Posts Tagged hand rehabilitation
[Abstract] Hybrid Brain-Computer Interface Controlled Soft Robotic Glove for Stroke Rehabilitation
Posted by Kostas Pantremenos in Paretic Hand, Rehabilitation robotics on April 26, 2024
Abstract:
Soft robotic glove controlled by a brain-computer interface (BCI) have demonstrated effectiveness in hand rehabilitation for stroke patients. Current systems mostly rely on static visual representations for patients to perform motor imagination (MI) tasks, resulting in lower BCI performance. Therefore, this study innovatively used MI and high-frequency steady-state visual evoked potential (SSVEP) to construct a friendly and natural hybrid BCI paradigm. Specifically, the stimulation interface sequentially presented decomposed action pictures of the left and right hands gripping a ball, with the pictures flashing at specific stimulation frequencies (left: 34 Hz, right: 35 Hz). Integrating soft robotic glove as feedback, we established a comprehensive “peripheral – central – peripheral” hand rehabilitation system to facilitate the hand rehabilitation of patients. Filter bank common spatial pattern (FBCSP) and filter bank canonical correlation analysis (FBCCA) algorithms were used to identify MI and SSVEP signals, respectively. Additionally, to fuse the features of these two signals, we proposed a novel fusion algorithm for improving the recognition accuracy of the system. The feasibility of the proposed system was validated through online experiments involving 12 healthy subjects and 9 stroke patients, achieving accuracy rates of 95.83 ± 6.83% and 63.33 ± 10.38%, respectively. The accuracy of MI and SSVEP in 12 healthy subjects reached 81.67 ± 15.63% and 95.14 ± 7.47%, both lower than the accuracy after fusion, these results confirmed the effectiveness of the proposed algorithm. The accuracy rate was more than 50% in both healthy subjects and patients, confirming the effectiveness of the proposed system.
[Abstract] A Review on Soft Exoskeletons for Hand Rehabilitation
Posted by Kostas Pantremenos in Paretic Hand, Rehabilitation robotics on February 24, 2024
Background: How to enhance the quality of life for the elderly has emerged as a key issue in many nations due to the ageing population. Stroke is the most prevalent disease among the elderly; specifically, the hand dysfunction caused by stroke is also a powerful obstacle to the daily life of the elderly. Soft Exoskeletons for Hand Rehabilitation (SEHRs) have become a major trend for the future due to the increasing demand for hand rehabilitation.
Objective: To provide a reference for readers in this field by introducing the most recent research developments in the field of SEHR, including their classification and properties.
Methods: By reviewing different types of hand rehabilitation exoskeleton research papers and patents, the advantages and disadvantages, differences, and applications of various SEHRs were summarized.
Results: According to the driving mode and realizing the function of SEHRs, the structure characteristics of SEHRs are analyzed and compared. The key problems and future development trends of SEHRs were expounded.
Conclusion: According to the driving method, the research shows that SEHRs can be divided into Air-Driven Soft Exoskeletons for Hand Rehabilitation (ADSEHRs), Motor-Driven Soft Exoskeletons for Hand Rehabilitation (MDSEHRs), and Hybrid-Driven Soft Exoskeletons for Hand Rehabilitation (HDSEHRs). Future research is required to further optimize the flexibility and adaptability of soft exoskeletons, improve their accuracy and sensitivity, and enhance human-machine interaction with the human hand.
[ARTICLE] Lightweight Soft Robotic Glove with Whole-Hand Finger Motion Tracking for Hand Rehabilitation in Virtual Reality – Full Text
Posted by Kostas Pantremenos in Paretic Hand, Rehabilitation robotics, Virtual reality rehabilitation on September 22, 2023
Abstract
Soft robotic gloves have attracted significant interest in hand rehabilitation in the past decade. However, current solutions are still heavy and lack finger-state monitoring and versatile treatment options. To address this, we present a lightweight soft robotic glove actuated by twisted string actuators (TSA) that provides whole-hand finger motion tracking. We have developed a virtual reality environment for hand rehabilitation training, allowing users to interact with various virtual objects. Fifteen small inertial measurement units are placed on the glove to predict finger joint angles and track whole-hand finger motion. We performed TSA experiments to identify design and control rules, by understanding how their response varies with input load and voltages. Grasping experiments were conducted to determine the grasping force and range of motion. Finally, we showcase an application of the rehabilitation glove in a Unity-based VR interface, which can actuate the operator’s fingers to grasp different virtual objects.
1. Introduction
Active rehabilitation training can benefit patients with hand injuries, neurological or musculoskeletal conditions, and those who have undergone hand surgery by improving muscle strength and overall physical condition. However, traditional training with a physiotherapist is expensive, and most physiotherapy mechanical devices only allow patients to perform simple movement training, which is often perceived as monotonous and boring, leading to reduced patient motivation. Moreover, these rehabilitation devices are mostly bulky and heavy, so are not suitable for home rehabilitation. Therefore, there is a need for a low-cost, portable, and entertaining rehabilitation glove.
One widely used technology in rehabilitation equipment is that of exoskeletons. These can be categorized into hard exoskeletons, which use rigid joints, links, and motors to drive finger movements, and flexible exoskeletons, which are designed around a glove for improved wearability. Hard exoskeletons [1,2] provide high force output but their size and weight make them inconvenient and pose a risk of secondary injury. Some research has been undertaken on hand/wrist exoskeletons [3], but they are still too bulky and heavy.
To address the limitations of hard exoskeletons, soft exoskeletons designed as gloves with peripheral actuation are a suitable solution. These exoskeletons are lightweight and comfortable to wear. Soft exoskeletons can be categorized based on actuation sources, among which pneumatic and motor-tendon-based actuation methods are the two most popular types. Current pneumatic-driven hand exoskeletons [4,5,6] normally have a pneumatic chamber made of silicone or other flexible material, which is fixed to the back of the fingers of the glove and bends upon inflation of the pneumatic pump, thus bending the fingers. Although it is simple to control, this method has the drawback of bulkiness due to the air pump drive source and pneumatic chamber. In addition, the bulky actuators on the fingers may cause interference between fingers upon actuation. In contrast, the motor-tendon driven method is much lighter, where a small motor can be connected to the fingertip via a tendon and the finger can be driven by the rotation of the motor [7,8,9]. This method has a much simpler glove design, which makes the hand-wearable part more comfortable and lighter. Twisted string actuators (TSA) [10,11] are a type of motor-tendon actuator that converts a motor’s rotational motion into linear motion using a twisted string or wire. Compared to regular motor-tendon actuators, this method can provide five times the actuation force of spool-based motor-tendon actuators, which allows for using smaller motors as actuation sources [12], resulting in a much smaller robotic glove system. Tsabedze et al. [12,13] developed soft robotic gloves for rehabilitation purposes that are small in size and lightweight. Hosseini et al. [14] developed a force-feedback haptic glove using two independent TSAs and force sensors. However, these TSA gloves are mainly initial demonstration versions lacking posture-sensing functionality. Robotic gloves without posture-sensing capability cannot sense the finger state or record the rehabilitation process, making it difficult for robotic control and for doctors to assess a patient’s recovery progress.
Data gloves with hand movement tracking are effective for assessing hand training and developing control strategies for rehabilitation. Various sensors have been investigated for use in data gloves, such as flexible bending sensors, inertial measurement units (IMUs), etc. Flexible bending sensors are highly resistive sensors that have the advantage of being lightweight, small and inexpensive. However, such sensors can be easily damaged and their costs can be high [15]. IMUs are accurate and inexpensive and can collect information on each joint by placing a corresponding sensor on each finger segment. A number of IMU-based data gloves have been developed [16,17,18,19] and have shown high accuracy for hand motion tracking.
The monotonous and repetitive nature of hand rehabilitation training can be demotivating for patients undergoing physical therapy. However, the introduction of hand VR rehabilitation systems has revolutionized the process by adding an element of fun to the training. Additionally, these systems allow patients to train at home, making it more convenient for them [20,21]. Pereira et al. [22] developed a VR game called StableHand, which features farm-based scenarios that provide contextualized elements to support rehabilitation tasks. The game received a high system usability score (SUS) of 84.3 and positive feedback from seven participants, indicating its potential to enhance hand rehabilitation therapy.
In this study, we propose a VR rehabilitation training glove system. This glove can drive the users to grasp various virtual objects while the users can visualize their grasping in a virtual environment. This glove has two main functions: actuation-assisted rehabilitation and real-time gesture recognition. To assist with grip training, a soft robotic glove was developed using five TSAs. Each TSA is composed of a motor and a pair of strings on the arm. The TSAs apply tension to the fingertips, yielding finger bending and helping the patient with their grip exercises. Elastic bands are attached to the back of the glove to help restore the patient’s fingers to their initial state after training. For finger gesture recognition, 15 IMUs are placed on the back of the glove. Every three IMUs are connected by one flexible printed circuit (FPC) board. The finger posture angles are solved through static threshold correction and a complementary filtering algorithm. Finally, we utilize a VR rehabilitation training system with objects in different shapes for grasping, in which the patient’s hand is mapped in real-time. The VR rehabilitation training glove is a low-cost device that costs about USD150. The proposed glove system can provide patients with more attractive, personalized, and effective rehabilitation training therapy with the help of rehabilitation training theory and VR, which can enhance patients’ initiative and motivation and improve the effectiveness of rehabilitation training.
2. Glove System Design and Experimental Testing
2.1. Overview
Figure 1 shows an overview of the proposed VR rehabilitation training glove system. This system comprises two main subsystems: a finger posture tracking system and a twisted string actuation (TSA) system. The finger posture tracking system utilizes fifteen IMUs to monitor the real-time angles of each finger joint and is referred to as the IMU system later in the text. These angle data are transmitted to the VR system, which uses a pre-built virtual hand to simulate the physical hand based on the recorded angles. The VR system also detects collisions between the virtual hand and objects in the virtual scene. When there is no collision, the TSA system continuously drives the twisted wires, converting the motor’s rotation into linear tension on the tendons to facilitate the patient’s gripping. Conversely, when a collision is detected, the TSA system stops functioning.
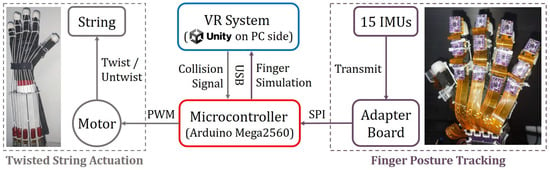
Figure 1. Overview of the proposed system.
[…]
[ARTICLE] BCI–VR-Based Hand Soft Rehabilitation System with Its Applications in Hand Rehabilitation After Stroke – Full Text
Posted by Kostas Pantremenos in Paretic Hand, REHABILITATION, Virtual reality rehabilitation on June 13, 2023
Abstract
The recovery of hand motor function can effectively improve the living standard of stroke patients and relieve their psychological anxiety. Traditional physical rehabilitation training is unable to target the cause of motor function loss; therefore, the rehabilitation effect is not ideal. The objective of this study is to propose a hand rehabilitation system combining brain–computer interface (BCI), soft hand rehabilitation glove and virtual reality (VR), and explore its effectiveness on hand movement disorders in stroke patients. The corresponding comparison experiments conducted on 11 stroke patients demonstrated that the proposed BCI-based hand rehabilitation system can not only mobilize more cerebral cortex to participate in the process of hand motor rehabilitation, but also enhance the muscle strength, muscle tension, and improve the hand motor dysfunction of stroke patients.
1 Introduction
The number of elderly people suffering from vascular disorders has increased rapidly in developed countries. It has become a social problem, with consequences of paralysis and worsening living conditions. Many diseases can cause paralysis, for example, stroke, spinal cord injury (SCI), amyotrophic lateral sclerosis, and multiple sclerosis, in which stroke accounts for the largest proportion. According to the report of the World Health Organization (WHO) in 2016, stroke is now the second leading cause of death in the world. Most poststroke patients experience partial paralysis, which occurs mainly in the upper limbs, especially the hands [1, 2]. Ghassemi et al. [3] research has shown that six months after the onset of stroke, about 65% of stroke patients still have hand dysfunction, only 15% of stroke patients can recover about half of their hand function, and only 3% of patients are able to recover more than 70% of their original functions [4].
Stroke treatment for hand rehabilitation may require different equipment and methods, but physiotherapy (PT) is a central component of the rehabilitation process. Improving hand function requires repetitive task practice rehabilitation, which includes breaking tasks down into individual movements and practicing them to improve hand strength, accuracy, and range of motion. Constraint-induced motor therapy (CIMT), neuromuscular stimulation (NMS) and mental practice with motor imagery are some of the most common treatments for the rehabilitation of paraplegic hands after stroke, and their efficacy has been well established [5, 6]. However, these techniques have some important limitations, especially for patients in chronic stages. For example, nearly 50 percent of chronic patients with severe functional affectation do not experience improvement with CIMT, and residual motor activity is necessary for CIMT; therefore, CIMT is not suitable for stroke patients with severe limb weakness [7, 8].[…]
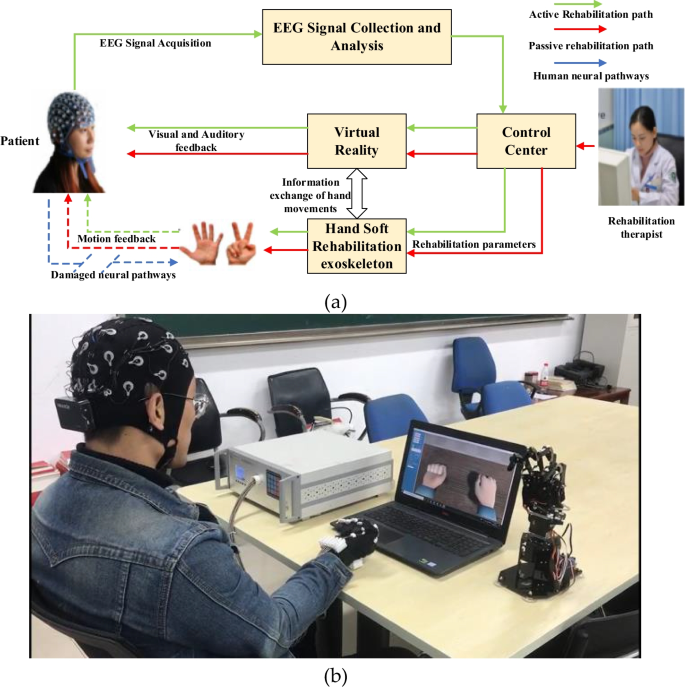
[ARTICLE] Commercial device-based hand rehabilitation systems for stroke patients: State of the art and future prospects – Full Text
Posted by Kostas Pantremenos in Paretic Hand, Video Games/Exergames on February 20, 2023
Abstract
Various hand rehabilitation systems have recently been developed for stroke patients, particularly commercial devices. Articles from 10 electronic databases from 2010 to 2022 were extracted to conduct a systematic review to explore the existing commercial training systems (hardware and software) and evaluate their clinical effectiveness. This review divided the rehabilitation equipment into contact and non-contact types. Game-based training protocols were further classified into two types: immersion and non-immersion. The results of the review indicated that the majority of the devices included were effective in improving hand function. Users who underwent rehabilitation training with these devices reported improvements in their hand function. Game-based training protocols were particularly appealing as they helped reduce boredom during rehabilitation training sessions. However, the review also identified some common technical drawbacks in the devices, particularly in non-contact devices, such as their vulnerability to the effects of light. Additionally, it was found that currently, there is no commercially available game-based training protocol that specifically targets hand rehabilitation. Given the ongoing COVID-19 pandemic, there is a need to develop safer non-contact rehabilitation equipment and more engaging training protocols for community and home-based rehabilitation. Additionally, the review suggests the need for revisions or the development of new clinical scales for hand rehabilitation evaluation that consider the current scenario, where in-person interactions might be limited.
1. Introduction
Stroke, also known as cerebrovascular accident (CVA), is a cerebral blood circulation disorder leading to a sudden loss of brain function [
]. According to the Global Burden of Disease Study 2016 (GBD2016) and 2017 (GBD2017), the incidence of stroke remains high. The mortality and disability-adjusted life-years (DALYs) caused by stroke reached 6.16 million deaths and 132 million DALYs in 2017, with a significant increase from 5.5 million deaths and 116 million DALYs in 2016 [
,
]. According to the GBD2019 Stroke Collaboration [
], stroke is still the third leading cause of death and disability globally. Among the countries, China bears the highest burden of stroke, with the DALYs caused by stroke ranking first in the world [
]. According to the “China Cardiovascular Health and Disease Report”, the national stroke population in China was 13 million in 2020 [
], and the morbidity and mortality rate continues to increase year by year [
], with an anticipated increase of 35% by 2050. Studies indicate that around 85% of stroke patients worldwide have hand dysfunction, with 60% still suffering from upper limb disorders, particularly in the fingers and wrists, after treatment and discharge [
]. Hand dysfunction causes difficulties in performing the most basic activities of daily living (ADLs), such as tying shoelaces, twisting towels, and picking up and manipulating objects, greatly impacting the individual’s quality of life [
].
The rehabilitation of fine hand activity is important for recovering upper limb function. Traditional treatment requires patients to receive regular and continuous physical therapies in professional rehabilitation institutions or hospitals [
]. The therapist assesses the patient’s conditions through observation, communication, questionnaires, and functional tests [
]. Current clinical scales used for upper limb assessment include the Fugl-Meyer Assessment (FMA) [
], Wolf Motor Function Test (WMFT) [
], Box and Block Test (BBT) [
], and Action Research Arm Test (ARAT) [
], and they have been validated through the existing reports. However, these scales are focused on upper limb movements rather than hand movements, and they are time-consuming, subjective, and inflexible [
]. For example, the Jebsen-Taylor Hand Function Test (JTHFT) [
] is a specific test for hand function, but it is not frequently updated, which raises doubts about its effectiveness. Conventional rehabilitation training is dependent on the therapist’s experience and can be labor-intensive and time-consuming; this can lead to a heavy work burden for physical therapists, which can affect the effectiveness of treatment [
]. Therefore, with the development of robotic and smart technologies, advanced rehabilitation devices and innovative approaches are emerging as alternative solutions to rehabilitation treatment.
Stroke rehabilitation is typically classified into three stages based on the level of the patient’s clinical manifestations: acute, recovery, and tertiary [
]. It can also be classified as mild, moderate, and severe based on the degree of injury. In the acute stage, patients are mostly unable to perform basic life skills independently and require professional rehabilitation staff to arrange occupational therapy (OT) [
]. Rehabilitation in this stage is primarily carried out in rehabilitation centers and may include the use of rehabilitation robots (e.g., powered exoskeleton or end-effect based rehabilitation robotics) and physiological signal-based equipment (e.g., brain-computer interface (BCI) and functional electrical stimulation (FES)) [
].
During the recovery and tertiary stages, the conditions of mild or moderate stroke patients are relatively stable, and they are eager to return home [
]. Rehabilitation in the community and at home is a more practical setting for post-rehabilitation, but providing patients with complicated and costly equipment in these settings is risky and impractical. This situation calls for the development of community and home-use rehabilitation devices or technology that are safe, easy to use and store, and low cost. Patients prefer non-contact devices as they are easier to use and highly adaptable. Additionally, non-contact devices do not need to be sterilized regularly, making them particularly practical during the COVID-19 pandemic [
].
Hand rehabilitation after a stroke is a long process, and motivation is crucial for the patient’s outcome [
]. The treatment outcome depends not only on the physical therapist’s rehabilitation process but also on the patient’s motivation for the training protocols [
]. To make rehabilitation more appealing, game-based training protocols are incorporated into the training system, such as 2D games, 3D games [
], virtual reality (VR) games [
], augmented reality (AR) games [
], etc. The use of games in training improves the enjoyment of rehabilitation, allowing patients to perform voluntary or active exercises and providing feedback and encouragement repeatedly [
]. It is worth noting that the use of virtual reality in game-based training protocols meets both the physiological and psychological needs of patients. VR creates a dynamic and motivating environment by merging touch, hearing, and vision, making patients more likely to participate in clinical or home training [
]. However, developing an effective rehabilitation game is challenging. Games that are too challenging for patients can be frustrating, and games that are too easy can quickly lose the patient’s interest [
].
Some patients may indeed experience failure and lack confidence when first playing training games for rehabilitation, which lead to decreased motivation to continue playing [
]. It is particularly true for stroke patients recovering from hand disorders, as there are currently limited options for games that are specifically designed to target these conditions. Some studies have shown positive outcomes from using games in rehabilitation [
], but more research is needed to develop games tailored to the specific needs of stroke patients with hand disorders.
Many hand rehabilitation devices and game-based training protocols have been reported, but most of them are self-made and have not been clinically tested. Meanwhile, few reviews have focused on the effectiveness of the existing commercial training systems with various game-based training protocols for hand rehabilitation. Therefore, this systematic review focused on evaluating the existing commercial training systems and the various game-based protocols used in hand rehabilitation. Furthermore, the review analyzed the hardware, game-based training protocols, and clinical outcomes. This review also provided future perspectives to guide the development of hand rehabilitation in the community and home settings during and after the COVID-19 pandemic.[…]
[ARTICLE] Sensorimotor Rhythm-Based Brain–Computer Interfaces for Motor Tasks Used in Hand Upper Extremity Rehabilitation after Stroke: A Systematic Review – Full Text
Posted by Kostas Pantremenos in Paretic Hand on February 4, 2023
by Jianghong Fu , Shugeng Chen , Jie Jia
Abstract
Brain–computer interfaces (BCIs) are becoming more popular in the neurological rehabilitation field, and sensorimotor rhythm (SMR) is a type of brain oscillation rhythm that can be captured and analyzed in BCIs. Previous reviews have testified to the efficacy of the BCIs, but seldom have they discussed the motor task adopted in BCIs experiments in detail, as well as whether the feedback is suitable for them. We focused on the motor tasks adopted in SMR-based BCIs, as well as the corresponding feedback, and searched articles in PubMed, Embase, Cochrane library, Web of Science, and Scopus and found 442 articles. After a series of screenings, 15 randomized controlled studies were eligible for analysis. We found motor imagery (MI) or motor attempt (MA) are common experimental paradigms in EEG-based BCIs trials. Imagining/attempting to grasp and extend the fingers is the most common, and there were multi-joint movements, including wrist, elbow, and shoulder. There were various types of feedback in MI or MA tasks for hand grasping and extension. Proprioception was used more frequently in a variety of forms. Orthosis, robot, exoskeleton, and functional electrical stimulation can assist the paretic limb movement, and visual feedback can be used as primary feedback or combined forms. However, during the recovery process, there are many bottleneck problems for hand recovery, such as flaccid paralysis or opening the fingers. In practice, we should mainly focus on patients’ difficulties, and design one or more motor tasks for patients, with the assistance of the robot, FES, or other combined feedback, to help them to complete a grasp, finger extension, thumb opposition, or other motion. Future research should focus on neurophysiological changes and functional improvements and further elaboration on the changes in neurophysiology during the recovery of motor function.
1. Introduction
Stroke causes the highest morbidity associated with disability-adjusted life years lost in China, with two million new cases annually [1]. Up to 66% of stroke survivors experience upper limb and hand motor impairments, which results in functional limitations in activities of daily living and decreased life quality [2,3], and leads to a heavy burden for the family and society. Hand rehabilitation after a stroke is difficult during neurorehabilitation. Traditional rehabilitation methods cannot fully meet the need of patients and the expectations of doctors [4]. Various methods were being applied in hand function rehabilitation, including central interventions such as mirror therapy, transcranial magnetic stimulation, transcranial direct current stimulation, brain–computer interfaces (BCIs), motor imagery, etc., peripheral interventions such as a robot, physical therapy, functional electrical stimulation, etc., and medicine such as botulinum for spasticity [5]. BCIs have been proven to be effective for hand motor recovery after stroke [6,7,8]. According to its working mechanism, BCIs can be classified as assistive or rehabilitative devices based on their clinical applications. In some laboratories, assistive BCIs are used as communication tools for amyotrophic lateral sclerosis patients [9,10] or as daily activity assistance for tetraplegia, such as drinking assistance [11,12]. Meanwhile, rehabilitative BCIs are mainly used in promoting functional recovery for such as stroke patients.
There were various kinds of rehabilitative BCIs equipment. In practice, the workflow of BCI is acquiring brain signals, extracting features, transforming the signal into command via external devices, and activating the sensory feedback. In non-invasive systems, BCIs involve brain activities measured by different kinds of equipment, such as electroencephalograph (EEG), functional magnetic resonance imaging, and functional near-infrared imaging, and the user’s movement intention such as motor imagery or motor attempt is decoded in real-time from the ongoing electrical activity of the brain by extracting relevant features [6]. Based on different features, such as common spatial pattern (CSP), and event-related desynchronization (ERD), different movement intentions can be classified by linear discriminant analysis (LDA) classifier, support vector machine. The algorithm converts the brain signals into information, then the external devices, such as the computer screen, robot, functional electrical stimulation (FES), or orthosis received the information and provide feedback to the subjects. The whole process forms a closed loop called neural feedback [13,14]. Auditory, visual, tactile, and proprioceptive feedback is commonly adopted in BCI, and their combination is used extensively in clinical experiments [15,16,17]. Motor imagery (MI), motor attempt (MA), or motor execution (ME) can activate several signal rhythm changes in the cerebral cortex [18,19], which can be captured and used to modulate the amplitude of sensorimotor rhythm (SMR) to control external devices. In addition, BCIs are a kind of active rehabilitation device. They achieved control of devices by catching the subject’s initiative. In particular, the motor task is not only the start factor for BCIs, but also the repeated training of task can promote motor recovery. Well-designed motor tasks and befitting feedback for the patients can enhance the BCI training and lead to a successful rehabilitation process.
Motor tasks usually concern the movement intention or the actual movement of the paretic limb. As we all know, hand recovery is a long and rough process. Several stroke patients in the acute stage can hardly move their hands, neither completely nor incompletely grasp, and they encounter kinds of difficulties, such as opening the fingers or moving their thumb or other fingers independently. The recovery of the hand function conforms to some rules, such as the six Brunnstrom recovery stages [20], but many of the hand functions stagnate at some specific stages. According to Brunnstrom recovery stage for stroke, in stage Ⅰ, there is no muscle contraction at all; in stage Ⅱ, there is subtle flexion of the hand; in stage Ⅲ, the hand can flex more actively but cannot be opened; in stage Ⅳ, patients can volitionally extend the thumb and other fingers partially; in the stage Ⅴ, patients can hold a ball or a cylinder, and they can extend their fingers simultaneously; and in the stage Ⅵ, the paretic hand can almost accomplish every kind of functional grasping and extending, but the speed and coordination are a little bit worse than the contralateral limb. The recovery rules can also be applied to shoulders, elbows, forearms, and wrists. It is obvious that an improvement from no active movement to active movement is a hard step, and the separation movement, from finger grasping to finger opening, is also a difficult process. Therefore, facing a series of difficulties, the task specificity of hand motions is of great importance, and the correlation with feedback is the main link and is discussed in detail in the review.
Clinical efficacy in hand function rehabilitation of stroke patients has been revealed by several reviews. Remsik et al. [21] considered BCIs as a method of hand function rehabilitation after stroke with a review. Monge–Pereira et al. [22] suggested EEG-based BCIs interventions may be a promising rehabilitation approach in subjects with stroke by a systematic review. Carvalho et al. [23] suggested that neurofeedback training with EEG-based BCIs might promote both clinical and neurophysiologic changes in stroke patients. Bai et al. [24] investigated the effectiveness of BCIs in restoring upper extremity function after stroke. Even though they have testified to the effectiveness of the BCIs, hardly have they forced deeply on these questions: Why was a motor task chosen in each BCI trial, and is it suitable for a stroke patient? Some stroke patients received BCIs training but gained small improvements. Except for other reasons such as a lack of treatment times, short treatment duration, etc., can the motor tasks help promote better motor recovery? Thus, this review concentrates on the motor tasks and feedback of BCI clinical trials based on upper limb and hand interventions with BCIs systems in patients after stroke, which is truly suitable for them to solve their problems. We use the traditional method to search articles and draw clinical recommendations. This review aims: (1) to explore the motor tasks design in EEG-based BCIs clinical trials, (2) to analyze the association between motor tasks and the neurologic mechanism, and (3) to discuss the feedback combing the motor tasks that were suitable for stroke patients. […]
[Abstract] Clinical Evaluation of a New Mechatronic Glove for Hand Rehabilitation
Posted by Kostas Pantremenos in Paretic Hand on January 17, 2023
Abstract:
This paper presents the validation and the improvements of a glove-type mechatronic recovery system through clinical testing for patients with neurological disorders. This system, called MANUTEX, evaluates the flexion and extension of the hand, stimulates the increase of muscle strength, as well as the coordination of fine movements of the patient’s hand. Fine motor skills such as precision, dexterity and coordination of the hand are improved too. The use of an exoskeleton type system for passive mobilization and functional electrical stimulation (FES), with surface electrodes, improves the remaining voluntary movement the patients may perform. A comprehensive testing of the MANUTEX functionality was carried out in a technical laboratory of the technical university, as a preamble for a first set of clinical trials at the Neurology Department of the Clinical Rehabilitation Hospital of Iasi. The purpose of this paper is to present the overall validation process and clinical results of using a new mechatronic glove with in the rehabilitation process for post stroke neurological patients.
Published in: 2022 E-Health and Bioengineering Conference (EHB)
[Abstract] Soft Exoskeleton for Hand Rehabilitation: An Overview
Posted by Kostas Pantremenos in Paretic Hand, Rehabilitation robotics on January 17, 2023
Abstract:
Robotics based rehabilitation have attracted much attention during the past two decades. Recent intensive research publications in the area indicates that soft exoskeleton is one of the promising technologies for robotic-based rehabilitation. This paper aims to review the up-to-date research work in soft exoskeletons material, manufacturing, sensing and control for hand rehabilitation. Applied materials, its preferred properties, and manufacturing technologies of soft exoskeletons are reviewed. Different position and force sensing technologies as well as recent control techniques applying bio-signals as control signals are reviewed. The major challenges, which are also recommendations for future research work, are highlighted.
[Book Chapter] Hand Gesture Controlled Game for Hand Rehabilitation – Google Books
Posted by Kostas Pantremenos in Books, Paretic Hand, REHABILITATION, Video Games/Exergames on December 28, 2022

Hand Gesture Controlled Game for Hand Rehabilitation
Table of Content
Contents
PeerReview Statements | 1 |
Designing User Interface for an ElderlyMuslimFriendly Mobile Application | 3 |
Comparison of Machine Learning Models for IoT Malware Classification | 15 |
SafeTravelApplication with Blockchain Technology | 29 |
Location Profiling for RetailSite Recommendation Using Machine Learning Approach | 48 |
A Primary Study on the Performances and Techniques | 68 |
A Case Study Using Machine Learning Techniques for Prediction of House Prices in WP Malaysia | 79 |
Identifying Demographic Factors Attributed to the Infection Rate of Covid19 in Malaysia | 92 |
Colourassisted PCB Inspection System with Hardware Support for Realtime Environment | 313 |
A Residual CNN Model for ICD Assignment | 331 |
Virtual Reality Game for Physical Rehabilitation | 342 |
Preliminary Study on Shadow Detection in DroneAcquired Images with UNET | 357 |
Mediating Schema Ontology for Linked Open Data Cloud Integration Using BottomUp Schema Mapping | 369 |
QR Steganography for Information Hiding of Patient Record | 392 |
A TwoStage Classification Chatbot for Suicidal Ideation Detection | 405 |
Heart Disease Classification | 413 |
Machine Learning Approaches to Intrusion Detection System Using BOTPE | 104 |
Email Plugin Suite for kResilient IdentityBased Encryption and Searchable Encryption | 120 |
Classification of Emotion Stimulation via Iranian Music Using Sparse Representation of EEG Signal | 133 |
ICABased Sensorimotor Rhythms Detection in ALS Patients for BCI Applications | 145 |
A Hybrid Unsupervised Mining Approach for Customer Segmentation in B2C eCommerce | 156 |
A Digital Rights Management System for Media Content Consumption | 171 |
A Review | 182 |
CNNBased Traffic Sign Recognition | 195 |
Hand Gesture Controlled Game for Hand Rehabilitation | 205 |
A Review on Industrial Revolution 40 IR40 Readiness Among Industry Players | 216 |
Technology Usage for Sustainable Health and Wellbeing in Ecommerce Throughout the COVID 19 Pandemic | 232 |
Objectivity and Subjectivity Classification with BERT for Bahasa Melayu | 246 |
Predictive Modelling of Student Performance in MMU Based on Machine Learning Approach | 258 |
Face Mask Detection Using Deep Learning | 279 |
Traffic Light Recognition Assistance for Colour Vision Deficiency Using Deep Learning | 289 |
Research Framework and Design of Incorporation of Conversational Agent in Mathematics Learning | 301 |
A Review and Analysis of Tools Used from 2018 till 2022 in Requirements Engineering | 424 |
Automatic Face Mask Detection and Violation of Social Distancing Application | 439 |
A Hybrid Automated Essay Scoring Using NLP and Random Forest Regression | 448 |
Machine Learning Regression Models to Predict Particulate Matter PM25 | 458 |
A Review | 469 |
Performance Analysis of OAMBased Advanced Symbol Modulation Schemes for OFDM Over FSO System | 480 |
Comparison of Word Embeddings for Sentiment Classification with Preconceived Subjectivity | 488 |
Autoencoders with Reconstruction Error and Dimensionality Reduction for Credit Card Fraud Detection | 503 |
Lossless ECG Signal Compression Using Nonlinear Predictor and ASCII Character Encoding | 513 |
Comparison of Plain and Dense Skip Connections on UNet Architecture for Change Detection | 524 |
Underwater Image Semantic Segmentation with Weighted Average Ensemble | 533 |
Dynamic Hand Gesture Recognition Based on Deep Learning for Muslim Elderly Care | 544 |
Comparison of Deep Learning Methods for Underwater Image Enhancement | 558 |
Integration of Pedagogical Agent in Learning | 572 |
[ARTICLE] Mechanism Design and Performance Analysis of a Wearable Hand Rehabilitation Robot – Full Text
Posted by Kostas Pantremenos in Paretic Hand, REHABILITATION, Rehabilitation robotics on December 20, 2022
Abstract
Hand rehabilitation is critical to the recovery of post-stroke patients. However, designing a modular mechanism of the hand to improve the human-machine compatibility and precision of operation is still a challenge. This paper proposes a new type of hand exoskeleton rehabilitation robot with nine degrees of freedom. With the flexible rods, the passive range of motion for finger adduction/abduction is extended under the premise of independent flexion/extension of the metacarpophalangeal and proximal interphalangeal joint. Based on hand anatomy, the relationship between the offset of the metacarpophalangeal joint and the body height in the process of flexion and extension is discussed, and it is applied to the structure optimization and control system. The genetic algorithm is employed to achieve the size optimization, and the kinematics is analyzed. Finally, a prototype is built and preliminary experiments are carried out, including the range of motion and the grasping ability of the robot. The experimental results show that the robot can realize the patients’ hand rehabilitation function and has certain adaptability.
1. Introduction
Stroke is still the main cause of death. The global lifetime risk of stroke from 25 years onward has increased from 22.8% in 1990 to 24.9% in 2016 [1]. According to the World Health Organization (WHO), more than 15 million people worldwide suffer from stroke every year, and approximately 60% of stroke patients have lifelong hand dysfunction [2]. However, hands play almost the most important role in the activities of daily living (ADLs), which covers 54% of body motion function, so paying more attention to hand rehabilitation is an urgent demand [3]. With the development of the interdisciplinary medical-industrial, rehabilitation with the robot is gaining acceptance due to its unique advantages, such as assisting physicians with repetitive and tedious training exercises, providing vivid and interesting virtual scenes, etc. However, researchers currently focus more on the rehabilitation of large joints (shoulder, elbow, wrist, hip, knee, and ankle) and less on hand rehabilitation, which to a certain extent reduces the cure rate of hands [4]. Therefore, in-depth research and development of hand rehabilitation robots should be to carried out.
Researchers have achieved excellent results on hand rehabilitation robots, and each of them has its characteristics. Inspired by the detailed reviews [5], this paper analyzes typical hand rehabilitation robots based on their rigidity, and they can be divided into three types: rigid, hybrid, and soft. The rigid type devices usually consist of rigid materials and structures, such as linkages, gears, slide rails, etc. Amadeo [6] is an end-effector commercially available device with rehabilitation modalities, including passive, assisted, and active motions. The rigid exoskeleton developed by Orlando et al. [7] is used for the index and thumb, it adopts four-bar mechanisms to transmit power, and the motors are arranged on the side of the finger. The robot presented by Kawasaki et al. [8] consists of gears and linkages, and it has 18 degrees of freedom (DoF). It can drive all the joints of the hand but at the cost of a large number of motors and a complex structure. For easy manufacturing and maintenance, the rigid electromechanical exoskeleton designed by Singh et al. [9] simultaneously moves the wrist joint and metacarpophalangeal joint with only a single motor, this unified handling of multiple joints simplifies the mechanical structure and reduces controlling difficulty. Oscar et al. [10] developed a robotic exoskeleton to present a solution for hand rehabilitation. It achieves flexion/extension(F/E) motion by rigid worm and gear, and a virtual environment is developed to attain human–machine interaction during the training process. Furthermore, driving one finger by a single motor through series connection between multiple linkage mechanisms seems popular in situations where resources are limited or cost savings are needed [11,12,13].
Due to the characteristics of flexible components (spring, Bowden wire, etc.), they could drive the device remotely and protect patients by its deformation or back-driven ability, as well as detect the patients’ motion angle or joint force. Therefore, it is increasingly adopted in hand rehabilitation robots. The devices that combine rigid and soft components are called hybrid in this paper. Starting with the index finger, Jo et al. [14] developed a hand exoskeleton combining the energy storage properties of spring and the precision of motion of a linkage, which has an automatic correction function for the patients’ motion. Cheng et al. [15,16] achieved active F/E and adduction/abduction(A/A) motion on an index finger rehabilitation robot through wire and linkage, allowing for more rehabilitation motion of the device. The hybrid hand rehabilitation robot proposed by Li et al. [17] includes an elastic-rod transmission system; it takes advantage of the Nickel-Titanium (Ni-Ti) rods with the super-elastic feature and prevents patients from potential injury by allowing a specific position error. The device developed by Agarwal et al. [18] adopts Bowden wire for remote actuation, easing the burden of patient donning, other robots that use the same cable approach are [19,20,21,22]. Additionally, series elastic actuators are often used in hand exoskeletons in hybrid type, such as [23,24].
Soft type means that the body of the device is almost always composed of soft compliant materials, and they are mostly presented in the form of gloves. The robotic rehabilitation glove developed by TRAN et al. [25] assembled nitinol wires and soft materials, such as silicone and Velcro to assist the hand in gripping, which is lightweight, compact, and highly adaptable. The soft glove designed by Fei et al. [26] could achieve separate as well as coordinate motion exercises of fingers and the wrist; the core component of this device is the Double-DOF soft Pneumatic Bending Actuators (DPBAs); this glove has potential in extending the hand functional training. Bützer et al. [27] developed a wearable exoskeleton named RELab tenoexo, it mainly consists of springs and has the advantage of low weight, uninstructive size, and high wearing comfort, but its excellent assist function affects the performance in rehabilitation, such as lacking the ability to train single joints. The fabric-based soft robotic glove designed by Cappello et al. [28] consists of different fabric layers of specific material properties and enclosed air bladders, this device is capable of grasping objects with different shapes due to its intrinsically compliant structure, and it is versatile enough to be used both in clinical as well as home settings. The soft exoskeleton presented by Yap et al. [29] uses pneumatic actuators with variable stiffness; this device is able to perform hand therapy exercises, such as full fist, straight fist, hook fist and table top, at different locations and it could assist patients with acceptable range of motion and force.
It can be seen that hand rehabilitation robots can be presented in various ways from the investigation above. Generally speaking, rigid type usually has high control accuracy and can drive multiple joints; it behaves well in physical therapy (PT) but also has the disadvantages of heavy load, difficult to wear, and requirements for joint alignment between human and machine. Soft type has the advantages of fewer drive components, being easy to wear, and having a lighter weight. It could assist patients in ADLs and perform well in occupational therapy (OT). This type of robot is mostly driven by the pneumatic or hydraulic actuators, but it is an unavoidable problem to arrange these actuators due to their large size. From the points above, the hybrid type seems to be a good form of hand rehabilitation robot because it combines the advantages of both rigid and soft, i.e., it could achieve precise control in training, and it reduces the burden on the patients, improving the adaptability and safety. In fact, the development of hybrid type has been increasing rapidly in recent years.
This paper proposes a hybrid type hand rehabilitation robot. In Table 1, some state-of-art robots were compared on aspects including type, active DoF, power transmission method, type of actuator, and decoupling of MCP/PIP joints. According to the factors above, the robot proposed by this paper has more DoF than others, improving the precision of operation. Specifically, first, the main structure of the robot is rigid, and nitinol rods are adopted as part of the drive component to expand the adaptability to A/A motion of the fingers; second, finger bones are designed as part of the mechanism, it realizes the joint alignment of the human and machine; third, Bowden wire is used for power transmission, it allows the control box to be positioned away from patients, reducing the burden on the hand. The most important feature of this device is adaptability and safety and its ability to realize PT and OT training. […]
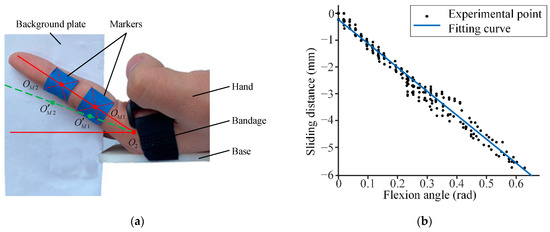