Ismail, M., Manaf, H., Hanapiah, F.A.: Development of Dual Cognitive Task Virtual Reality Game Addressing Stroke Rehabilitation. In: Proceedings of the 2019 3rd International Conference on Virtual and Augmented Reality Simulations. pp. 21-25. ACM, Perth WN Australia (2019
- Ma, M., Zheng, H.: Virtual Reality and Serious Games in Healthcare. In: Brahnam, S. and Jain, L.C. (eds.) Advanced Computational Intelligence Paradigms in Healthcare 6. Virtual Reality in Psychotherapy, Rehabilitation, and Assessment. pp. 169-192. Springer Berlin Heidelberg, Berlin, Heidelberg (2011)
- Gonçalves, A.R., Muñoz, J.E., Gouveia, É.R., Cameirão, M. da S., Bermúdez i Badia, S.: Effects of prolonged multidimensional fitness training with exergames on the physical exertion levels of older adults. Vis Comput. 37, 19-30 (2021). https://doi.org/10.1007/s00371-019-01736-0
- Hossain, M.S., Hardy, S., Alamri, A., Alelaiwi, A., Hardy, V., Wilhelm, C.: AR-based serious game framework for post-stroke rehabilitation. Multimedia Syst. 22, 659–674 (2016). https://doi.org/10.1007/s00530-015-0481-6Article Google Scholar
- Streicher, A., Smeddinck, J.D.: Personalized and Adaptive Serious Games. In: Dörner, R., Göbel, S., Kickmeier-Rust, M., Masuch, M., Zweig, K. (eds.) Entertainment Computing and Serious Games, pp. 332–377. Springer International Publishing, Cham (2016)Chapter Google Scholar
- Hocine, N., Gouaïch, A., Cerri, S.A., Mottet, D., Froger, J., Laffont, I.: Adaptation in serious games for upper-limb rehabilitation: an approach to improve training outcomes. User Model. User-Adap. Inter. 25, 65–98 (2015). https://doi.org/10.1007/s11257-015-9154-6Article Google Scholar
- Thielbar, K.O., Lord, T.J., Fischer, H.C., Lazzaro, E.C., Barth, K.C., Stoykov, M.E., Triandafilou, K.M., Kamper, D.G.: Training finger individuation with a mechatronic-virtual reality system leads to improved fine motor control post-stroke. J NeuroEngineering Rehabil. 11, 171 (2014). https://doi.org/10.1186/1743-0003-11-171Article Google Scholar
- Sinaga, K.P., Yang, M.-S.: Unsupervised K-Means Clustering Algorithm. IEEE. Access 8, 80716–80727 (2020). https://doi.org/10.1109/ACCESS.2020.2988796Article Google Scholar
- Colombo, R., Pisano, F., Micera, S., Mazzone, A., Delconte, C., Carrozza, M.C., Dario, P., Minuco, G.: Robotic techniques for upper limb evaluation and rehabilitation of stroke patients. IEEE Trans. Neural Syst. Rehabil. Eng. 13, 311–324 (2005). https://doi.org/10.1109/TNSRE.2005.848352Article Google Scholar
- Sunderland, A., Tinson, D., Bradley, L., Hewer, R.L.: Arm function after stroke. An evaluation of grip strength as a measure of recovery and a prognostic indicator. Journal of Neurology, Neurosurgery & Psychiatry. 52, 1267-1272 (1989). https://doi.org/10.1136/jnnp.52.11.1267
- Kim, H., Miller, L.M., Fedulow, I., Simkins, M., Abrams, G.M., Byl, N., Rosen, J.: Kinematic Data Analysis for Post-Stroke Patients Following Bilateral Versus Unilateral Rehabilitation With an Upper Limb Wearable Robotic System. IEEE Trans. Neural Syst. Rehabil. Eng. 21, 153–164 (2013). https://doi.org/10.1109/TNSRE.2012.2207462Article Google Scholar
- Kong, K.-H., Lee, J.: Temporal recovery and predictors of upper limb dexterity in the first year of stroke: A prospective study of patients admitted to a rehabilitation centre. NRE. 32, 345–350 (2013). https://doi.org/10.3233/NRE-130854Article Google Scholar
- Bigoni, M., Baudo, S., Cimolin, V., Cau, N., Galli, M., Pianta, L., Tacchini, E., Capodaglio, P., Mauro, A.: Does kinematics add meaningful information to clinical assessment in post-stroke upper limb rehabilitation? A case report. J Phys Ther Sci. 28, 2408–2413 (2016). https://doi.org/10.1589/jpts.28.2408
- Sucar, L.E., Luis, R., Leder, R., Hernáindez, J., Sáinchez, I.: Gesture therapy: A vision-based system for upper extremity stroke rehabilitation. In: 2010 Annual International Conference of the IEEE Engineering in Medicine and Biology. pp. 3690-3693. IEEE, Buenos Aires (2010)
- Housman, S.J., Scott, K.M., Reinkensmeyer, D.J.: A Randomized Controlled Trial of Gravity-Supported, Computer-Enhanced Arm Exercise for Individuals With Severe Hemiparesis. Neurorehabil. Neural Repair 23, 505–514 (2009). https://doi.org/10.1177/1545968308331148Article Google Scholar
- Kong, K.-H., Loh, Y.-J., Thia, E., Chai, A., Ng, C.-Y., Soh, Y.-M., Toh, S., Tjan, S.-Y.: Efficacy of a Virtual Reality Commercial Gaming Device in Upper Limb Recovery after Stroke: A Randomized. Controlled Study. Topics in Stroke Rehabilitation. 23, 333–340 (2016). https://doi.org/10.1080/10749357.2016.1139796Article Google Scholar
- Rand, D., Givon, N., Weingarden, H., Nota, A., Zeilig, G.: Eliciting Upper Extremity Purposeful Movements Using Video Games: A Comparison With Traditional Therapy for Stroke Rehabilitation. Neurorehabil. Neural Repair 28, 733–739 (2014). https://doi.org/10.1177/1545968314521008Article Google Scholar
- Broeren, J., Claesson, L., Goude, D., Rydmark, M., Sunnerhagen, K.S.: Virtual Rehabilitation in an Activity Centre for Community-Dwelling Persons with Stroke. Cerebrovasc. Dis. 26, 289–296 (2008). https://doi.org/10.1159/000149576Article Google Scholar
- Sin, H., Lee, G.: Additional Virtual Reality Training Using Xbox Kinect in Stroke Survivors with Hemiplegia. American Journal of Physical Medicine & Rehabilitation. 92, 871–880 (2013). https://doi.org/10.1097/PHM.0b013e3182a38e40Article Google Scholar
- Kwon, J.-S., Park, M.-J., Yoon, I.-J., Park, S.-H.: Effects of virtual reality on upper extremity function and activities of daily living performance in acute stroke: A double-blind randomized clinical trial. NRE. 31, 379–385 (2012). https://doi.org/10.3233/NRE-2012-00807Article Google Scholar
- Lee, S., Kim, Y., Lee, B.-H.: Effect of Virtual Reality-based Bilateral Upper Extremity Training on Upper Extremity Function after Stroke: A Randomized Controlled Clinical Trial: Bilateral Upper Extremity Training in Post Stroke. Occup. Ther. Int. 23, 357–368 (2016). https://doi.org/10.1002/oti.1437Article Google Scholar
- Subramanian, S.K., Lourenço, C.B., Chilingaryan, G., Sveistrup, H., Levin, M.F.: Arm Motor Recovery Using a Virtual Reality Intervention in Chronic Stroke: Randomized Control Trial. Neurorehabil. Neural Repair 27, 13–23 (2013). https://doi.org/10.1177/1545968312449695Article Google Scholar
- Zheng, C., Liao, W., Xia, W.: Effect of combined low-frequency repetitive transcranial magnetic stimulation and virtual reality training on upper limb function in subacute stroke: a double-blind randomized controlled trail. J. Huazhong Univ. Sci. Technol. [Med. Sci.]. 35, 248-254 (2015). https://doi.org/10.1007/s11596-015-1419-0
- Piron, L., Turolla, A., Agostini, M., Zucconi, C., Cortese, F., Zampolini, M., Zannini, M., Dam, M., Ventura, L., Battauz, M., Tonin, P.: Exercises for paretic upper limb after stroke: A combined virtual-reality and telemedicine approach. J. Rehabil. Med. 41, 1016–102 (2009). https://doi.org/10.2340/16501977-0459Article Google Scholar
- Brunner, I., Skouen, J.S., Hofstad, H., Aßmus, J., Becker, F., Sanders, A.-M., Pallesen, H., Qvist Kristensen, L., Michielsen, M., Thijs, L., Verheyden, G.: Virtual Reality Training for Upper Extremity in Subacute Stroke (VIRTUES): A multicenter RCT. Neurology 89, 2413–2421 (2017). https://doi.org/10.1212/WNL.0000000000004744Article Google Scholar
- Choi, Y.-H., Ku, J., Lim, H., Kim, Y.H., Paik, N.-J.: Mobile game-based virtual reality rehabilitation program for upper limb dysfunction after ischemic stroke. RNN. 34, 455–463 (2016). https://doi.org/10.3233/RNN-150626Article Google Scholar
- Kiper, P., Agostini, M., Luque-Moreno, C., Tonin, P., Turolla, A.: Reinforced Feedback in Virtual Environment for Rehabilitation of Upper Extremity Dysfunction after Stroke: Preliminary Data from a Randomized Controlled Trial. Biomed. Res. Int. 2014, 1–8 (2014). https://doi.org/10.1155/2014/752128Article Google Scholar
- Ma, M., Bechkoum, K.: Serious games for movement therapy after stroke. In: 2008 IEEE International Conference on Systems. Man and Cybernetics, pp. 1872–1877. IEEE, Singapore, Singapore (2008)
- Nasri, N., Orts-Escolano, S., Cazorla, M.: An sEMG-Controlled 3D Game for Rehabilitation Therapies: Real-Time Time Hand Gesture Recognition Using Deep Learning Techniques. Sensors. 20, 6451 (2020). https://doi.org/10.3390/s20226451Article Google Scholar
- Dhiman, A., Solanki, D., Bhasin, A., Das, A., Lahiri, U.: An intelligent, adaptive, performance-sensitive, and virtual reality-based gaming platform for the upper limb. Comput Anim Virtual Worlds. 29, e1800 (2018). https://doi.org/10.1002/cav.1800Article Google Scholar
- Vallejo, D., Gmez-Portes, C., Albusac, J., Glez-Morcillo, C., Castro-Schez, J.J.: Personalized Exergames Language: A Novel Approach to the Automatic Generation of Personalized Exergames for Stroke Patients. Appl. Sci. 10, 7378 (2020). https://doi.org/10.3390/app10207378Article Google Scholar
- Afyouni, I., Murad, A., Einea, A.: Adaptive Rehabilitation Bots in Serious Games. Sensors. 20, 7037 (2020). https://doi.org/10.3390/s20247037Article Google Scholar
- Aguilar-Lazcano, C.A., Rechy-Ramirez, E.J.: Performance analysis of Leap motion controller for finger rehabilitation using serious games in two lighting environments. Measurement 157, 107677 (2020). https://doi.org/10.1016/j.measurement.2020.107677Article Google Scholar
- Rechy-Ramirez, E.J., Marin-Hernandez, A., Rios-Figueroa, H.V.: A human-computer interface for wrist rehabilitation: a pilot study using commercial sensors to detect wrist movements. Vis Comput. 35, 41–55 (2019). https://doi.org/10.1007/s00371-017-1446-xArticle Google Scholar
- Burke, J.W., McNeill, M.D.J., Charles, D.K., Morrow, P.J., Crosbie, J.H., McDonough, S.M.: Optimising engagement for stroke rehabilitation using serious games. Vis Comput. 25, 1085–1099 (2009). https://doi.org/10.1007/s00371-009-0387-4Article Google Scholar
- de los Reyes-Guzmàin, A., Lozano-Berrio, V., Alvarez-Rodríguez, M., López-Dolado, E., Ceruelo-Abajo, S., Talavera-Díaz, F., Gil-Agudo, A.: RehabHand: Oriented-tasks serious games for upper limb rehabilitation by using Leap Motion Controller and target population in spinal cord injury. NeuroRehabilitation 48(3), 365–373 (2021). https://doi.org/10.3233/NRE-201598
- Harvey, C., Selmanović, E., O’Connor, J., Chahin, M.: A comparison between expert and beginner learning for motor skill development in a virtual reality serious game. Vis Comput. 37, 3–17 (2021). https://doi.org/10.1007/s00371-019-01702-wArticle Google Scholar
- David, L., Bouyer, G., Otmane, S.: Towards a low-cost interactive system for motor self-rehabilitation after stroke. IJVR. 17, 40-45 (2017). https://doi.org/10.20870/IJVR.2017.17.2.2890
- Li, C., Cheng, L., Yang, H., Zou, Y., Huang, F.: An Automatic Rehabilitation Assessment System for Hand Function Based on Leap Motion and Ensemble Learning. Cybern. Syst. 52, 3–25 (2021). https://doi.org/10.1080/01969722.2020.1827798Article Google Scholar
- de Souza, M.R.S.B., Gonçalves, R.S., Carbone, G.: Feasibility and Performance Validation of a Leap Motion Controller for Upper Limb Rehabilitation. Robotics 10, 130 (2021). https://doi.org/10.3390/robotics10040130Article Google Scholar
- Koter, K., Samowicz, M., Redlicka, J., Zubrycki, I.: Hand Measurement System Based on Haptic and Vision Devices towards Post-Stroke Patients. 23 (2022)
- David, L.: Conception et évaluation d’un système de réalité virtuelle pour l’assistance à l’auto-rééducation motrice du membre supérieur post-AVC. 165
- Orhan, U., Hekim, M., Ozer, M.: EEG signals classification using the K-means clustering and a multilayer perceptron neural network model. Expert Syst. Appl. 38, 13475–13481 (2011). https://doi.org/10.1016/j.eswa.2011.04.149Article Google Scholar
- Bangor, A.: Determining What Individual SUS Scores Mean: Adding an Adjective Rating Scale. 4, 10 (2009)Google Scholar
- Franck, J.A., Timmermans, A.A.A., Seelen, H.A.M.: Effects of a dynamic hand orthosis for functional use of the impaired upper limb in sub-acute stroke patients: A multiple single case experimental design study. TAD. 25, 177–187 (2013). https://doi.org/10.3233/TAD-130374Article Google Scholar
Source: https://link.springer.com/article/10.1007/s00530-023-01180-0