Posts Tagged prefrontal cortex
[BLOG POST] How Trauma Impairs Brain Function – Video
Posted by Kostas Pantremenos in TBI, Video on September 6, 2021
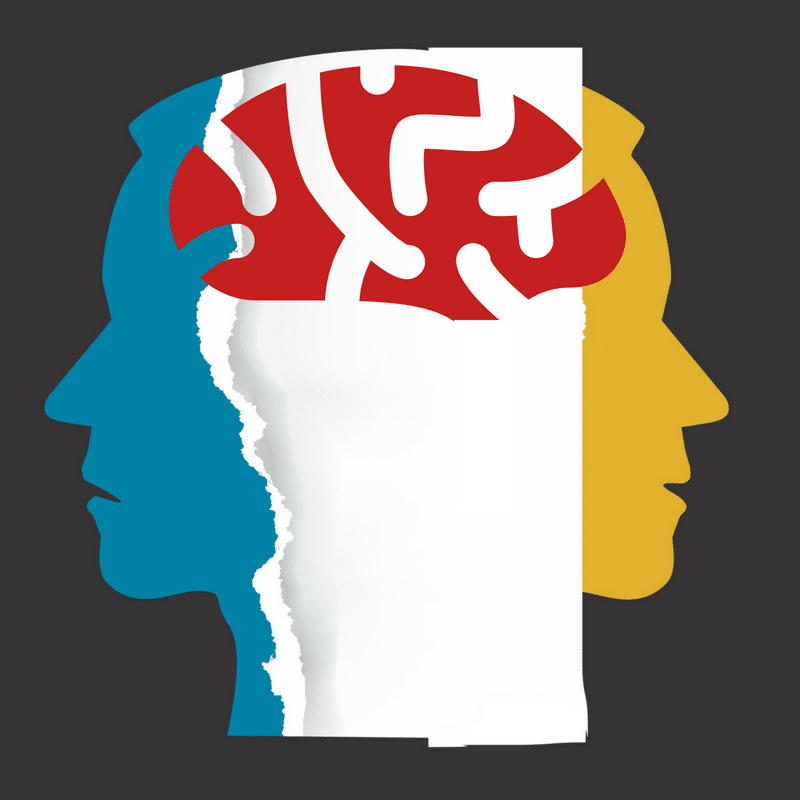
Trauma can actually alter the function of your brain during the stressful event and result in lasting changes in certain brain regions. These changes can impair cognitive function and memory encoding and recall at the moment and in the future. Recent neuroscientific findings in this area have real implications for the victims of crime and the legal system. The science of epigenetics is even showing that the effects of trauma can be passed down through genes to future generations.
Your Thinking Brain Shuts Down
At any given moment, the prefrontal cortex of your brain is primarily in charge of conscious thought and behavior. This is the area of higher level brain function allowing you to focus attention, think rationally, and inhibit impulses. In states of extreme stress, fear, or terror, your prefrontal cortex becomes impaired. A surge of stress chemicals basically shuts it down.
One study, “Stress signaling pathways that impair prefrontal cortex structure and function”, explains it like this:
The prefrontal cortex (PFC) – the most evolved brain region – subserves our highest-order cognitive abilities. However, it is also the brain region that is most sensitive to the detrimental effects of stress exposure. Even quite mild acute uncontrollable stress can cause a rapid and dramatic loss of prefrontal cognitive abilities, and more prolonged stress exposure causes architectural changes in prefrontal dendrites. ays can lead to symptoms of profound prefrontal cortical dysfunction in mental illness.”
You probably can recall a time when you were in an emergency situation, one that necessitated a clear head. Just when you needed it the most, you most likely found that your thinking brain was completely unavailable. When the executive center of your brain goes offline, you are less able to direct your attention, make any kind of sense of what is happening before you, and less able to store the memories or recall the experience in an orderly way.
Fear Takes Over Your Brain
At some point during a traumatic event, fear kicks in and your amygdala starts running the show. Your amygdala are small almond-shaped masses (there are two of them) located deep in the temporal lobe of your brain. The amygdala is an ancient limbic system structure primarily responsible for processing memory, decision-making, motivation, and emotional reactions – most significantly, those related to survival. You have your amygdala to blame and thank for primal emotions, such as fear, anger, and pleasure.
The amygdala acts as your brain’s threat radar. When the amygdala sounds the alarm, your body responds with an almost instantaneous sequence of hormonal and physiological changes preparing you to fight for your life or flee. When this happens, most of your physical and mental resources get allocated to making sure you survive.
Once your fear circuitry takes over, it determines where your attention goes. You could zero in on the imminent threat or it could direct your attention completely away from the threat. In this case, you might end up focusing on seemingly trivial details. Either way, what gets processed by your brain tends to be fragmentary sensations, not the pertinent elements of the unfolding experience. What gets your attention is what is going to be encoded into memory.
Trauma Impairs Memory
Activation of your brain’s fear circuitry also impairs the functioning of the hippocampus and memory storage. The hippocampus encodes experiences into short-term memory and later can process them as long-term memories. Fear interferes with the ability of the hippocampus to encode and store “contextual information,” like the various details of an experience. Fear also causes time sequencing of encoded information to be altered. This means that you may not remember events happening in the actual order in which they occurred.
Research shows that the amygdala plays a crucial role in the formation and storage of emotional memories, resulting in what is known as “emotional learning.” While both positive and negative emotional memory storage may be facilitated by the amygdala, studies confirm that it puts special emphasis on threats, which results in “fear conditioning.”
Recent studies suggest that the hippocampus goes into a super-encoding state briefly right before and after fear kicks in. For example, somebody may remember every little detail about what happened right before and after a traumatic event including contextual details and the exact sequence of events. However, they may have very fragmented and incomplete memories of the actual happening.Trauma can alter the function of your brain during the event and result in lasting changes.
Real-Life Implications
Neuroscientific research is shedding light on a new understanding of how brain function is altered in traumatic situations. This new information has far-reaching implications for the legal system. For example, discrepancies in a victim’s story, should not be interpreted as lies, but as their brain’s response to extreme trauma.
The article, Why Rape and Trauma Survivors Have Fragmented and Incomplete Memories, furthers the point:
These advances in our understanding of the impact of trauma on the brain have enormous implications for the criminal justice system. It is not reasonable to expect a trauma survivor – whether a rape victim, a police officer or a soldier – to recall traumatic events the way they would recall their wedding day. They will remember some aspects of the experience in exquisitely painful detail. Indeed, they may spend decades trying to forget them. They will remember other aspects not at all, or only in jumbled and confused fragments. Such is the nature of terrifying experiences, and it is a nature that we cannot ignore.”
Here’s a really good video depicting Trauma and the Brain:
[Abstract +References] Reorganization of Bioelectrical Activity in the Neocortex after Stroke by Rehabilitation Using a Brain–Computer Interface Controlling a Wrist Exoskeleton
Posted by Kostas Pantremenos in Neuroplasticity, Paretic Hand, Rehabilitation robotics on November 29, 2020
The process of the functional rearrangement of the motor cortex of the brain after stroke is due to neuroplasticity, and this underlies motor recovery. Functional magnetic resonance imaging (fMRI) and electroencephalography (EEG) are currently recognized as the most informative methods for studying these processes. The course of the neuroplastic process can be evaluated from the power levels of EEG rhythms during imagination of movements in the paralyzed arm in right-handed patients after stroke in the left hemisphere monitored at different times – before and after courses of neurorehabilitation using a brain–computer interface controlling a wrist exoskeleton. Powerful excitatory interactions in the primary motor cortex and frontoparietal areas in the lesioned and “intact” hemispheres are initially seen, and these probably reflect reorganization of neural networks. Rehabilitation courses were followed by restoration of bioelectrical activity in the primary motor cortex due to recovery of efficient connections with the premotor and superior parietal zones and decreases in the pathological influences of the contralateral hemisphere.
References
- Ang, K. K., Chua, K. S., Phua, K. S., et al., “A randomized controlled trial of EEG-based motor imagery brain–computer interface robotic rehabilitation for stroke,” Clin. EEG Neurosci, 46, No. 4, 310–320 (2015), https://doi.org/https://doi.org/10.1177/1550059414522229.
- Asadi-Pooya, A. A., Dlugos, D., Skidmore, C., and Sperling, M. R., “Atlas of Electroencephalography, 3rd edition,” Epileptic Disord., 19, No. 3, 384 (2017), https://doi.org/https://doi.org/10.1684/epd.2017.0934.
- Bach-y-Rita, P., “Brain plasticity as a basis for recovery of function in humans,” Neuropsychologia, 28, No. 6, 547–554 (1990).CAS Article Google Scholar
- Benali, A., Weiler, E., Benali, Y., et al., “Excitation and inhibition jointly regulate cortical reorganization in adult rats,” J. Neurosci., 28, No. 47, 12284–12293 (2008), https://doi.org/https://doi.org/10.1523/jneurosci.1952-08.2008.
- Berthier, M. L., García-Casares, N., Walsh, S. F., et al., “Recovery from post-stroke aphasia: lessons from brain imaging and implications for rehabilitation and biological treatments,” Discov. Med., 12, No. 65, 275–289 (2011).PubMed Google Scholar
- Bobrov, P. D., Korshakov, A. V., Roshchin, V. Yu., and Frolov, A. A., “A Bayesian approach to brain–computer interfaces based on motor imagery,” Zh. Vyssh. Nerv. Deyat., 62, No. 1, 89–99 (2012).CAS Google Scholar
- Buzsáki, G. and Wang, X. J., “Mechanisms of gamma oscillations,” Annu. Rev. Neurosci., 35, 203–225 (2012), https://doi.org/https://doi.org/10.1146/annurevneuro-062111-150444.
- Cervera, M. A., Soekadar, S. R., Ushiba, J., et al., “Brain–computer interfaces for post-stroke motor rehabilitation: a meta-analysis,” Ann. Clin. Transl. Neurol., 5, No. 5, 651–663 (2018), https://doi.org/https://doi.org/10.1002/acn3.544.
- Chen, C. C., Lee, S. H., Wang, W. J., et al., “EEG-based motor network biomarkers for identifying target patients with stroke for upper limb rehabilitation and its construct validity,” PLoS One, 12, No. 6, e0178822 (2017), https://doi.org/https://doi.org/10.1371/journal.pone.0178822.eCollection.
- Chollet, F., “Pharmacologic approaches to cerebral aging and neuroplasticity: insights from the stroke model,” Dialogues Clin. Neurosci., 15, No. 1, 67–76 (2013).Article Google Scholar
- Cunningham, D. A., Machado, A., Janini, D., et al., “Assessment of inter- hemispheric imbalance using imaging and noninvasive brain stimulation in patients with chronic stroke,” Arch. Phys. Med. Rehabil., 96, No. 4, Supplement, S94–103 (2015), https://doi.org/https://doi.org/10.1016/j.apmr.2014.07.419.
- Damulin, I. V. and Ekusheva, E. V., “Neuroplasticity processes after stroke,” Nevrol. Neiropsikh. Psikhosom., 3, 69–74 (2014), https://doi.org/https://doi.org/10.14412/2074-2711-2014-3-69-74.
- Fries, P., “Rhythms for cognition: communication through coherence,” Neuron, 88, No. 1, 220–235 (2015).CAS Article Google Scholar
- Frolov, A. A., Mokienko, O. A., Lyukmanov, R. Kh., et al., “Preliminary results of a controlled study of the efficacy of hand BCI-exoskeleton technology in poststroke paralysis of the hand,” Vestn. Ross. Gos. Med. Univ., 2, 17–25 (2016).Google Scholar
- Frolov, A., Mokienko, O., Lukmanov, R., et al., “Post-stroke rehabilitation training with a motor-imagery-based brain–computer interface (BCI)-controlled hand exoskeleton: A randomized controlled multicenter trial,” Front. Neurosci., 11, No. 400, 1–11 (2017).Google Scholar
- Gazzola, V. and Keysers, C., “The observation and execution of actions share motor and somatosensory voxels in all tested subjects: single-subject analyses of unsmoothed fMRI data,” Cereb. Cortex, 19, No. 6, 1239–1255 (2009), https://doi.org/https://doi.org/10.1093/cercor/bhn181.
- Jeon, Y., Nam, C. S., Kim, Y.-J., and Whang, M. C., “Event-related (de) synchronization (ERD/ERS) during motor imagery tasks: Implications for brain–computer interfaces,” Int. J. Industr. Ergon., 41, 428–436 (2011).Article Google Scholar
- Kahana, M. J., Seelig, D., and Madsen, J. R., “Theta returns,” Curr. Opin. Neurobiol., 11, No. 6, 739–744 (2001), https://doi.org/https://doi.org/10.1016/S095964388(01)0027861.
- Klimesch, W., “Alpha-band oscillations, attention, and controlled access to stored information,” Trends Cogn. Sci., 16, No. 12, 606–617 (2012), https://doi.org/https://doi.org/10.1016/j.tics.2012.10.007.
- Klimesch, W., Doppelmayr, M., Pachinger, T., and Russegger, H., “Eventrelated desynchronization in the alpha band and the processing of semantic information,” Brain Res. Cogn. Brain Res., 6, No. 2, 83–94 (1997), https://doi.org/https://doi.org/10.1016/S092666410(97)0001869.
- Klimesch, W., Doppelmayr, M., Russegger, H., and Pachinger, T., “Theta band power in the human scalp EEG and the encoding of new information,” Neuroreport, 7, No. 7, 1235–1240 (1996).CAS Article Google Scholar
- Landau, A. N. and Fries, P., “Attention samples stimuli rhythmically,” Curr. Biol., 22, 1000–1004 (2012), https://doi.org/https://doi.org/10.1016/j.cub.2012.03.054.
- Lotze, M., Beutling, W., Loibl, M., et al., “Contralesional motor cortex activation depends on ipsilesional corticospinal tract integrity in well-recovered subcortical stroke patients,” Neurorehabil. Neural Repair, 26, No. 6, 594–603 (2012), https://doi.org/https://doi.org/10.1177/1545968311427706.
- Mitchell, D. J., McNaughton, N., Flanagan, D., and Kirk, I. J., “Frontalmidline theta from the perspective of hippocampal ‘theta,” Prog. Neurobiol., 86, No. 3, 156–185 (2008), https://doi.org/https://doi.org/10.1016/j.pneurobio.2008.09.005.
- Monge-Pereira, E., Ibañez-Pereda, J., Alguacil-Diego, I. M., et al., “Use of electroencephalography brain–computer interface systems as a rehabilitative approach for upper limb function after a stroke: A systematic review,” PM R, 9, No. 9, 918–932 (2017).Article Google Scholar
- Mukhin, K. Yu., Petrukhin, A. S., and Glukhova, L. Yu., Epilepsy. An Atlas of Electroclinical Diagnosis, Alvares Publishing, Moscow (2004).
- Murta, T., Leite, M., Carmichael, D. W., et al., “Electrophysiological correlates of the BOLD signal for EEG-informed fMRI,” Hum. Brain Mapp., 36, No. 1, 391–414 (2015), https://doi.org/https://doi.org/10.1002/hbm.22623.
- Neuper, C., Scherer, R., Reiner, M., and Pfurtscheller, G., “Imagery of motor actions: differential effects of kinesthetic and visual-motor mode of imagery in single-trial EEG,” Cogn. Brain Res., 25, No. 3, 668 (2005).Article Google Scholar
- Nicolo, P., Rizk, S., Magnin, C., et al., “Coherent neural oscillations predict future motor and language improvement after stroke,” Brain, 138, No. 10, 3048–3060 (2015), https://doi.org/https://doi.org/10.1093/brain/awv200.
- Ono, T., Shindo, K., Kawashima, K., et al., “Brain–computer interface with somatosensory feedback improves functional recovery from severehemiplegia due to chronic stroke,” Front. Neuroeng., 7, 19, eCollection 2014 (2014), https://doi.org/https://doi.org/10.3389/fneng.2014.00019.
- Pascual-Leone, A., Amedi, A., Fregni, F., and Merabet, L. B., “The plastic human brain cortex,” Annu. Rev. Neurosci., 28, 377–401 (2005).CAS Article Google Scholar
- Pfurtscheller, G. and Lopes da Silva, F. H., “Event-related EEG/MEG synchronization and desynchronization: basic principles,” Clin. Neurophysiol., 110, 1842–1857 (1999).CAS Article Google Scholar
- Pichiorri, F., Petti, M., Caschera, S., et al., “An EEG index of sensorimotor interhemispheric coupling after unilateral stroke: clinical and neurophysiological study,” Eur. J. Neurosci., 47, No. 2, 158–163 (2018), https://doi.org/https://doi.org/10.1111/ejn.13797.
- Prabhakaran, S., Zarahn, E., Riley, C., et al., “Inter-individual variability in the capacity for motor recovery after ischemic stroke,” Neurorehabil. Neural Repair, 22, No. 1, 64–71 (2008).Article Google Scholar
- Rehme, A. K., Eickhoff, S. B., Wang, L. E., et al., “Dynamic causal modeling of cortical activity from the acute to the chronic stage after stroke,” Neuroimage, 55, No. 3, 1147–1158 (2011), https://doi.org/https://doi.org/10.1016/j.neuroimage.2011.01.014.
- Remsik, A., Young, B., Vermilyea, R., et al., “A review of the progression and future implications of brain–computer interface therapies for restoration of distal upper extremity motor function after stroke,” Expert Rev. Med. Devices, 13, No. 5, 445–454 (2016), https://doi.org/https://doi.org/10.1080/17434440.2016.1174572.
- Rizzolatti, G. and Sinigaglia, C., “The functional role of the parieto-frontal mirror circuit: interpretations and misinterpretations,” Nat. Rev. Neurosci., 11, No. 4, 264–274 (2010), https://doi.org/https://doi.org/10.1038/nrn2805.
- Rossiter, H. E., Davis, E. M., Clark, E. V., et al., “Beta oscillations reflect changes in motor cortex inhibition in healthy ageing,” NeuroImage, 91, 360–365 (2014).Article Google Scholar
- Sato, J. R., Rondinoni, C., Sturzbecher, M., et al., “From EEG to BOLD: brain mapping and estimating transfer functions in simultaneous EEG-fMRI acquisitions,” Neuroimage, 50, No. 4, 1416–1426 (2010), https://doi.org/https://doi.org/10.1016/j.neuroimage.2010.01.075.
- Sauseng, P., Griesmayr, B., Freunberger, R., and Klimesch, W., “Control mechanisms in working memory: a possible function of EEG theta oscillations,” Neurosci. Biobehav. Rev., 34, No. 7, 1015–1022 (2010), https://doi.org/https://doi.org/10.1016/j.neubiorev.2009.12.006.
- Stinear, C. M. and Ward, N. S., “How useful is imaging in predicting outcomes in stroke rehabilitation?” Int. J. Stroke, 8, No. 1, 33–37 (2013), https://doi.org/https://doi.org/10.1111/j.1747-4949.2012.00970.x.
- Stinear, C. M., “Prediction of motor recovery after stroke: advances in biomarkers,” Lancet Neurol., 16, No. 10, 826–836 (2017), https://doi.org/https://doi.org/10.1016/S1474-4422(17)30283-1.
- Stroganova, T. A., Orekhova, E. V., and Posikera, I. N., “The theta rhythm of the infant EEG and the development of the mechanisms of voluntary control of attention in the 2nd half of the first year of life,” Zh. Vyssh. Nerv. Deyat., 48, No. 6, 945–964 (1998).CAS Google Scholar
- Suffczynski, P., Kalitzin, S., Pfurtscheller, G., and Lopes da Silva, F. H., “Computational model of thalamo-cortical networks: dynamical control of alpha rhythms in relation to focal attention,” Int. J. Psychophysiol., 43, No. 1, 25–40 (2001), https://doi.org/https://doi.org/10.1016/S016768760(01)0017765.
- Takeuchi, N., Oouchida, Y., and Izumi, S., “Motor control and neural plasticity through interhemispheric interactions,” Neural Plast., Art. 823285 (2012), https://doi.org/https://doi.org/10.1155/2012/823285.
- Vinogradova, O. S., Kitchigina, V. F., and Zenchenko, C. I., “Pacemaker neurons of the forebrain medical septal area and theta rhythm of the hippocampus,” Membr. Cell Biol., 11, No. 6, 715–725 (1998).CAS PubMed Google Scholar
- Yamawaki, N., Stanford, I. M., Hall, S. D., and Woodhall, G. L., “Pharmacologically induced and stimulus evoked rhythmic neuronal oscillatory activity in the primary motor cortex in vitro,” Neuroscience, 151, No. 2, 386–395 (2008).CAS Article Google Scholar
- Zenkov, L. R., Clinical Epileptology (with elements of neurophysiology): Guidelines for Doctors, MIA, Moscow (2010), 2nd ed.
[ARTICLE] Enhancing cognitive control training with transcranial direct current stimulation: a systematic parameter study – Full Text
Posted by Kostas Pantremenos in Cognitive Rehabilitation, REHABILITATION, tDCS/rTMS on August 9, 2020
Abstract
Background
Cognitive control (CC) is an important prerequisite for goal-directed behaviour and efficient information processing. Impaired CC is associated with reduced prefrontal cortex activity and various mental disorders, but may be effectively tackled by transcranial direct current stimulation (tDCS)-enhanced training. However, study data are inconsistent as efficacy depends on stimulation parameters whose implementations vary widely between studies.
Objective
We systematically tested various tDCS parameter effects (anodal/cathodal polarity, 1/2 mA stimulation intensity, left/right prefrontal cortex hemisphere) on a six-session CC training combined with tDCS.
Methods
Nine groups of healthy humans (male/female) received either anodal/cathodal tDCS of 1/2 mA over the left/right PFC or sham stimulation, simultaneously with a CC training (modified adaptive Paced Auditory Serial Addition Task [PASAT]). Subjects trained thrice per week (19 min each) for two weeks. We assessed performance progress in the PASAT before, during, and after training. Using a hierarchical approach, we incrementally narrowed down on optimal stimulation parameters supporting CC. Long-term CC effects as well as transfer effects in a flanker task were assessed after the training period as well as three months later.
Results
Compared to sham stimulation, anodal but not cathodal tDCS improved performance gains. This was only valid for 1 mA stimulation intensity and particularly detected when applied to the left PFC.
Conclusions
Our results confirm beneficial, non-linear effects of anodal tDCS on cognitive training in a large sample of healthy subjects. The data consolidate the basis for further development of functionally targeted tDCS, supporting cognitive control training in mental disorders and guiding further development of clinical interventions.
Introduction
Continuously changing environments require dynamic adaptation by means of filtering and evaluating internal and external stimuli to orchestrate goal-directed behaviour. This is especially important for situations in which distractions might influence efficient responses. Important information is maintained, while non-relevant stimuli must be suppressed or ignored. Dysfunctions of cognitive control (CC) processes are at the core of many psychopathological conditions [1,2], comprise the intentional selection of thoughts, emotions, and behaviours based on current task demands [3] involving functions of attention, memory, and emotional control [4], and are associated with altered patterns of brain activation [5,6]. The prefrontal cortex (PFC), particularly the dorsolateral prefrontal cortex (dlPFC), is known to be highly involved in CC processes [7] by means of processes related to working memory [8], encoding of task relevant rules and responses [9], and emotion regulation [10].
Transcranial direct current stimulation (tDCS) has been put forward as a means to influence these processes by modulating the likelihood of neuronal firing in response to a stimulus [11]. At the macroscopic level, within the common and safe range of stimulation parameters (1–2 mA, up to 30 min of stimulation [12]), it is supposed that anodal tDCS predominantly enhances, while cathodal tDCS mainly reduces the excitability and spontaneous activity of the targeted and connected areas [13]. This polarity-dependent modulation of brain activity by tDCS has a remarkable potential to influence corresponding cognition and behaviour [[14], [15], [16]]. However, tDCS does not induce cortical activity per se. It develops its effects particularly in interaction with spontaneous neuronal activity [17,18]. This activity-dependent influence on brain networks allows for a ‘functional targeting’ of stimulation when tDCS is directly coupled with the respective cognitive or behavioural process [19], where the target regions are activated (i.e. by a task) and further specifically modulated by the stimulation [20]. Correspondingly, tDCS effects have been found especially in neuronal correlates of task features that were active during stimulation [21]. Therefore, the combination of tDCS with task training is suggested to have a synergistic ‘neuroenhancing’ effect that is currently subject of extensive research [[22], [23], [24], [25]]. However, available data are still inconsistent as efficacy depends on stimulation parameters that vary widely between studies. For a meaningful clinical application, a sustainable enhancement of adaptive plasticity would be most desirable [26]. Based on this notion, a specific activation of the CC network and concomitant tDCS holds promise to provide new treatment strategies for cognitive and behavioural disorders [[27], [28], [29]]. In a plethora of studies, stimulation has already shown to enhance CC by changing emotion regulation processes [30], improving frustration tolerance [31], modulating emotional vulnerability [32], dissolving attentional biases [33], augmenting working memory training [16], and increasing multitasking capacity [34]. However, reliability of results and the plausibility of approaches leaves room for improvement, not at least because studies often yield varying results even for similar tasks [[35], [36], [37], [38]]. Therefore, reliable knowledge about the efficacy of parameter settings is mandatory for further advancements [39].
To this aim, we systematically tested different standard stimulation parameters (anodal/cathodal tDCS with 1/2 mA to the left/right dlPFC) in 162 healthy subjects, combining repeated CC training (6 sessions within 2 weeks) with tDCS, and additionally analysed pre- and post-training assessments. We applied a modified adaptive paced auditory serial addition task (PASAT) to challenge and train CC [40]. This task requires continuous updating of working memory with parallel distracting performance feedback; it is known to activate CC [31], critically involves resources within the PFC [41], and adapts task difficulty to individual performance [42]. We hypothesized that adding anodal but not cathodal tDCS to PASAT-induced neuronal activity of the dlPFC [43,44] can enhance cognitive training effects [45,46], improve performance of the PASAT or similar, even more challenging tasks [16,31,[46], [47], [48], [49], [50]], and that higher stimulation intensity does not increase efficacy [51]. Furthermore, we wanted to test if the laterality of stimulation matters. Therefore, PASAT performance under eight different tDCS conditions (combined N = 119) was compared to a sham intervention group (N = 43). Analyses were conducted hierarchically, allowing us to narrow down the responsible factors for the most efficient combination of CC training and tDCS.[…]
[TED Talk] The Brain-Changing Effects of Exercise
Posted by Kostas Pantremenos in Cognitive Rehabilitation, Educational on December 19, 2018
What’s the most transformative thing that you can do for your brain today? Exercise! says neuroscientist Wendy Suzuki. Get inspired to go to the gym as Suzuki discusses the science of how working out boosts your mood and memory — and protects your brain against neurodegenerative diseases like Alzheimer’s.
This talk was presented at an official TED conference, and was featured by our editors on the home page.
Transcript
What if I told you there was something that you can do right now that would have an immediate, positive benefit for your brain including your mood and your focus? And what if I told you that same thing could actually last a long time and protect your brain from different conditions like depression, Alzheimer’s disease or dementia. Would you do it? Yes!
I am talking about the powerful effects of physical activity. Simply moving your body,has immediate, long-lasting and protective benefits for your brain. And that can last for the rest of your life. So what I want to do today is tell you a story about how I used my deep understanding of neuroscience, as a professor of neuroscience, to essentially do an experiment on myself in which I discovered the science underlying why exercise is the most transformative thing that you can do for your brain today. Now, as a neuroscientist, I know that our brains, that is the thing in our head right now, that is the most complex structure known to humankind. But it’s one thing to talk about the brain,and it’s another to see it.
So here is a real preserved human brain. And it’s going to illustrate two key areas that we are going to talk about today. The first is the prefrontal cortex, right behind your forehead, critical for things like decision-making, focus, attention and your personality.The second key area is located in the temporal lobe, shown right here. You have two temporal lobes in your brain, the right and the left, and deep in the temporal lobe is a key structure critical for your ability to form and retain new long-term memories for facts and events. And that structure is called the hippocampus. So I’ve always been fascinated with the hippocampus. How could it be that an event that lasts just a moment, say, your first kiss, or the moment your first child was born, can form a memory that has changed your brain, that lasts an entire lifetime? That’s what I want to understand. I wanted to start and record the activity of individual brain cells in the hippocampus as subjects were forming new memories. And essentially try and decode how those brief bursts of electrical activity, which is how neurons communicate with each other, how those brief bursts either allowed us to form a new memory, or did not.
But a few years ago, I did something very unusual in science. As a full professor of neural science, I decided to completely switch my research program. Because I encountered something that was so amazing, with the potential to change so many lives that I had to study it. I discovered and I experienced the brain-changing effects of exercise. And I did it in a completely inadvertent way. I was actually at the height of all the memory work that I was doing — data was pouring in, I was becoming known in my field for all of this memory work. And it should have been going great. It was, scientifically. But when I stuck my head out of my lab door, I noticed something. I had no social life. I spent too much time listening to those brain cells in a dark room, by myself. (Laughter) I didn’t move my body at all. I had gained 25 pounds. And actually, it took me many years to realize it, I was actually miserable. And I shouldn’t be miserable. And I went on a river-rafting trip — by myself, because I had no social life.And I came back —
thinking, “Oh, my God, I was the weakest person on that trip.” And I came back with a mission. I said, “I’m never going to feel like the weakest person on a river-rafting trip again.” And that’s what made me go to the gym. And I focused my type-A personalityon going to all the exercise classes at the gym. I tried everything. I went to kickbox, dance, yoga, step class, and at first it was really hard. But what I noticed is that after every sweat-inducing workout that I tried, I had this great mood boost and this great energy boost. And that’s what kept me going back to the gym. Well, I started feeling stronger. I started feeling better, I even lost that 25 pounds.
And now, fast-forward a year and a half into this regular exercise program and I noticed something that really made me sit up and take notice. I was sitting at my desk, writing a research grant, and a thought went through my mind that had never gone through my mind before. And that thought was, “Gee, grant-writing is going well today.” And all the scientists —
yeah, all the scientists always laugh when I say that, because grant-writing never goes well. It is so hard; you’re always pulling your hair out, trying to come up with that million-dollar-winning idea. But I realized that the grant-writing was going well,because I was able to focus and maintain my attention for longer than I had before.And my long-term memory — what I was studying in my own lab — seemed to be better in me. And that’s when I put it together.
Maybe all that exercise that I had included and added to my life was changing my brain. Maybe I did an experiment on myself without even knowing it. So as a curious neuroscientist, I went to the literature to see what I could find about what we knewabout the effects of exercise on the brain. And what I found was an exciting and a growing literature that was essentially showing everything that I noticed in myself.Better mood, better energy, better memory, better attention. And the more I learned,the more I realized how powerful exercise was. Which eventually led me to the big decision to completely shift my research focus. And so now, after several years of really focusing on this question, I’ve come to the following conclusion: that exercise is the most transformative thing that you can do for your brain today for the following three reasons.
Number one: it has immediate effects on your brain. A single workout that you do will immediately increase levels of neurotransmitters like dopamine, serotonin and noradrenaline. That is going to increase your mood right after that workout, exactly what I was feeling. My lab showed that a single workout can improve your ability to shift and focus attention, and that focus improvement will last for at least two hours.And finally, studies have shown that a single workout will improve your reaction timeswhich basically means that you are going to be faster at catching that cup of Starbucks that falls off the counter, which is very, very important.
But these immediate effects are transient, they help you right after. What you have to do is do what I did, that is change your exercise regime, increase your cardiorespiratory function, to get the long-lasting effects. And these effects are long-lasting because exercise actually changes the brain’s anatomy, physiology and function. Let’s start with my favorite brain area, the hippocampus. The hippocampus —or exercise actually produces brand new brain cells, new brain cells in the hippocampus, that actually increase its volume, as well as improve your long-term memory, OK? And that including in you and me.
Number two: the most common finding in neuroscience studies, looking at effects of long-term exercise, is improved attention function dependent on your prefrontal cortex. You not only get better focus and attention, but the volume of the hippocampus increases as well. And finally, you not only get immediate effects of mood with exercise but those last for a long time. So you get long-lasting increases in those good mood neurotransmitters.
But really, the most transformative thing that exercise will do is its protective effects on your brain. Here you can think about the brain like a muscle. The more you’re working out, the bigger and stronger your hippocampus and prefrontal cortex gets. Why is that important? Because the prefrontal cortex and the hippocampus are the two areas that are most susceptible to neurodegenerative diseases and normal cognitive decline in aging. So with increased exercise over your lifetime, you’re not going to cure dementia or Alzheimer’s disease, but what you’re going to do is you’re going to create the strongest, biggest hippocampus and prefrontal cortex so it takes longer for these diseases to actually have an effect. You can think of exercise, therefore, as a supercharged 401K for your brain, OK? And it’s even better, because it’s free.
And so I’m going to tell you the answer to that question. First, good news: you don’t have to become a triathlete to get these effects. The rule of thumb is you want to get three to four times a week exercise minimum 30 minutes an exercise session, and you want to get aerobic exercise in. That is, get your heart rate up. And the good news is, you don’t have to go to the gym to get a very expensive gym membership.Add an extra walk around the block in your power walk. You see stairs — take stairs.And power-vacuuming can be as good as the aerobics class that you were going to take at the gym.
So I’ve gone from memory pioneer to exercise explorer. From going into the innermost workings of the brain, to trying to understand how exercise can improve our brain function, and my goal in my lab right now is to go beyond that rule of thumb that I just gave you — three to four times a week, 30 minutes. I want to understand the optimum exercise prescription for you, at your age, at your fitness level, for your genetic background, to maximize the effects of exercise today and also to improve your brain and protect your brain the best for the rest of your life.
[ARTICLE] Near-Infrared Spectroscopy in Gait Disorders – Is it Time to Begin? – Full Text
Posted by Kostas Pantremenos in Gait Rehabilitation - Foot Drop on February 17, 2017
Abstract
Walking is a complex motor behavior with a special relevance in clinical neurology. Many neurological diseases, such as Parkinson’s disease and stroke, are characterized by gait disorders whose neurofunctional correlates are poorly investigated. Indeed, the analysis of real walking with the standard neuroimaging techniques poses strong challenges, and only a few studies on motor imagery or walking observation have been performed so far. Functional near-infrared spectroscopy (fNIRS) is becoming an important research tool to assess functional activity in neurological populations or for special tasks, such as walking, because it allows investigating brain hemodynamic activity in an ecological setting, without strong immobility constraints. A systematic review following PRISMA guidelines was conducted on the fNIRS-based examination of gait disorders. Twelve of the initial yield of 489 articles have been included in this review. The lesson learnt from these studies suggest that oxy-hemoglobin levels within the prefrontal and premotor cortices are more sensitive to compensation strategies reflecting postural control and restoration of gait disorders. Although this field of study is in its relative infancy, the evidence provided encourages the translation of fNIRS in clinical practice, as it offers a unique opportunity to explore in depth the activity of the cortical motor system during real walking in neurological patients. We also discuss to what extent fNIRS may be applied for assessing the effectiveness of rehabilitation programs.
Introduction
Walking is one of the most fundamental motor functions in humans,1–3 often impaired in some focal neurological conditions (ie, stroke), or neurodegenerative diseases, such as Parkinson’s disease (PD).4 Worldwide almost two thirds of people over 70 years old suffer from gait disorders, and because of the progressively ageing population, an increasing pressure on health care systems is expected in the coming years.5
Although the physiological basis of walking is well understood, pathophysiological mechanisms in neurological patients have been poorly described. This is caused by the difficulty to assess in vivo neuronal processes during overt movements.
During the past 20 years, functional magnetic resonance imaging (fMRI) has been the preferred instrument to investigate mechanisms underlying movement control6 as well as movement disorders.7 fMRI allows measuring the blood oxygenation level-dependent (BOLD) signal that, relying on variations in deoxy-hemoglobin (deoxyHb) concentrations, provides an indirect measure of functional activity of the human brain.8 Patterns of activation/deactivation and connectivity across brain regions can be detected with a very high spatial resolution for both cortical and subcortical structures. This technique, however, is characterized by severe limitations and constraints about motion artifacts and only small movements are allowed inside the scanner. This entails dramatic compromises on the experimental design and on the inclusion/exclusion criteria. Multiple solutions have been attempted to overcome such limitations. For instance, many neuroimaging studies have been performed on the motor imagery,9,10 but imaging can be different from subject to subject,11 and imagined walking and actual walking engage different brain networks.12 Other authors have suggested the application of virtual reality,13 and there have been a few attempts to allow an almost real-walking sequence while scanning with fMRI.14,15Additional opportunities to investigate the mechanisms sustaining walking control include the use of surrogate tasks in the scanner as proxy of walking tasks,16 or to “freeze” brain activations during walking using positron emission tomography (PET) radiotracers, which allow the retrospective identification of activation patterns, albeit with some uncertainties and low spatial and temporal resolution.12
Therefore, until now there has not been an ecological way to noninvasively assess neurophysiological correlates of walking processes in gait disorders.
Functional near-infrared spectroscopy (fNIRS) is becoming an important research tool to assess functional activity in special populations (neurological and psychiatric patients)17 or for special tasks.18–21 fNIRS is a noninvasive optical imaging technique that, similarly to fMRI, measures the hemodynamic response to infer the underlying neural activity. Optical imaging is based on near-infrared (650-1000 nm) light propagation into scattering tissues and its absorption by 2 major chromophores in the brain, oxy-hemoglobin (oxyHb) and deoxyHb, which show specific absorption spectra depending on the wavelength of the photons.22 Typically, an fNIRS apparatus is composed of a light source that is coupled to the participant’s head via either light-emitting diodes (LEDs) or through fiber-optical bundles with a detector that receives the light after it has been scattered through the tissue. A variation of the optical density of the photons measured by detectors depends on the absorption of the biological tissues (Figure 1A). Using more than one wavelength and applying the modified Beer-Lambert law, it is possible to infer on the changes of oxyHb and deoxyHb concentrations.23 fNIRS has a number of definite advantages compared to fMRI, its major competitor: (a) it does not pose immobility constrains,25 (b) is portable,26 (c) allows recording during real walking,27 (d) allows long-lasting recordings, (e) it does not produce any noise, (f) it makes possible the investigation of brain activity during sleep,28 (f) it allows to obtain a richer picture of the neurovascular coupling as it measures changes in both oxyHb and deoxyHb concentration with high temporal resolution (up to milliseconds). High temporal resolution is usually not mandatory for the investigation of the hemodynamic response whose dynamic takes at least 3 to 5 seconds, but it can be useful for the study of transient hemodynamic activity like the initial dip29 or to detect subtle temporal variations in the latency of the hemodynamic response across different experimental conditions.19,21,30 The major drawback of fNIRS in comparison to fMRI is its lower spatial resolution (few centimeters under the skull) and its lack of sensitivity to subcortical regions.18,19 However, this might be considered a minor limitation, as there is a large body of evidence suggesting that (a) cortical mechanisms take place in walking,31 (b) the organization of the motor system is distributed along large brain regions,32and (c) the function of subcortical structures is mirrored in the cerebral cortex.33
Figure 1. Illustration of penetration depth of near-infrared light into the tissue in a probe configuration used to investigate motor performances during walking task (upper row). The picture shows brain reconstruction from a high-resolution anatomical MRI. The spheres placed over the skull correspond to vitamin E capsules employed during the MRI to mark the positions of the optodes and to allow the coregistration of the individual anatomy together with the optode position. In this illustration, only the photons propagation from one source (S) to one detector (D) have been simulated. The yellow-red scale indicates the degree of sensitivity74 for the considered source-detector pair to the head/brain structures. (A, B, and C) Lower row: Examples of fNIRS experimental device used for assessing brain activity during real walking tasks. These fNIRS approaches included either commercial device, such as (A) wireless portable fNIRS system (NIRx; Germany) or support systems for treadmill walking activity with body weight support24 (B) or with free movement range (C).
Continue —> Near-Infrared Spectroscopy in Gait Disorders – Feb 14, 2017