Introduction
Stroke is a severe neurological disease caused by the blockages or rupture of cerebral blood vessels, leading to significant physical disability and cognitive impairment (1, 2). The recent statistics from the World Health Organization indicate that worldwide 15 million people annually suffer from the effect of stroke, and more than 5 million stroke patients survive and, however, require a prolonged physical therapy to recover motor function. Recent trends predict increased stroke incidence at younger ages in the upcoming years (3, 4). Approximately four-fifths of all survived stroke patients suffer from the problems of hemiparesis or hemiplegia and, as a result, have difficulties in performing activities of daily living (ADL). Stroke causes tremendous mental and economic pressure on the patients and their families (5). Medical research has proved that, owing to the neural plasticity of the human brain, appropriate rehabilitation trainings are beneficial for stroke survivors to recover musculoskeletal motor abilities. Repetitive and task-oriented functional activities have substantial positive effects on improving motor coordination and avoiding muscle atrophy (6, 7). Traditional stroke rehabilitation therapy involves many medical disciplines, such as orthopedics, physical medicine, and neurophysiology (8, 9). Physiotherapists and medical personnel are required to provide for months one-on-one interactions to patients that are labor intensive, time consuming, patient-passive, and costly. Besides, the effectiveness of traditional therapeutic trainings is limited by the personal experiences and skills of therapists (10, 11).
In recent decades, robot-assisted rehabilitation therapies have attracted increasing attention because of their unique advantages and promising applications (12, 13). Compared with the traditional manual repetitive therapy, the use of robotic technologies helps improve the performance and efficiency of therapeutic training (14). Robot-assisted therapy can deliver high-intensive, long-endurance, and goal-directed rehabilitation treatments and reduce expense. Besides, the physical parameters and the training performance of patients can be monitored and evaluated via built-in sensing systems that facilitate the improvement of the rehabilitation strategy (15, 16). Many therapeutic robots have been developed to improve the motor functions of the upper extremity of disabled stroke patients exhibiting permanent sensorimotor arm impairments (17). The existing robots used for upper limb training can be basically classified into two types: end-point robots and exoskeleton robots. End-point robots work by applying external forces to the distal end of impaired limbs, and some examples are MIME (18), HipBot (19), GENTLE/s (20), and TA-WREX (21). Comparatively, exoskeleton robots have complex structures similar to anatomy of the human skeleton; some examples of such robots are NMES (22), HES (23), NEUROExos (24), CAREX-7 (25), IntelliArm (26), BONES (27), and RUPERT (28). The joints of the exoskeleton need to be aligned with the human anatomical joints for effective transfer of interactive forces.
The control strategies applied in therapeutic robots are important to ensure the effectiveness of rehabilitation training. So far, according to the training requirement of patients with different impairment severities, many control schemes have been developed to perform therapy and accelerate recovery. Early rehabilitation robot systems implemented patient-passive control algorithms to imitate the manual therapeutic actions of therapists. These training schemes are suitable for patients with severe paralysis to passively execute repetitive reaching tasks along predefined trajectories. Primary clinical results indicate that patient-passive training contributes to motivating muscle contraction and preventing deterioration of arm functions. The control of the human–robot interaction system is a great challenge due to its highly nonlinear characteristics. Many control algorithms have been proposed to enhance the tracking accuracy of passive training, such as the robust adaptive neural controller (29), fuzzy adaptive backstepping controller (30), neural proportional–integral–derivative (PID) controller (31), fuzzy sliding mode controller (32), and neuron PI controller (33).
The major disadvantage of patient-passive training is that the active participation of patients is neglected during therapeutic treatment (34). Several studies suggest that, for the patients who have regained parts of motor functions, the rehabilitation treatment integrated with the voluntary efforts of patients facilitates the recovery of lost motor ability (35). The patient-active control, normally referred as patient-cooperative control and assist-as-needed control, is capable of regulating the human–robot interaction depending on the motion intention and the disability level of patients. Keller et al. proposed an exoskeleton for pediatric arm rehabilitation. A multimodal patient-cooperative control strategy was developed to assist upper limb movements with an audiovisual game-like interface (36). Duschauwicke et al. proposed an impedance-based control approach for patient-cooperative robot-aided gait rehabilitation. The affected limb was constrained with a virtual tunnel around the desired spatial path (37). Ye et al. proposed an adaptive electromyography (EMG) signals-based control strategy for an exoskeleton to provide efficient motion guidance and training assistance (38). Oldewurtel et al. developed a hybrid admittance–impedance controller to maximize the contribution of patients during rehabilitation training (39). Banala et al. developed a force-field assist-as-need controller for intensive gait rehabilitation training (40). However, there are two limitations in the existing patient-cooperative control strategies. Firstly, the rehabilitation training process is not completely patient-active, as the patient needs to perform training tasks along a certain predefined trajectory. Secondly, existing control strategies are executed in self-designed virtual scenarios that are generally too simple, rough, and uninteresting. Besides, applying a certain control strategy to different virtual reality scenarios is difficult.
Taking the above issues into consideration, the main contribution of this paper is to develop a control strategy for an upper limb exoskeleton to assist disabled patients in performing active rehabilitation training in a virtual scenario based on their own active motion intentions. Firstly, the overall structure design and the real-time control system of the exoskeleton system are briefly introduced. A dynamic model of the human–robot interaction system is then established using the Lagrangian approach. After that, an admittance-based patient-active controller combined with an audiovisual therapy interface is proposed to induce the active participation of patients during training. Existing commercial virtual games without a specific predetermined training trajectory can be integrated into the controller via a virtual keyboard unit. Finally, three types of experiments, namely the position tracking experiment without interaction force, the free arm movement experiment, and the virtual airplane-game operation experiment, are conducted with healthy and disabled subjects. The experimental results demonstrate the feasibility of the proposed exoskeleton and control strategy.
Exoskeleton Robot Design
The architecture of the proposed exoskeleton is shown in Figure 1. This wearable force-feedback exoskeleton robot has seven actuated degrees of freedom (DOFs) and two passive DOFs covering the natural range of movement (ROM) of humans in ADL. The robot has been designed with an open-chain structure to mimic the anatomy of the human right arm and provide controllable assistance torque to each robot joint. There are three actuated DOFs at the shoulder for internal/external rotation, abduction/adduction, and flexion/extension; two DOFs at the elbow for flexion/extension and pronation/supination; and two DOFs at the wrist for flexion/extension and ulnal/radial deviation. Besides, since the center of rotation of the glenohumeral joint varies with the shoulder girdle movement, the robot is mounted on a self-aligning platform with two passive translational DOFs to compensate the human–robot misalignment and to guarantee interaction comfort. […]
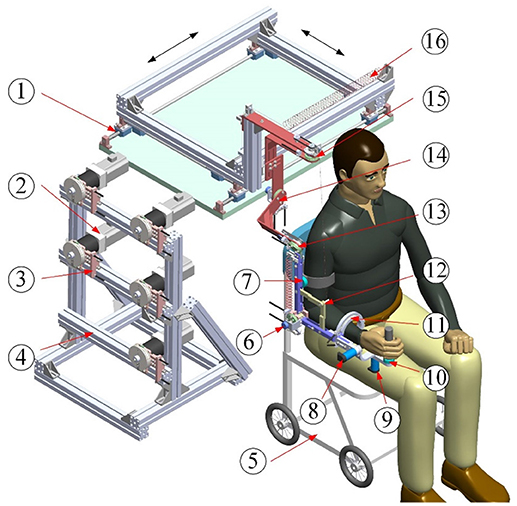
Figure 1. Architecture of upper limb rehabilitation exoskeleton (1-Self-aligning platform; 2-AC servo motor; 3-Bowden cable components; 4-Support frame; 5-Wheelchair; 6-Elbow flexion/extension; 7-Proximal force/torque sensor; 8-Wrist flexion/extension; 9-Wrist ulnal/radial deviation; 10-Distal force/torque sensor; 11-Forearm pronation/supination; 12-Auxiliary links; 13-Shoulder flexion/extension; 14-Shoulder abduction/adduction; 15-Shoulder internal/external; 16-Free-length spring).