Posts Tagged brain–computer interface
[Review] Brain–Computer Interfaces for Upper Limb Motor Recovery after Stroke: Current Status and Development Prospects – Full Text
Posted by Kostas Pantremenos in Paretic Hand on January 17, 2024
Brain–computer interfaces (BCIs) are a group of technologies that allow mental training with feedback for post-stroke motor recovery. Varieties of these technologies have been studied in numerous clinical trials for more than 10 years, and their construct and software are constantly being improved. Despite the positive treatment results and the availability of registered medical devices, there are currently a number of problems for the wide clinical application of BCI technologies. This review provides information on the most studied types of BCIs and its training protocols and describes the evidence base for the effectiveness of BCIs for upper limb motor recovery after stroke. The main problems of scaling this technology and ways to solve them are also described.
Introduction
Brain–computer interface (BCI) is a technology that allows to convert data on the electrical or metabolic activity of the brain into control signals for an external technical device. In post-stroke rehabilitation, BCI is used to provide feedback to a patient during motor imagery training [1–3]. The scientific justification for this method has been the data on the positive effect of the motor imagery process on neuroplasticity due to activation of motor structures of the central nervous system (CNS) [4–8]. By providing feedback during motor imagery, the BCI systems enhance the effectiveness of such training sessions [9]. In general, training with the use of the BCI technology in patients after stroke includes the following processes: a patient is asked to mentally perform a movement of the paralyzed limb; the BCI technology using non-invasive sensors records brain signals accompanying the mental performance of the task; in real time, these signals are recognized and converted into a control command for an external device; the patient is provided with feedback on the quality of the mental task performance using the external device [10].
To date, at least 20 randomized controlled trials (RCTs) on the use of BCI for upper limb motor recovery after stroke are known worldwide, and 11 systematic reviews, 8 of which are accompanied by a meta-analysis, have been published on this topic between 2019 and 2023 [11–21]. Foreign and domestic manufacturers have developed several medical devices for use in clinical practice of post-stroke rehabilitation [22–25].
In Russia, clinical trials of BCI after stroke first began in 2011 at Research Center of Neurology (Moscow, Russia) [26, 27]. In a subsequent multicentre RCT, it was shown that a course of training with the BCI–exoskeleton complex improved the rehabilitation results of patients with focal brain damage in terms of hand motor recovery [28]. The proven technology was subsequently registered as a medical device and is currently used in a number of clinical centres [24, 29].
Despite the extensive evidence base and the availability of ready-made BCI technologies, there are currently some limitations to their widespread use in post-stroke rehabilitation, and further research and development is underway [30–37].
The aim of this review is to analyse scientific articles devoted to the study of the use of BCI technologies in post-stroke upper limb paresis, to outline the main problems and prospects for further development in this field.
Literature search methodology
Articles from peer-reviewed, full-text, open access scientific journals on the use of non-invasive BCIs for upper limb motor recovery after stroke were selected for analysis. The search query was formulated according to the rules of the MEDLINE bibliographic database: ((brain–computer[tiab] OR brain–machine[tiab] OR neural interfac*[tiab]) OR “Brain–Computer interfaces”[Mesh]) AND stroke[mh] AND (upper extremity[tiab] OR hand[tiab] OR arm[tiab]). Additionally, a literature search was conducted in the eLIBRARY.RU system using the key words “brain–computer interface”, “neurocomputer interface”, “neurointerface”. The date of the search was July 3, 2023.
Varieties of brain–computer interface systems and their application after stroke
All BCIs used in research or in the practice of post-stroke rehabilitation have distinctive features (see the Figure). The training protocols and BCI models studied in RCTs differ in the control paradigm of the interface, the type of signal recorded, the online signal processing algorithm, and the type of external technical device for providing feedback.
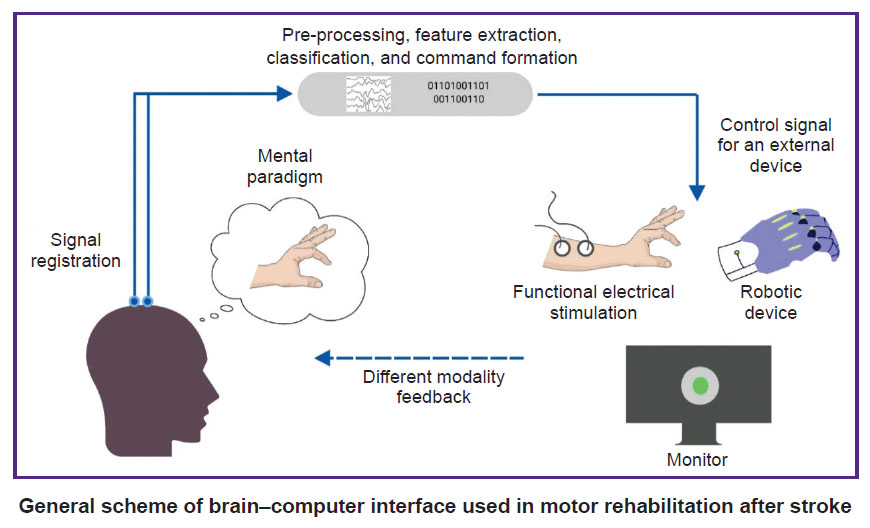
[ARTICLE] Motor Network Reorganization Induced in Chronic Stroke Patients with the Use of a Contralesionally-Controlled Brain Computer Interface – Full Text
Posted by Kostas Pantremenos in REHABILITATION on July 6, 2022
ABSTRACT
Upper extremity weakness in chronic stroke remains a problem not fully addressed by current therapies. Brain–computer interfaces (BCIs) engaging the unaffected hemisphere are a promising therapy that are entering clinical application, but the mechanism underlying recovery is not well understood. We used resting state functional MRI to assess the impact a contralesionally driven EEG BCI therapy had on motor system functional organization. Patients used a therapeutic BCI for 12 weeks at home. We acquired resting-state fMRI scans and motor function data before and after the therapy period. Changes in functional connectivity (FC) strength between motor network regions of interest (ROIs) and the topographic extent of FC to specific ROIs were analyzed. Most patients achieved clinically significant improvement. Motor FC strength and topographic extent decreased following BCI therapy. Motor recovery correlated with reductions in motor FC strength across the entire motor network. These findings suggest BCI-mediated interventions may reverse pathologic strengthening of dysfunctional network interactions.
1. Introduction
Stroke causes adult disability in approximately 800,000 adults annually in the United States [1]. Unilateral upper motor weakness, known as hemiparesis, occurs in 77% of new stroke cases [2]. Hemiparesis frequently persists into the chronic stage of stroke; 65% of chronic stroke patients report reduced motor function 6 months after stroke [3,4]. Patients rarely obtain substantial motor improvement 3 months after a stroke, with residual motor deficits effectively becoming permanent [5–11]. Behavioral adaptations instead of spontaneous recovery generally underlie subsequent improvements [9]. Recent innovations in rehabilitation techniques, however, offer new opportunities for motor recovery, even in the chronic stage.
The efficacy of brain–computer interfaces (BCIs) for post-stroke motor rehabilitation has been demonstrated with a variety of designs [12]. However, there is a lack of consensus regarding the neurophysiological mechanisms driving recovery through BCI [13–16], which necessitated further study. Functional recovery was previously shown in a severely impaired chronic stroke population treated with a BCI system using signals from the contralesional motor cortex [17]. The former study used cortical EEG signals to control a robotic hand orthosis. Additionally, the efficacy of BCI on motor recovery was linked to changes in EEG activity in motor regions within frequencies used for BCI [17]. Given that this contralesional BCI system, known as the IpsiHand (Neurolutions, Santa Cruz CA), recently received FDA market authorization and will be applied to stroke populations, understanding the mechanism of its clinical benefit is of high importance. Power fluctuations in alpha (8–12 Hz) and beta (13–25 Hz) frequencies are observed in motor cortex during motor activity [18,19]. These frequencies are also used for BCI control [17]. We therefore hypothesized BCI may have affected neural circuitry to facilitate motor recovery via experience-dependent plasticity. However, previously recorded EEG signals only assess broad cortical regions with limited anatomic specificity. Here, we used functional MR imaging to study whether BCI therapy affected functional connectivity organization in the motor cortex and cerebellum.
Networks of correlated spontaneous brain activity during rest have been extensively described using functional MRI (fMRI) [20–22]. Strokes disrupt ‘functional connectivity’ networks [23–26]. Furthermore, the extent of network disruption correlated with stroke-induced impairments in multiple behavioral domains [23,25–27]. Strokes altered network modularity, typically by a decrease and then a partial recovery in association with behavioral improvements [25,28,29]. Connectivity changes between specific regions have also been implicated in stroke recovery [30–32]. Further, performance on motor function assessment tasks after a stroke was reduced with disrupted interhemispheric motor network connectivity [24,33]. Thus, recovery from stroke induced by BCI might involve changes in resting-state functional connectivity (rsFC).
The objective of the current study was to determine whether an EEG-driven BCI controlled by motor signals from the unaffected hemisphere reorganized brain networks for motor control. Based on previous reports linking motor network organization with post-stroke motor function, we hypothesized that motor recovery achieved during BCI therapy would change motor network connectivity, and that these rsFC changes in motor systems would correlate with the strength of recovery. Increases in interhemispheric connectivity, and decreases in intrahemispheric connectivity have previously been reported during stroke recovery [24,25,30,33–35]. Consequently, we hypothesized motor recovery via BCI would lead to similar patterns of change in inter- and intrahemispheric rsFC. The unexpected findings in this study suggest a potential novel recovery mechanism associated with BCI induced recovery in chronic stroke.[…]
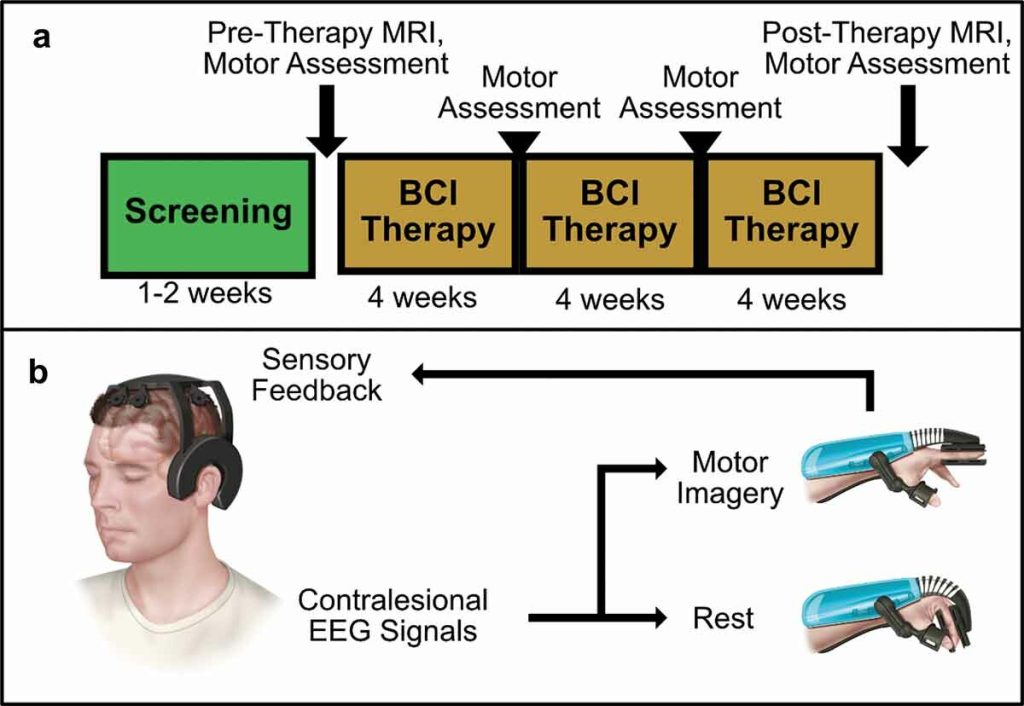
Figure 1. BCI Intervention protocol and system design overview. (a) Protocol Timeline. Screening for EEG feature frequency and inclusion and exclusion criteria occur over several sessions in a 1–2 week period. Following screening, patients undergo an MRI scan and motor assessments before receiving their BCI device. Patients perform BCI therapy for 12 weeks at home, returning every 4 weeks for motor assessments. A final MRI scan and motor assessment is performed after 12 weeks of therapy. (b) BCI System Design.
[Abstract] Brain–Computer Interface Training Based on Brain Activity Can Induce Motor Recovery in Patients With Stroke: A Meta-Analysis
Posted by Kostas Pantremenos in Paretic Hand on January 5, 2022
Abstract
Background
Brain–computer interface (BCI) is a procedure involving brain activity in which neural status is provided to the participants for self-regulation. The current review aims to evaluate the effect sizes of clinical studies investigating the use of BCI-based rehabilitation interventions in restoring upper extremity function and effective methods to detect brain activity for motor recovery.
Methods
A computerized search of MEDLINE, CENTRAL, Web of Science, and PEDro was performed to identify relevant articles. We selected clinical trials that used BCI-based training for post-stroke patients and provided motor assessment scores before and after the intervention. The pooled standardized mean differences of BCI-based training were calculated using the random-effects model.
Results
We initially identified 655 potentially relevant articles; finally, 16 articles fulfilled the inclusion criteria, involving 382 participants. A significant effect of neurofeedback intervention for the paretic upper limb was observed (standardized mean difference = .48, [.16-.80], P = .006). However, the effect estimates were moderately heterogeneous among the studies (I2 = 45%, P = .03). Subgroup analysis of the method of measurement of brain activity indicated the effectiveness of the algorithm focusing on sensorimotor rhythm.
Conclusion
This meta-analysis suggested that BCI-based training was superior to conventional interventions for motor recovery of the upper limbs in patients with stroke. However, the results are not conclusive because of a high risk of bias and a large degree of heterogeneity due to the differences in the BCI interventions and the participants; therefore, further studies involving larger cohorts are required to confirm these results.
[REVIEW] Brain–computer interface robotics for hand rehabilitation after stroke: a systematic review – Full Text
Posted by Kostas Pantremenos in Paretic Hand, Rehabilitation robotics on January 30, 2021
Abstract
Background
Hand rehabilitation is core to helping stroke survivors regain activities of daily living. Recent studies have suggested that the use of electroencephalography-based brain-computer interfaces (BCI) can promote this process. Here, we report the first systematic examination of the literature on the use of BCI-robot systems for the rehabilitation of fine motor skills associated with hand movement and profile these systems from a technical and clinical perspective.
Methods
A search for January 2010–October 2019 articles using Ovid MEDLINE, Embase, PEDro, PsycINFO, IEEE Xplore and Cochrane Library databases was performed. The selection criteria included BCI-hand robotic systems for rehabilitation at different stages of development involving tests on healthy participants or people who have had a stroke. Data fields include those related to study design, participant characteristics, technical specifications of the system, and clinical outcome measures.
Results
30 studies were identified as eligible for qualitative review and among these, 11 studies involved testing a BCI-hand robot on chronic and subacute stroke patients. Statistically significant improvements in motor assessment scores relative to controls were observed for three BCI-hand robot interventions. The degree of robot control for the majority of studies was limited to triggering the device to perform grasping or pinching movements using motor imagery. Most employed a combination of kinaesthetic and visual response via the robotic device and display screen, respectively, to match feedback to motor imagery.
Conclusion
19 out of 30 studies on BCI-robotic systems for hand rehabilitation report systems at prototype or pre-clinical stages of development. We identified large heterogeneity in reporting and emphasise the need to develop a standard protocol for assessing technical and clinical outcomes so that the necessary evidence base on efficiency and efficacy can be developed.
Background
There is growing interest in the use of robotics within the field of rehabilitation. This interest is driven by the increasing number of people requiring rehabilitation following problems such as stroke (with an ageing population), and the global phenomenon of insufficient numbers of therapists able to deliver rehabilitation exercises to patients [1, 2]. Robotic systems allow a therapist to prescribe exercises that can then be guided by the robot rather than the therapist. An important principle within the use of such systems is that the robots assist the patient to actively undertake a prescribed movement rather than the patient’s limb being moved passively. This means that it is necessary for the system to sense when the patient is trying to generate the required movement (given that, by definition, the patient normally struggles with the action). One potential solution to this issue is to use force sensors that can detect when the patient is starting to generate the movement (at which point the robot’s motors can provide assistive forces). It is also possible to use measures of muscle activation (EMGs) to detect the intent to move [3]. In the last two decades there has been a concerted effort by groups of clinicians, neuroscientists and engineers to integrate robotic systems with brain signals correlated with a patient trying to actively generate a movement, or imagine a motor action, to enhance the efficacy and effectiveness of stroke rehabilitation- these systems fall under the definition of Brain Computer Interfaces, or BCIs [4].
BCIs allow brain state-dependent control of robotic devices to aid stroke patients during upper limb therapy. While BCIs in their general form have been in development for almost 50 years [5] and were theoretically made possible since the discovery of the scalp-recorded human electroencephalogram (EEG) in the 1920s [6], their application to rehabilitation is more recent [7,8,9]. Graimann et al. [10] defined a BCI as an artificial system that provides direct communication between the brain and a device based on the user’s intent; bypassing the normal efferent pathways of the body’s peripheral nervous system. A BCI recognises user intent by measuring brain activity and translating it into executable commands usually performed by a computer, hence the term “brain–computer interface”.
Most robotic devices used in upper limb rehabilitation exist in the form of exoskeletons or end-effectors. Robotic exoskeletons (i.e., powered orthoses, braces) are wearable devices where the actuators are biomechanically aligned with the wearer’s joints and linkages; allowing the additional torque to provide assistance, augmentation and even resistance during training [11]. In comparison, end-effector systems generate movement through applying forces to the most distal segment of the extremity via handles and attachments [11]. Rehabilitation robots are classified as Class II-B medical devices (i.e., a therapeutic device that administers the exchange of energy, mechanically, to a patient) and safety considerations are important during development [12, 13]. Most commercial robots are focused on arms and legs, each offering a unique therapy methodology. There is also a category of device that target the hand and finger. While often less studied than the proximal areas of the upper limb, hand and finger rehabilitation are core component in regaining activities of daily living (ADL) [14]. Many ADLs require dexterous and fine motor movements (e.g. grasping and pinching) and there is evidence that even patients with minimal proximal shoulder and elbow control can regain some hand capacity long-term following stroke [15].
The strategy of BCI-robot systems (i.e. systems that integrate BCI and robots into one unified system) in rehabilitation is to recognise the patient’s intention to move or perform a task via a neural or physiological signal, and then use a robotic device to provide assistive forces in a manner that mimics the actions of a therapist during standard therapy sessions [16]. The resulting feedback is patient-driven and is designed to aid in closing the neural loop from intention to execution. This process is said to promote use-dependent neuroplasticity within intact brain regions and relies on the repeated experience of initiating and achieving a specified target [17, 18]; making the active participation of the patient in performing the therapy exercises an integral part of the motor re-learning process [19, 20].
The aforementioned scalp-recorded EEG signal is a commonly used instrument for data acquisition in BCI systems because it is non-invasive, easy to use and can detect relevant brain activity with high temporal resolution [21, 22]. In principle, the recognition of motor imagery (MI), the imagination of movement without execution, via EEG can allow the control of a device independent of muscle activity [10]. It has been shown that MI-based BCI can discriminate motor intent by detecting event-related spectral perturbations (ERSP) [23, 24] and/or event-related desynchronisation/synchronisation (ERD/ERS) patterns in the µ (9–11 Hz) and β (14–30 Hz) sensorimotor rhythm of EEG signals [24]. However, EEG also brings with it some challenges. These neural markers are often concealed by various artifacts and may be difficult to recognise through the raw EEG signal alone. Thus, signal processing (including feature extraction and classification) is a vital part of obtaining a good MI signal for robotic control. A general pipeline for EEG data processing involves several steps. First, the data undergo a series of pre-processing routines (e.g., filtering and artifact removal) before feature extraction and classification for use as a control signal for the robotic hand. There are variety of methods to remove artifact from EEG and these choices depend on the overall scope of the work [25]. For instance, Independent Component Analysis (ICA) and Canonical Correlation Analysis (CCA) can support real-time applications but are dependent on manual input. In contrast, regression and wavelet methods are automated but support offline applications. There also exist automated and real-time applications such as adaptive filtering or using blind source separation (BSS) based methods. Recently, the research community has been pushing real-time artifact rejection by reducing computational complexity e.g. Enhanced Automatic Wavelet-ICA (EAWICA) [26], hybrid ICA—Wavelet transform technique (ICA-W) [27] or by developing new approaches such as adaptive de-noising frameworks [28] and Artifact Subspace Reconstruction (ASR) [29]. Feature extraction involves recognising useful information (e.g., spectral power, time epochs, spatial filtering) for better discriminability among mental states. For example, the common spatial patterns (CSP) algorithm is a type of spatial filter that maximises the variance of band pass-filtered EEG from one class to discriminate it from another [30]. Finally, classification (which can range from linear and simple algorithms such as Linear Discriminant Analysis (LDA), Linear Support Vector Machine (L-SVM) up to more complex techniques in deep learning such as Convolutional Neural Networks (CNN) and Recurrent Neural Networks (RNN) [31, 32] involves the translation of these signals of intent to an action that provides the user feedback and closes the loop of the motor intent-to-action circuit.
The potential of MI-based BCIs has gained considerable attraction because the neural activity involved in the control of the robotic device may be a key component in the rehabilitation itself. For example, MI of movement is thought to activate some of the neural networks involved in movement execution (ME) [33,34,35,36]. The resulting rationale is that encouraging the use of MI could increase the capacity of the motor cortex to control major muscle movements and decrease the necessity to use neural circuits damaged post-stroke. The scientific justification for this approach was first provided by Jeannerod [36] who suggested that the neural substrates of MI are part of a shared network that is also activated during the simulation of action by the observation of action (AO) [36]. These ‘mirror neuron’ systems are thought to be an important component of motor control and learning [36]—hence the belief that activating these systems could aid rehabilitation. The use of a MI-BCI to control a robot in comparison to traditional MI and physical practice provides a number of benefits to its user and the practitioner. These advantages include the fact that the former can provide a more streamlined approach such as sensing physiological states, automating visual and/or kinaesthetic feedback and enriching the task and increasing user motivation through gamification. There are also general concerns around the utility of motor imagery without physical movement (and the corresponding muscle development that comes from these) and it is possible that these issues could be overcome through a control strategy that progressively reduces the amount of support provided by the MI-BCI system and encourages active motor control [37, 38].
A recent meta-analysis of the neural correlates of action (MI, AO and ME) quantified ‘conjunct’ and ‘contrast’ networks in the cortical and subcortical regions [33]. This analysis, which took advantage of open-source historical data from fMRI studies, reported consistent activation in the premotor, parietal and somatosensory areas for MI, AO and ME. Predicated on such data, researchers have reasoned that performing MI should cause activation of the neural substrates that are also involved in controlling movement and there have been a number of research projects that have used AO in combination with MI in neurorehabilitation [39,40,41] and motor learning studies [42, 43] over the last decade.
One implication of using MI and AO to justify the use of BCI approaches is that great care must be taken with regard to the quality of the environment in which the rehabilitation takes place. While people can learn to modulate their brain rhythms without using motor imagery and there is variability across individuals in their ability to imagine motor actions, MI-driven BCI systems require (by design at least) for patient to imagine a movement. Likewise, AO requires the patients to clearly see the action. This suggests that the richness and vividness of the visual cues provided is an essential part of an effective BCI system. It is also reasonable to assume that feedback is important within these processes and thus the quality of feedback should be considered as essential. Afterall, MI and AO are just tools to modulate brain states [40] and the effectiveness of these tools vary from one stroke patient to another [44]. Finally, motivation is known to play an important role in promoting active participation during therapy [20, 45]. Thus, a good BCI system should incorporate an approach (such as gaming and positive reward) that increases motivation. Recent advances in technology make it far easier to create a rehabilitation environment that provides rich vivid cues, gives salient feedback and is motivating. For example, the rise of immersive technologies, including virtual reality (VR) and augmented reality (AR) platforms [45,46,47], allows for the creation of engaging visual experiences that have the potential to improve a patient’s self-efficacy [48] and thereby encourage the patient to maintain the rehabilitation regime. One specific example of this is visually amplifying the movement made by a patient when the movement is of limited extent so that the patient can see their efforts are producing results [49].
In this review we set out to examine the literature to achieve a better understanding of the current value and potential of BCI-based robotic therapy with three specific objectives:
- (1)Identify how BCI technologies are being utilised in controlling robotic devices for hand rehabilitation. Our focus was on the study design and the tasks that are employed in setting up a BCI-hand robot therapy protocol.
- (2)Document the readiness of BCI systems. Because BCI for rehabilitation is still an emerging field of research, we expected that most studies would be in their proof-of-concept or clinical testing stages of development. Our purpose was to determine the limits of this technology in terms of: (a) resolution of hand MI detection and (b) the degree of robotic control.
- (3)Evaluate the clinical significance of BCI-hand robot systems by looking at the outcome measures in motor recovery and determine if a standard protocol exists for these interventions.
It is important to note that there have been several recent reviews exploring BCI for stroke rehabilitation. For example, Monge-Pereira et al. [50] compiled EEG-based BCI studies for upper limb stroke rehabilitation. Their systematic review (involving 13 clinical studies on stroke and hemiplegic patients) reported on research methodological quality and improvements in the motor abilities of stroke patients. Cervera et al. [51] performed a meta-analysis on the clinical effectiveness of BCI-based stroke therapy among 9 randomised clinical trials (RCT). McConnell et al. [52] undertook a narrative review of 110 robotic devices with brain–machine interfaces for hand rehabilitation post-stroke. These reviews, in general, have reported that such systems provide improvements in both functional and clinical outcomes in pilot studies or trials involving small sample sizes. Thus, the literature indicates that EEG-based BCI are a promising general approach for rehabilitation post-stroke. The current work complements these previous reports by providing the first systematic examination on the use of BCI-robot systems for the rehabilitation of fine motor skills associated with hand movement and profiling these systems from a technical and clinical perspective.[…]
[Abstract +References] Reorganization of Bioelectrical Activity in the Neocortex after Stroke by Rehabilitation Using a Brain–Computer Interface Controlling a Wrist Exoskeleton
Posted by Kostas Pantremenos in Neuroplasticity, Paretic Hand, Rehabilitation robotics on November 29, 2020
The process of the functional rearrangement of the motor cortex of the brain after stroke is due to neuroplasticity, and this underlies motor recovery. Functional magnetic resonance imaging (fMRI) and electroencephalography (EEG) are currently recognized as the most informative methods for studying these processes. The course of the neuroplastic process can be evaluated from the power levels of EEG rhythms during imagination of movements in the paralyzed arm in right-handed patients after stroke in the left hemisphere monitored at different times – before and after courses of neurorehabilitation using a brain–computer interface controlling a wrist exoskeleton. Powerful excitatory interactions in the primary motor cortex and frontoparietal areas in the lesioned and “intact” hemispheres are initially seen, and these probably reflect reorganization of neural networks. Rehabilitation courses were followed by restoration of bioelectrical activity in the primary motor cortex due to recovery of efficient connections with the premotor and superior parietal zones and decreases in the pathological influences of the contralateral hemisphere.
References
- Ang, K. K., Chua, K. S., Phua, K. S., et al., “A randomized controlled trial of EEG-based motor imagery brain–computer interface robotic rehabilitation for stroke,” Clin. EEG Neurosci, 46, No. 4, 310–320 (2015), https://doi.org/https://doi.org/10.1177/1550059414522229.
- Asadi-Pooya, A. A., Dlugos, D., Skidmore, C., and Sperling, M. R., “Atlas of Electroencephalography, 3rd edition,” Epileptic Disord., 19, No. 3, 384 (2017), https://doi.org/https://doi.org/10.1684/epd.2017.0934.
- Bach-y-Rita, P., “Brain plasticity as a basis for recovery of function in humans,” Neuropsychologia, 28, No. 6, 547–554 (1990).CAS Article Google Scholar
- Benali, A., Weiler, E., Benali, Y., et al., “Excitation and inhibition jointly regulate cortical reorganization in adult rats,” J. Neurosci., 28, No. 47, 12284–12293 (2008), https://doi.org/https://doi.org/10.1523/jneurosci.1952-08.2008.
- Berthier, M. L., García-Casares, N., Walsh, S. F., et al., “Recovery from post-stroke aphasia: lessons from brain imaging and implications for rehabilitation and biological treatments,” Discov. Med., 12, No. 65, 275–289 (2011).PubMed Google Scholar
- Bobrov, P. D., Korshakov, A. V., Roshchin, V. Yu., and Frolov, A. A., “A Bayesian approach to brain–computer interfaces based on motor imagery,” Zh. Vyssh. Nerv. Deyat., 62, No. 1, 89–99 (2012).CAS Google Scholar
- Buzsáki, G. and Wang, X. J., “Mechanisms of gamma oscillations,” Annu. Rev. Neurosci., 35, 203–225 (2012), https://doi.org/https://doi.org/10.1146/annurevneuro-062111-150444.
- Cervera, M. A., Soekadar, S. R., Ushiba, J., et al., “Brain–computer interfaces for post-stroke motor rehabilitation: a meta-analysis,” Ann. Clin. Transl. Neurol., 5, No. 5, 651–663 (2018), https://doi.org/https://doi.org/10.1002/acn3.544.
- Chen, C. C., Lee, S. H., Wang, W. J., et al., “EEG-based motor network biomarkers for identifying target patients with stroke for upper limb rehabilitation and its construct validity,” PLoS One, 12, No. 6, e0178822 (2017), https://doi.org/https://doi.org/10.1371/journal.pone.0178822.eCollection.
- Chollet, F., “Pharmacologic approaches to cerebral aging and neuroplasticity: insights from the stroke model,” Dialogues Clin. Neurosci., 15, No. 1, 67–76 (2013).Article Google Scholar
- Cunningham, D. A., Machado, A., Janini, D., et al., “Assessment of inter- hemispheric imbalance using imaging and noninvasive brain stimulation in patients with chronic stroke,” Arch. Phys. Med. Rehabil., 96, No. 4, Supplement, S94–103 (2015), https://doi.org/https://doi.org/10.1016/j.apmr.2014.07.419.
- Damulin, I. V. and Ekusheva, E. V., “Neuroplasticity processes after stroke,” Nevrol. Neiropsikh. Psikhosom., 3, 69–74 (2014), https://doi.org/https://doi.org/10.14412/2074-2711-2014-3-69-74.
- Fries, P., “Rhythms for cognition: communication through coherence,” Neuron, 88, No. 1, 220–235 (2015).CAS Article Google Scholar
- Frolov, A. A., Mokienko, O. A., Lyukmanov, R. Kh., et al., “Preliminary results of a controlled study of the efficacy of hand BCI-exoskeleton technology in poststroke paralysis of the hand,” Vestn. Ross. Gos. Med. Univ., 2, 17–25 (2016).Google Scholar
- Frolov, A., Mokienko, O., Lukmanov, R., et al., “Post-stroke rehabilitation training with a motor-imagery-based brain–computer interface (BCI)-controlled hand exoskeleton: A randomized controlled multicenter trial,” Front. Neurosci., 11, No. 400, 1–11 (2017).Google Scholar
- Gazzola, V. and Keysers, C., “The observation and execution of actions share motor and somatosensory voxels in all tested subjects: single-subject analyses of unsmoothed fMRI data,” Cereb. Cortex, 19, No. 6, 1239–1255 (2009), https://doi.org/https://doi.org/10.1093/cercor/bhn181.
- Jeon, Y., Nam, C. S., Kim, Y.-J., and Whang, M. C., “Event-related (de) synchronization (ERD/ERS) during motor imagery tasks: Implications for brain–computer interfaces,” Int. J. Industr. Ergon., 41, 428–436 (2011).Article Google Scholar
- Kahana, M. J., Seelig, D., and Madsen, J. R., “Theta returns,” Curr. Opin. Neurobiol., 11, No. 6, 739–744 (2001), https://doi.org/https://doi.org/10.1016/S095964388(01)0027861.
- Klimesch, W., “Alpha-band oscillations, attention, and controlled access to stored information,” Trends Cogn. Sci., 16, No. 12, 606–617 (2012), https://doi.org/https://doi.org/10.1016/j.tics.2012.10.007.
- Klimesch, W., Doppelmayr, M., Pachinger, T., and Russegger, H., “Eventrelated desynchronization in the alpha band and the processing of semantic information,” Brain Res. Cogn. Brain Res., 6, No. 2, 83–94 (1997), https://doi.org/https://doi.org/10.1016/S092666410(97)0001869.
- Klimesch, W., Doppelmayr, M., Russegger, H., and Pachinger, T., “Theta band power in the human scalp EEG and the encoding of new information,” Neuroreport, 7, No. 7, 1235–1240 (1996).CAS Article Google Scholar
- Landau, A. N. and Fries, P., “Attention samples stimuli rhythmically,” Curr. Biol., 22, 1000–1004 (2012), https://doi.org/https://doi.org/10.1016/j.cub.2012.03.054.
- Lotze, M., Beutling, W., Loibl, M., et al., “Contralesional motor cortex activation depends on ipsilesional corticospinal tract integrity in well-recovered subcortical stroke patients,” Neurorehabil. Neural Repair, 26, No. 6, 594–603 (2012), https://doi.org/https://doi.org/10.1177/1545968311427706.
- Mitchell, D. J., McNaughton, N., Flanagan, D., and Kirk, I. J., “Frontalmidline theta from the perspective of hippocampal ‘theta,” Prog. Neurobiol., 86, No. 3, 156–185 (2008), https://doi.org/https://doi.org/10.1016/j.pneurobio.2008.09.005.
- Monge-Pereira, E., Ibañez-Pereda, J., Alguacil-Diego, I. M., et al., “Use of electroencephalography brain–computer interface systems as a rehabilitative approach for upper limb function after a stroke: A systematic review,” PM R, 9, No. 9, 918–932 (2017).Article Google Scholar
- Mukhin, K. Yu., Petrukhin, A. S., and Glukhova, L. Yu., Epilepsy. An Atlas of Electroclinical Diagnosis, Alvares Publishing, Moscow (2004).
- Murta, T., Leite, M., Carmichael, D. W., et al., “Electrophysiological correlates of the BOLD signal for EEG-informed fMRI,” Hum. Brain Mapp., 36, No. 1, 391–414 (2015), https://doi.org/https://doi.org/10.1002/hbm.22623.
- Neuper, C., Scherer, R., Reiner, M., and Pfurtscheller, G., “Imagery of motor actions: differential effects of kinesthetic and visual-motor mode of imagery in single-trial EEG,” Cogn. Brain Res., 25, No. 3, 668 (2005).Article Google Scholar
- Nicolo, P., Rizk, S., Magnin, C., et al., “Coherent neural oscillations predict future motor and language improvement after stroke,” Brain, 138, No. 10, 3048–3060 (2015), https://doi.org/https://doi.org/10.1093/brain/awv200.
- Ono, T., Shindo, K., Kawashima, K., et al., “Brain–computer interface with somatosensory feedback improves functional recovery from severehemiplegia due to chronic stroke,” Front. Neuroeng., 7, 19, eCollection 2014 (2014), https://doi.org/https://doi.org/10.3389/fneng.2014.00019.
- Pascual-Leone, A., Amedi, A., Fregni, F., and Merabet, L. B., “The plastic human brain cortex,” Annu. Rev. Neurosci., 28, 377–401 (2005).CAS Article Google Scholar
- Pfurtscheller, G. and Lopes da Silva, F. H., “Event-related EEG/MEG synchronization and desynchronization: basic principles,” Clin. Neurophysiol., 110, 1842–1857 (1999).CAS Article Google Scholar
- Pichiorri, F., Petti, M., Caschera, S., et al., “An EEG index of sensorimotor interhemispheric coupling after unilateral stroke: clinical and neurophysiological study,” Eur. J. Neurosci., 47, No. 2, 158–163 (2018), https://doi.org/https://doi.org/10.1111/ejn.13797.
- Prabhakaran, S., Zarahn, E., Riley, C., et al., “Inter-individual variability in the capacity for motor recovery after ischemic stroke,” Neurorehabil. Neural Repair, 22, No. 1, 64–71 (2008).Article Google Scholar
- Rehme, A. K., Eickhoff, S. B., Wang, L. E., et al., “Dynamic causal modeling of cortical activity from the acute to the chronic stage after stroke,” Neuroimage, 55, No. 3, 1147–1158 (2011), https://doi.org/https://doi.org/10.1016/j.neuroimage.2011.01.014.
- Remsik, A., Young, B., Vermilyea, R., et al., “A review of the progression and future implications of brain–computer interface therapies for restoration of distal upper extremity motor function after stroke,” Expert Rev. Med. Devices, 13, No. 5, 445–454 (2016), https://doi.org/https://doi.org/10.1080/17434440.2016.1174572.
- Rizzolatti, G. and Sinigaglia, C., “The functional role of the parieto-frontal mirror circuit: interpretations and misinterpretations,” Nat. Rev. Neurosci., 11, No. 4, 264–274 (2010), https://doi.org/https://doi.org/10.1038/nrn2805.
- Rossiter, H. E., Davis, E. M., Clark, E. V., et al., “Beta oscillations reflect changes in motor cortex inhibition in healthy ageing,” NeuroImage, 91, 360–365 (2014).Article Google Scholar
- Sato, J. R., Rondinoni, C., Sturzbecher, M., et al., “From EEG to BOLD: brain mapping and estimating transfer functions in simultaneous EEG-fMRI acquisitions,” Neuroimage, 50, No. 4, 1416–1426 (2010), https://doi.org/https://doi.org/10.1016/j.neuroimage.2010.01.075.
- Sauseng, P., Griesmayr, B., Freunberger, R., and Klimesch, W., “Control mechanisms in working memory: a possible function of EEG theta oscillations,” Neurosci. Biobehav. Rev., 34, No. 7, 1015–1022 (2010), https://doi.org/https://doi.org/10.1016/j.neubiorev.2009.12.006.
- Stinear, C. M. and Ward, N. S., “How useful is imaging in predicting outcomes in stroke rehabilitation?” Int. J. Stroke, 8, No. 1, 33–37 (2013), https://doi.org/https://doi.org/10.1111/j.1747-4949.2012.00970.x.
- Stinear, C. M., “Prediction of motor recovery after stroke: advances in biomarkers,” Lancet Neurol., 16, No. 10, 826–836 (2017), https://doi.org/https://doi.org/10.1016/S1474-4422(17)30283-1.
- Stroganova, T. A., Orekhova, E. V., and Posikera, I. N., “The theta rhythm of the infant EEG and the development of the mechanisms of voluntary control of attention in the 2nd half of the first year of life,” Zh. Vyssh. Nerv. Deyat., 48, No. 6, 945–964 (1998).CAS Google Scholar
- Suffczynski, P., Kalitzin, S., Pfurtscheller, G., and Lopes da Silva, F. H., “Computational model of thalamo-cortical networks: dynamical control of alpha rhythms in relation to focal attention,” Int. J. Psychophysiol., 43, No. 1, 25–40 (2001), https://doi.org/https://doi.org/10.1016/S016768760(01)0017765.
- Takeuchi, N., Oouchida, Y., and Izumi, S., “Motor control and neural plasticity through interhemispheric interactions,” Neural Plast., Art. 823285 (2012), https://doi.org/https://doi.org/10.1155/2012/823285.
- Vinogradova, O. S., Kitchigina, V. F., and Zenchenko, C. I., “Pacemaker neurons of the forebrain medical septal area and theta rhythm of the hippocampus,” Membr. Cell Biol., 11, No. 6, 715–725 (1998).CAS PubMed Google Scholar
- Yamawaki, N., Stanford, I. M., Hall, S. D., and Woodhall, G. L., “Pharmacologically induced and stimulus evoked rhythmic neuronal oscillatory activity in the primary motor cortex in vitro,” Neuroscience, 151, No. 2, 386–395 (2008).CAS Article Google Scholar
- Zenkov, L. R., Clinical Epileptology (with elements of neurophysiology): Guidelines for Doctors, MIA, Moscow (2010), 2nd ed.