If you’ve followed the latest from the world of neuroscience, you might get the impression that the field is in deep trouble.
In July, a report in the Proceedings of the National Academy of Sciences declared that as many as 40,000 papers using the standard tool in neuroscience research, functional magnetic resonance imaging (fMRI), could suffer from a statistical flaw that rendered their results to be a false positive.
Headlines feared the worst:
- “A bug in fMRI software could invalidate 15 years of brain research”
- “Why Two Decades of Brain Research Could Be Seriously Flawed”
- “Software faults raise questions about the validity of brain studies”
These studies aren’t necessarily wrong, per se. It’s that the common statistical software they used is prone to wrongly guess the borders of “lit up” areas in the brain.
But authors of the PNAS paper quickly realized their estimate of 40,000 papers was too high. (The actual number of papers implicated is probably closer to 3,500, one of the authors wrote in a blog post. And PNAS has since amended the paper.)
Still, the results cast some doubt over the validity of fMRI. And they weren’t an anomaly. Over the past few years, doubt about fMRI research has been mounting. Researchers have found brain activity in dead fish. One 2009 paper found an epidemic of “puzzlingly high” correlations in fMRI research.
All this uncertainty has provoked a question: Is fMRI actually a faulty tool that should not be trusted?
Not at all, neuroscientists say. “It’s unfair to blame the tool,” Rebecca Saxe of MIT, who has been using fMRI since its earliest days, told me. “It’s like blaming a telescope when somebody’s wrong about [identifying] a planet. It’s not the telescope’s fault. The general problem is there are lots of ways to fool yourself with fMRI data.”
There are questions fMRI is good at answering and questions it is bad at answering. There are right ways to use fMRI, and there are irresponsible ways to use it.
I asked several experts in the field a simple question: What’s most important to know about fMRI and its limitations? Here’s what I learned.
1) An fMRI measures blood flow, not neural activity
When fMRI first became available as a scientific tool in the 1990s, it was a revelation.
Before then, scientists who wanted to learn about brain function had few options: They could wait for a patient with a brain injury to come along and test her mental abilities. Or they could inject people with radioactive dyes and then scan them with X-rays. The arrival of fMRI meant that a great many more scientists could study the brain. Neuroscience, as a field of research, exploded. In the past 20 years, 40,000 papers have been publishedusing fMRI.
“With fMRI, suddenly you could study the brain of a healthy person,” says Saxe. Better yet, fMRI wasn’t dangerous. You could repeat tests on the same person without fear of harming them. “There was a lot of hope,” she says. Finally, scientists thought they could peer into the brain and find the cause of autism.
And it was all thanks to magnets. An fMRI is conducted in a MRI machine equipped to scan people’s heads — it’s a giant doughnut-shaped device (people are loaded in through the center on a sliding table).The machine’s huge magnets can pick up on small changes in the brain; specifically, they’re looking for the presence of oxygenated blood.
When the brain region is activated, it calls out for more oxygen. The fMRI then follows oxygenated blood as it flows through the brain. On the printout of the scan, these oxygenated brain regions “light up.” Scientists can see areas smaller than a millimeter cubed (also called a voxel, which just means it’s a pixel in three dimensions) That blood flow is a sign that neural activity is happening in a given brain region.
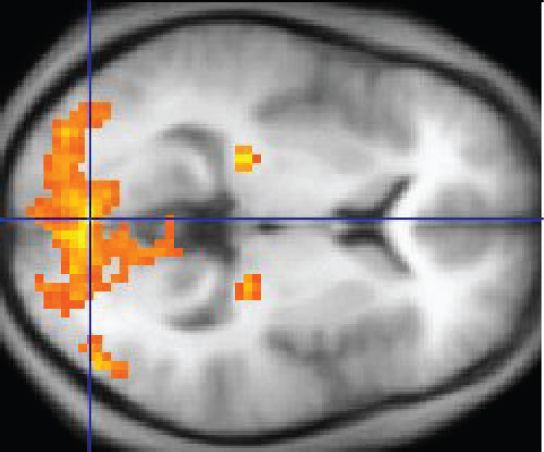
From the 1990s onward, the use of the tool exploded. In 2015, there were more than 29,000 academic articles in the PubMed database mentioning “fMRI.”
2) fMRI studies go wrong not just because of the limitations of the tool. They go wrong because science, overall, has flaws.
With fMRI, scientists began to chart an atlas of the brain and its function. They confirmed that there was a specific area of the brain devoted to interpreting faces. And they found wonderfully simple things, like how wiggling of the fingers are controlled by a tiny area near the earlobes. (Alas, fMRI hasn’t revealed the secrets to disorders like autism or schizophrenia.)
But amid the successes, there was a quietly growing concern that labs were using the tool inappropriately and generating false positives with it.
The July PNAS paper was the latest in a string of papers casting doubt fMRI science. Here are two most famous ones:
- In 2009, a researcher showed brain activity in a dead salmon. Dead salmon aren’t thinking, and the paper revealed that neuroscientists need to be vigilant about separating out signal from noise. (The neuroscientists I spoke with said the field was largely aware of this problem and could correct for it when this paper came out.)
- Also in 2009, a paper in Perspectives in Psychological Science found an epidemic of “puzzlingly high” correlations in papers that tried to associate brain scans with personality types.
“The common thing people would do is say, ‘We found that activation in some area correlates with some aspect of people’s personality,’” Russell Poldrack, a researcher at Stanford’s Center for Reproducible Neuroscience, explains. They’d then focus their analysis on that specific area and find extremely high correlations. “This is problematic because it is basically double dipping.”
The Perspectives paper concluded: “[I]t is quite possible that a considerable number of relationships reported in this literature are entirely illusory.”
Each of these papers highlighted a big concern with fMRI methods, and for the most part, the field has corrected course. But problems sill remain.
There’s currently a bigger “crisis” in psychology and social science where researchers are realizing some of their most celebrated findings don’t replicate under stricter methods. “All the things that are wrong in psychology are clearly wrong in neuroimaging,” Poldrack says.
At Vox, we’ve discussed these problems in science at length. There’s publication bias — the trend that journals only publish positive, confirmatory results. That creates the file drawer effect: Because negative results are not published, the published literature may paint a too-rosy description of a theory. And then there’s p-hacking: the suite of methodological tweaks researchers can employ to ensure they land on a significant (i.e., publishable) result.
The problems make for an uneasy time for scientists: They’d like to charge ahead with new scientific questions, but also feel a nagging anxiety that they should recheck all the work their questions have been built upon.
There’s some reason to suspect these problems might be even be more problematic in neuroscience than in psychology at large. Here’s why: fMRIs are extremely expensive, costing hundreds of dollars an hour to operate. Money is pressure, and scientists don’t want to be left for nothing to show for a $20,000 study. The expense also makes it less likely for scientists to do replications of past work, and less likely to run large numbers of subjects through an experiment.
3) fMRI is good at mapping broad regions of brain activity. But it’s not good at specifics.
We know fMRI measures blood flow and not neural activity directly. And the assumption (that’s been validated in studies) is that blood flow correlates with neural activity. The blood flow can reveal changes in brain areas as small as a millimeter cubed.
But still, at best, that’s just a crude view of things. There can be hundreds of thousands of neurons in a tiny voxel.

“The analogy is it’s like flying over a city and seeing where the lights are on,” Tal Yarkoni, who studies neuroscience research methods at University of Texas Austin, says. If you’re in an airplane, you might look the window and identify a patch of land as a residential area. But it’s impossible to know what people are doing in their homes. And it’s difficult to understand how people in their homes interact with the city’s center.
And fMRI is like that. You can learn what broad areas of the brain are working. But figuring out what, exactly, those brain areas are doing is a totally different problem.
4) The results of a study can be correct, but the interpretation of those results can be very, very wrong
The frustrating part about fMRI research is that it can be really hard to interpret what a brain region “lighting up” means.
“For a while anytime people saw activation in the anterior cingulate cortex, they would say, that must mean the subject is experiencing conflict,” Poldrack says. “What we found is that the anterior cingulate lights up in almost a third of all papers. The fact that it is active tells you almost nothing about what is going on.”
It’s too easy to look at what “lights up” in the brain, and then craft a story around it. Here’s an example from 2007, after a group of scientists put 20 voters in an fMRI and asked them questions about politicians:
When viewing images of [Hillary Clinton], these voters exhibited significant activity in the anterior cingulate cortex, an emotional center of the brain that is aroused when a person feels compelled to act in two different ways but must choose one. It looked as if they were battling unacknowledged impulses to like Mrs. Clinton.
Did the voters’ anterior cingulates “light up”?
Yes.
Does it mean they’re conflicted about her as a candidate?
You can’t really say.
“It’s crazy to think of a situation where there’s not an association between some brain region and some behavior,” Yarkoni says. “Pick any brain region you like, and pick behavior you’d like — and the correlation between the two is not going to be zero. It can’t be zero. There are hundreds of millions of neurons in that brain region. And somehow, some way, there’s going to be a path between activation in that region to the behavior you care about.”
To try to infer “what the subject is thinking or what their preferences are by the activity of one part of the brain is extremely difficult, ” says Peter Bandettini, chief of the department on fMRI methods at the National Institute of Mental Health.
Here’s another, more recent example.
You’ve might have seen a headline like “Dogs understand both words and intonation of human speech.” The story was based off a Science paper that found words of praise raised activation in the left hemisphere of dogs’ brains while being scanned in an fMRI. That result was interpreted in many articles to mean the dogs understood the words, because in humans, we respond to words we understand with a similar pattern of activation.
But if it’s hard to draw conclusions from human fMRI data, dog data may be even a bigger stretch.
“What they’ve shown is that the left hemisphere is more active than the right for auditory processing,” Gregory Berns, a neurobiologist not involved in the study, writes me in an email. “But this is a far reach from lexical processing, which is what they claim.”
(Attila Andics, one of the authors of the dog fMRI paper wrote me, disagreeing with this assessment. “The left hemisphere was more active for lexically marked sounds — meaningful words — but not for … meaningless sound sequences,” he writes. “This is why it was fair to call it lexical processing.” But Andics agrees that a lot of the articles about his study were exaggerated: “We made no claims about what dogs actually understand,” he says.)
If a headline based on an fMRI study feels a bit too incredible, it’s possible that the journalist (perhaps egged on by the university’s press department and the scientists themselves) is reading too much into the results.
5) fMRI isn’t great at establishing the order of brain activity
The brain is complexly interwoven. All of its structures work with one another to complete thoughts. In order to understand how it works, we need to understand how activation in one region impacts activation in another.
Surprisingly, fMRI isn’t very good at creating a fine-grained time series of brain activity.
Brain regions “turn on” within milliseconds of one another. But “the hemodynamic response, just due to the blood vessels, is about two seconds. so that certainty is down to about two seconds on how much you can discern when something turns on thoughts,” Bandettini says. “You just can’t discern one part of the brain turning on first before the other.”
6) Ultimately, progress will be made: fMRI researchers keep using the machine in new, intriguing ways
The classic fMRI studies are kind of simple. Place a participant in the machine, have him complete as task, and then see which regions of the brain are active during that task. The goal is to create a map of the functioning brain — what regions matter for which tasks and why.
For the reasons mentioned above, this approach has many limitations. To overcome them, fMRI researchers have come up with a new, radical-sounding approach for conducting studies: They’ve stopped caring what the brain is actually doing.
fMRI produces patterns of activity. These patterns are more complicated in ways that we may never be able to understand. But a machine might.
Recently, studies have been employing the following design: Scientists put people in an MRI, have them do a task, and then, using machine-learning software, ask the computers to look for patterns between the brain activation and the task the participant is completing.

The scientists, in effect, train the computer to brain-read. That is: They can take guesses about what a participant is doing just by looking at brain data. “You might not care about the brain at all; you might just be viewing the brain as a tool for trying to predict some outcome of interest,” Yarkoni says.
In a way, the prediction makes fMRI a cleaner science: Either a prediction is true or it is not. There’s less ambiguity in interpreting results.
This has some practical applications. A computer can look to see what different levels of pain look like in an fMRI scan. Then when a new person enters the scanner, the computer can better predict what level of pain she’s feeling.
“The next phase of fMRI is … huge databases of thousands or tens of thousands of subjects, and having very well-curated data along with a whole list of behavioral measures for each subject,” Bandettini says. “You can then go back and start using this more clinically.” You can put a patient in an fMRI and ask the computer to see if his brain is exhibiting the complicated pattern that suggests it’s schizophrenic.
This approach can also help us learn about brain structures as well.
Recently I reported on a study where scientists used fMRI and machine learning to reconstruct images the participants saw in the scanner. The regions targeted in the scan have been long known to be related to vivid memories. Because the artificial intelligence was able to make the connection between faces and brain activity, that suggests that region of the brain has something to do with remembering faces. Which shows how this machine learning approach can help confirm the insights gained in more traditional fMRI studies.
7) And remember: Researchers have more than one tool to study the brain
Also know that fMRI isn’t the only tool researchers have to peer into the brain. There are electroencephalograms (a.k.a. EEG — think of those caps with dozens of electrodes), which are good at seeing broad patterns of brain activity in a time series (like states of sleep). There’s magnetoencephalography, MEG, which uses magnets to record electrical currents in the brain. And there are new exciting tools like optogenetics, a technique for activating and studying neural pathways with light (this has only been used in lab animals). Like fMRI, each tool has its advantages and flaws for scientists to grapple with.
There will never be one ultimate tool to understand the brain — besides, perhaps, for the brain itself.
The scientists I spoke to all agreed: Researchers have published sloppy work based on fMRI data in the past. Sadly, that’s true of any tool in science. But here’s the good news: Researchers want to get better.
Correction: This post originally misstated how MRI machines operate. They don’t “spin magnets.” The magnets remain stationary and cause atoms inside the scanner to spin aligned in the same direction.